AI in Risk Scoring – The Next-Gen Risk Management Paves a Path for Businesses
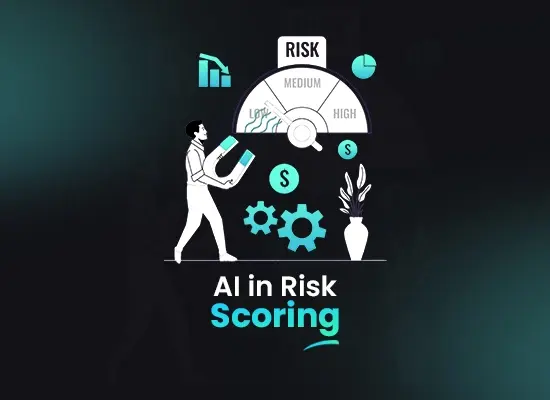
AI in risk scoring is revolutionising risk management for businesses. By analysing vast amounts of data, AI can identify subtle patterns and predict potential issues with greater accuracy than traditional methods. This allows businesses to make informed decisions, streamline operations, and mitigate risks proactively, paving the way for a more secure and profitable future.
What is Risk Scoring?
In today’s complex landscape, risk assessment software empowered by risk management with AI plays a crucial role in various industries. This software utilises AI in risk and compliance to generate risk scores, numerical values that represent the likelihood and potential impact of an undesirable event.
Risk analysis is a powerful tool that goes beyond traditional methods of risk assessment. It leverages the power of Artificial Intelligence (AI) to analyse vast amounts of data, identifying patterns and relationships that might be missed by humans. This allows for a more comprehensive and data-driven approach to risk evaluation, leading to improved decision-making.
By incorporating AI in risk assessment, risk scoring software can consider various factors, including historical data, current trends, and external influences. This comprehensive analysis allows for a more nuanced understanding of potential risks, enabling organisations to prioritise effectively and allocate resources strategically.
Furthermore, risk analysis is not static. As data engineering services evolve and new information becomes available, AI in risk and compliance enables the software to continuously refine and update the scores, ensuring that decision-making remains informed by the most current insights. This dynamic approach helps organisations adapt and respond to changes in the risk landscape proactively.
In conclusion, risk analysis powered by risk management with AI offers a sophisticated and data-driven approach to risk assessment. By leveraging the power of AI, organisations can gain deeper insights into potential risks, make informed decisions, and ultimately achieve their goals in a more secure and efficient manner.
Seamless Collaboration | Cost-Efficient Solutions | Faster Time-to-Market
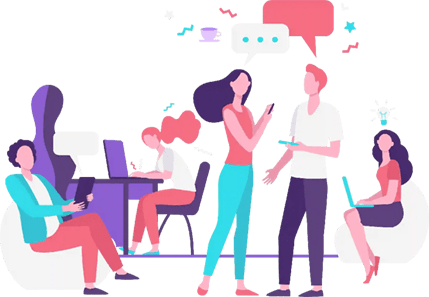
What is AI in Risk Scoring?
The realm of risk management is undergoing a significant transformation with the integration of artificial intelligence (AI). This powerful technology is finding diverse applications within risk management with AI, particularly in the areas of AI in risk analysis and AI in risk and compliance.
Risk analysis software traditionally relied on pre-defined rules and statistical models. However, these methods often lacked the ability to analyse complex data sets and identify subtle patterns. This is where AI steps in, offering a more sophisticated approach to AI in risk assessment.
AI algorithms can process massive amounts of data, including historical records, transactional behaviour, and external information sources. This enables them to identify subtle patterns and correlations that might escape traditional methods. By analysing these intricate details, AI can generate risk scores that are more accurate and predictive compared to conventional techniques.
This shift towards AI in risk analysis holds immense potential for various industries. Financial institutions can use it to assess creditworthiness and fraud risk more effectively. Insurance companies can leverage it to tailor insurance premiums based on individual risk profiles. Additionally, businesses across all sectors can benefit from AI in risk and compliance by proactively identifying and mitigating potential risks, allowing for more informed decision-making.
However, it’s important to remember that risk management with AI, like any powerful tool, requires responsible application and ethical considerations. It’s crucial to ensure that AI models are developed and deployed with fairness, transparency, and accountability at the forefront. By harnessing the potential of AI while addressing its limitations, we can create a future where risk assessment software empowers organisations to navigate an increasingly complex and dynamic world.
Importance of AI in Risk Scoring:
The world is becoming increasingly complex, and organisations face a growing number of risks across various domains. This is where risk management with AI comes in, offering a powerful tool for navigating these ever-evolving challenges. AI, or artificial intelligence, has revolutionised the way we approach risk assessment, bringing about significant advancements in AI in risk and compliance.
One of the core applications of AI in this field is risk assessment with AI. AI algorithms are capable of analysing massive amounts of data, identifying hidden patterns and trends that traditional methods might miss. This allows for the creation of more sophisticated risk assessment models, leading to more accurate and nuanced risk scores. These scores, in turn, inform better decision-making across the organisation, whether it’s related to creditworthiness, fraud detection, or operational efficiency.
Risk analysis software powered by AI offers several advantages over traditional methods. Firstly, it automates a significant portion of the risk assessment process, freeing up valuable human resources for other tasks. Secondly, AI models can learn and adapt over time, constantly improving their accuracy and effectiveness as they are exposed to new data analytics. This continuous learning capability makes AI in risk and compliance a powerful tool for staying ahead of emerging threats and vulnerabilities.
Overall, the importance of AI in risk analysis cannot be overstated. As organisations face an increasingly complex risk landscape, AI offers a powerful and versatile tool for proactive risk management. By leveraging the capabilities of risk assessment with AI, organisations can make more informed decisions, optimise resource allocation, and ultimately achieve greater resilience in the face of uncertainty.
Traditional Approaches to Risk Scoring:
For a long time, risk management with AI primarily relied on traditional approaches for risk analysis. These methods involved building static models based on historical data and pre-defined rules. While these approaches played a role in early AI in risk and compliance efforts, they presented several limitations.
One major limitation of traditional approaches is their reliance on historical data. This data might not always capture the nuances of risk assessment in a constantly evolving environment. Additionally, these models often require significant manual effort to maintain and update, making them inflexible and time-consuming.
Another limitation is the lack of adaptability. Traditional models struggle to learn and adapt to new information and changing patterns, leading to potentially inaccurate risk scores. This can hinder the effectiveness of risk analysis software and decision-making processes.
Despite these limitations, traditional approaches still hold some value. They can provide a baseline for risk management with AI and machine learning development by offering a foundation for building more sophisticated models. However, as the field of AI in risk and compliance continues to evolve, the limitations of traditional approaches become increasingly apparent. This paves the way for the exploration of more advanced techniques, such as machine learning and deep learning, to enhance risk assessment capabilities.
Machine Learning Algorithms for Risk Scoring:
The landscape of risk management is undergoing a significant transformation with the AI integration. AI empowers organisations to leverage machine learning (ML) algorithms for risk scoring, significantly enhancing their ability to assess, manage, and mitigate potential threats.
At the heart of this transformation lies risk assessment with AI. Machine learning algorithms, trained on vast datasets, analyse historical data, identify patterns, and predict the likelihood of future events. This powerful capability allows organisations to proactively identify and prioritise risks, moving beyond reactive approaches to proactive risk mitigation.
AI in risk and compliance plays a crucial role in ensuring adherence to regulations and ethical practices. Machine learning operations (MLOps) can be utilised to assess compliance risk, identify potential regulatory violations, and automate compliance processes. This not only reduces the risk of non-compliance but also streamlines internal processes, leading to significant efficiency gains.
Risk analysis software powered by machine learning algorithms offers numerous benefits. These software solutions can automate various aspects of the risk assessment process, freeing up human resources for more strategic tasks. Additionally, they provide real-time insights and continuous monitoring, enabling organisations to adapt their risk management strategies in a dynamic environment.
By implementing risk management with AI, organisations can gain a competitive edge. Machine learning algorithms offer a data-driven approach to risk assessment, enabling informed decision-making, improved resource allocation, and ultimately increased resilience in the face of evolving risks.
Existing Open-Source Models for AI in Risk Scoring:
The field of risk management is undergoing a significant transformation with the integration of AI. Open-source models are playing a crucial role in democratising access to this powerful technology, allowing organisations of all sizes to leverage risk assessment with AI, AI in risk and compliance, and risk management with AI strategies.
These open-source models offer a cost-effective and flexible alternative to traditional, proprietary solutions. Developers and organisations can readily access, adapt, and deploy these models for various risk assessment tasks, fostering innovation and collaboration in the risk analysis software landscape.
Several open-source models demonstrate the potential of risk management with AI. TensorFlow Risk (TFR) provides a framework for building and deploying risk assessment models, while scikit-learn offers various machine learning algorithms applicable to risk analysis and classification. Additionally, projects like PMML (Predictive Model Markup Language) facilitate the exchange and integration of risk assessment models across different platforms and tools.
The adoption of open-source AI in risk management presents both opportunities and challenges. While it empowers organisations with greater control and transparency, careful consideration of potential biases, data quality, and explainability remains vital. As the field evolves, open-source AI holds immense promise for shaping the future of risk analysis software and empowering organisations to navigate an increasingly complex risk landscape.
Challenges and Limitations of AI in Risk Scoring:
AI in risk scoring, particularly in areas like risk assessment and compliance, offers immense potential. However, it’s crucial to acknowledge the challenges and limitations of using AI-powered risk analysis systems.
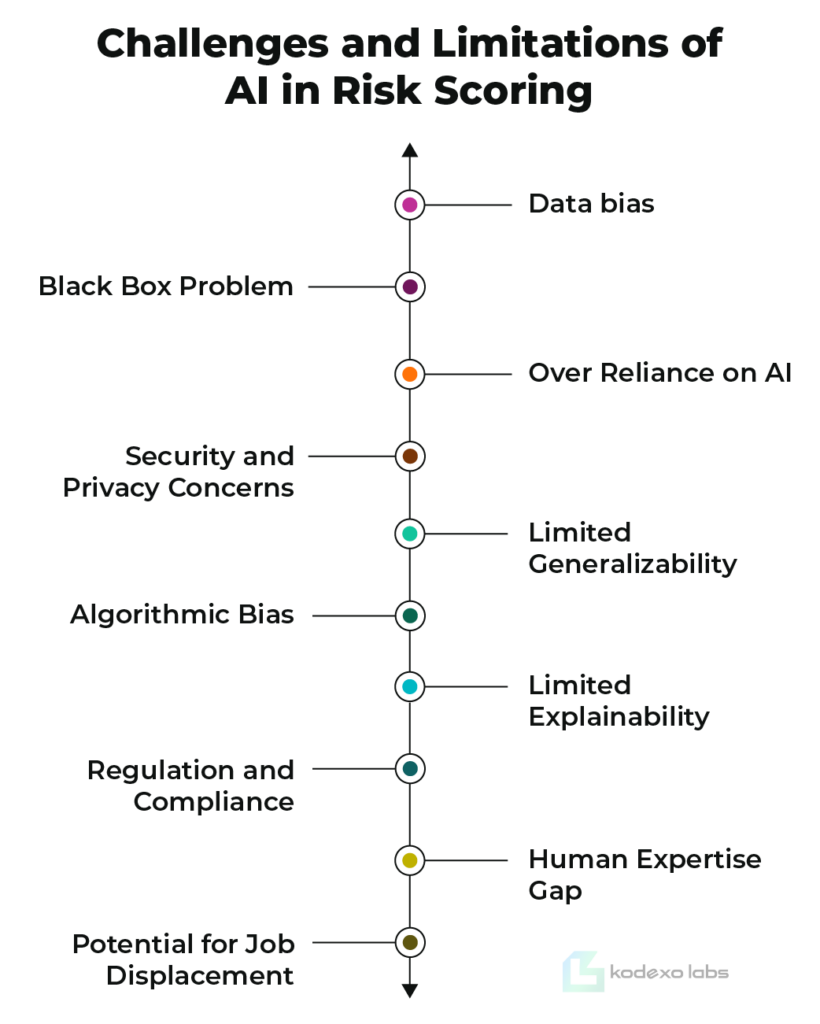
Data Bias:
AI models are only as good as the data they’re trained on. Biases present in historical data used for training can be perpetuated and amplified by AI, leading to unfair and inaccurate risk assessments. Mitigating data bias requires careful data selection, cleaning, and ongoing monitoring.
Black Box Problem:
Many AI models lack transparency, making it difficult to understand how they arrive at specific risk scores. This “black box” effect hinders explainability and can raise concerns about fairness and accountability, especially in areas like loan approvals or criminal justice.
Over Reliance on AI:
While AI can be a valuable tool, relying solely on AI-generated risk scores for critical decisions can be risky. Human expertise and judgment remain essential for interpreting and contextualizing AI outputs, ensuring responsible decision-making in risk management and compliance.
Security and Privacy Concerns:
AI systems can be vulnerable to cyberattacks and data breaches, potentially exposing sensitive information or compromising the integrity of risk assessments. Robust security measures and data privacy protocols are crucial when using Risk and compliance with AI processes.
Limited Generalizability:
AI models often perform well on specific datasets they’re trained on. However, applying them to new or different contexts can lead to inaccurate or unreliable results. Careful consideration of generalizability and potential limitations is crucial when employing AI risk assessment software.
Algorithmic Bias:
Even without explicit bias in the training data, the algorithms themselves can introduce bias if they’re not designed and implemented carefully. Developers and users must be aware of potential algorithmic bias and take steps to mitigate it through proper design, testing, and ongoing monitoring.
Limited Explainability:
Difficulties in explaining how AI models arrive at specific risk scores can hinder user trust and acceptance. Efforts to improve model explainability and provide transparent insights into the decision-making process are crucial for building trust in AI-based risk assessment.
Regulation and Compliance:
The use of AI in risk management and compliance is evolving rapidly, and regulations are still catching up. Organizations need to stay up-to-date on evolving regulations and ensure their AI practices adhere to legal and ethical frameworks.
Human Expertise Gap:
While AI can automate certain aspects of risk assessment, it can’t replace human expertise entirely. Organizations implementing AI risk analysis systems must invest in training and development to ensure their workforce possesses the necessary skills to utilize and oversee AI responsibly.
Potential for Job Displacement:
Concerns exist regarding AI potentially displacing human jobs in areas like credit scoring or fraud detection. Responsible implementation of AI requires careful planning and consideration of potential workforce impacts, including retraining and upskilling initiatives.
By recognizing these challenges and limitations, organizations can approach AI for risk analysis with a critical and responsible perspective, harnessing its benefits while mitigating its potential risks. Remember, Risk and compliance with AI is a powerful tool, but it should be used thoughtfully and responsibly to ensure fair, accurate, and ethical risk management practices.
Explainability and Interpretability for AI in Risk Scoring Models:
Understanding how AI makes decisions within risk scoring models is vital for the use of AI in risk management. Explainability and interpretability are essential for building trust within Risk and compliance with AI. These concepts allow us to determine why a model arrived at a specific outcome, highlighting potential biases or flaws present in the AI in risk assessment. Explainability is particularly important for regulatory mandates, ensuring the reasoning behind any AI used in risk assessment software can be clearly articulated.
Risk and compliance with AI demands transparency. Understanding the inner workings of AI in risk assessment enables organizations to justify their risk-related decisions confidently. This promotes ethical practices, fosters trust with stakeholders, and enhances overall risk management strategies. By prioritizing explainability and interpretability principles, businesses relying on risk assessment software can make better-informed, risk-aware choices powered by AI.
Explainable and interpretable AI in risk management offers a significant advantage: understanding risk factors. Analysing how AI in risk assessment uses various features – historical data, behavioural patterns, etc. – provides a deeper grasp of the factors truly driving risk. This insight allows for more targeted interventions by the Risk and compliance with AI teams, ensuring resources are directed towards areas of highest concern. Risk assessment software powered by explainable AI streamlines operations and enables more efficient, data-driven risk mitigation plans.
As reliance on AI in risk management and Risk and compliance with AI grows, explainability and interpretability become non-negotiable. Regulators and stakeholders alike seek transparency and accountability when AI is involved in risk assessment. Embracing these principles not only ensures compliance but also reinforces confidence in the reliability and fairness of risk assessment software.
Model Evaluation and Validation Techniques:
The deployment of AI in risk management requires careful attention to model evaluation and validation techniques. Risk and compliance with AI solutions often involve the use of predictive risk analysis models, where accuracy and reliability are paramount. One crucial aspect is ensuring these AI models remain free from bias that could lead to discriminatory or harmful outcomes. Continuous monitoring of AI in risk assessment models can help maintain their effectiveness and identify areas where potential issues like biases or model drift might occur.
A critical component of AI in risk management involves thorough model evaluation. This can include the use of standard metrics such as accuracy, precision, recall, and F1-score to measure the ability of Risk assessment with AI models to correctly predict outcomes. In the context of risk scoring, evaluating the model’s ability to discriminate between high-risk and low-risk cases is vital. Robust validation processes should be in place to confirm that Risk and compliance with AI solutions remain accurate and reliable over time.
In AI in risk assessment, model validation often involves using holdout datasets that were not used during the model’s training phase. This helps ensure that the model is generalizable to new data and not simply overfitting to the training set. AI in risk management solutions, including risk assessment software, necessitate continuous model monitoring to detect potential model drift, concept drift, or data shifts. This ensures the model maintains its integrity over time, even as underlying conditions and data distributions may change
Risk and compliance with AI systems heavily rely on sophisticated scoring models. Validation involves stress testing these models under various adversarial conditions or simulated scenarios. This allows practitioners to assess the robustness and resilience of the AI in risk assessment and management solutions. Using back testing techniques, risk managers can evaluate the model’s performance on historical data, providing valuable insights into potential shortcomings that need to be addressed.
Seamless Collaboration | Cost-Efficient Solutions | Faster Time-to-Market
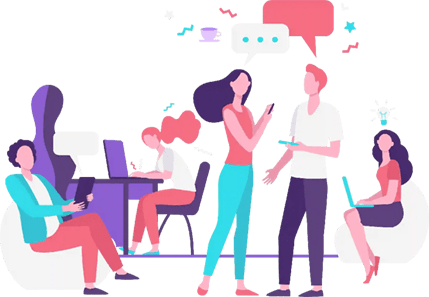
Future Trends and Innovations in AI in Risk Scoring:
AI in risk management is rapidly evolving, leading to exciting innovations in AI in risk assessment and Risk and compliance with AI. These advancements are poised to significantly alter the landscape of risk scoring and offer businesses a powerful advantage.
One key trend is the increasing sophistication of AI-powered risk assessment software. These tools leverage advanced machine learning algorithms to analyse vast amounts of data, uncovering hidden patterns and identifying previously unseen risks. This allows for more accurate and granular risk assessments, enabling organizations to make informed decisions concerning loan approvals, insurance premiums, and potential fraud.
Risk and compliance with AI are another area witnessing rapid growth. AI models can automate compliance tasks, analyse regulations for potential conflicts, and even predict potential compliance breaches. This not only reduces manual workload but also strengthens risk management practices by proactively identifying and mitigating compliance risks
Looking ahead, we can expect further integration of AI in risk management solutions. Cloud-based platforms, for example, will offer real-time risk insights and enable organizations to continuously adapt their risk assessment strategies in a dynamic environment. Additionally, the rise of explainable AI will be crucial in building trust and transparency in AI-powered risk assessment software. By understanding the rationale behind AI decisions, organizations can ensure responsible and ethical implementation of these powerful tools.
Conclusion:
While AI in risk scoring holds immense potential, its implementation requires careful consideration. While it offers increased accuracy, efficiency, and personalization, concerns like bias, transparency, and responsible use remain crucial. Mitigating potential harm and developing ethical frameworks are key to unlocking AI’s positive impact in risk assessment.
AI in risk scoring offers potential for enhanced accuracy and efficiency, but ethical concerns and responsible development require ongoing attention. In conclusion, AI in risk scoring is bright. With continuous advancements in AI in risk assessment and Risk and compliance with AI, organizations can expect to gain a deeper understanding of risks, make better decisions, and navigate uncertainty with greater confidence.
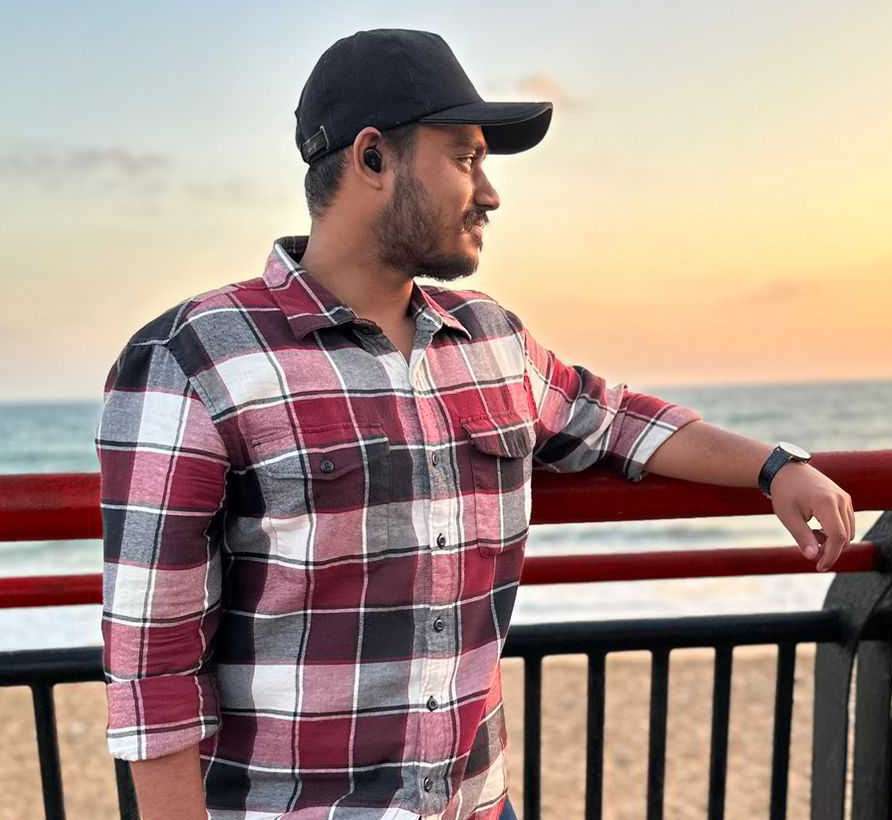
Author Bio
Syed Ali Hasan Shah, a content writer at Kodexo Labs with knowledge of data science, cloud computing, AI, machine learning, and cyber security. In an effort to increase awareness of AI’s potential, his engrossing and educational content clarifies technical challenges for a variety of audiences, especially business owners.