AI in Treatment Systems – Healthcare’s New Face Unlocked with AI’s Capabilities
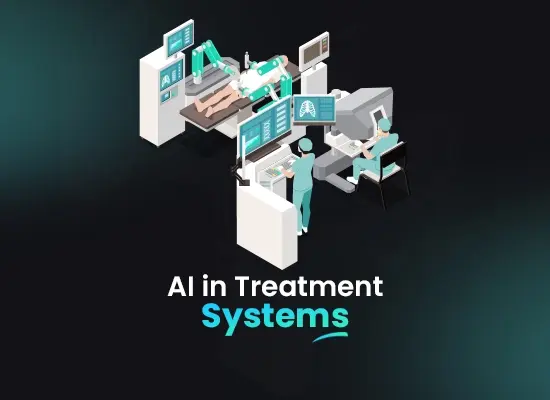
The world of treatment systems, across various fields, is undergoing a significant transformation due to the integration of Artificial Intelligence (AI). From optimising industrial processes to personalising healthcare approaches, AI’s ability to analyse vast amounts of data and make intelligent decisions is revolutionising how treatment is delivered and managed. This influx of AI is leading to enhanced efficiency, improved accuracy, and the potential for personalised treatment plans, making significant strides towards more effective and successful treatment systems.
What is AI in Treatment?
AI in treatment is rapidly transforming the way healthcare professionals approach patient care. By harnessing the power of Machine Learning (ML) and Artificial Intelligence (AI), these tools are augmenting traditional methods to deliver more precise and personalised treatment plans.
One key area of impact is AI in recommendation systems. These systems analyse vast amounts of medical data, including patient records, clinical trials, and medical research. By identifying patterns and correlations, AI can recommend the most effective treatment options based on an individual’s specific medical history, diagnosis, and other relevant factors. This not only personalised care but also allows doctors to leverage the collective knowledge base of medicine, potentially leading to improved outcomes.
Another exciting application is automated treatment planning. AI algorithms can analyse medical scans and images, taking into account factors like tumour size and location. Based on this analysis, AI can suggest potential treatment options, such as radiation therapy dosage or surgical approaches. However, it’s important to remember that AI in treatment is meant to be a supportive tool, not a replacement for human expertise. Doctors use their knowledge, experience, and judgement alongside AI recommendations to create the best possible treatment plan for each patient.
The impact of AI in treatment extends beyond individual patients. Healthcare systems as a whole benefit from the efficiency and accuracy AI offers. By automating tasks like data analysis and treatment planning, AI frees up valuable time for doctors to focus on patient interaction and complex decision-making. Additionally, AI development can help healthcare systems optimise resource allocation and identify areas for improvement, ultimately leading to a more efficient and cost-effective healthcare system.
In conclusion, AI in treatment represents a significant leap forward in personalised medicine. Through AI treatment, automated treatment planning, and its overall impact on healthcare systems, AI is poised to revolutionise the way we approach patient care, leading to a future of more effective and efficient medical treatment.
Seamless Collaboration | Cost-Efficient Solutions | Faster Time-to-Market
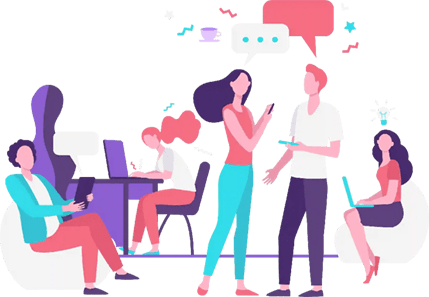
What is the role of AI in Recommendation Systems in Healthcare?
The role of AI in recommendation systems within healthcare is rapidly transforming the way medical professionals approach patient care. These AI-powered treatment recommendation analyse vast amounts of medical data, including patient history, test results, and treatment outcomes, to suggest personalised strategies for diagnosis, treatment, and preventive care. At the core of this transformation lies AI and Machine Learning development ability to analyse complex medical information and identify patterns that might be missed by the human eye. This allows healthcare systems to leverage AI in treatment for various purposes, including:
Automated Treatment Planning:
By analysing data on past successful treatments for similar patient profiles, AI in treatment can suggest potential treatment plans tailored to the individual’s specific needs. This can significantly improve the efficiency and accuracy of treatment planning, allowing doctors to focus on the nuances of each case.
Improved Diagnosis:
AI in treatment can assist healthcare professionals by analysing patient symptoms and medical history to suggest potential diagnoses. This can be particularly helpful in identifying rare or complex conditions, ultimately leading to faster and more accurate diagnoses.
Personalised Care:
Healthcare is no longer a one-size-fits-all approach. AI in treatment recommendation allows providers to personalise treatment plans by factoring in a patient’s unique medical history, lifestyle habits, and genetic makeup. This personalised approach can lead to better treatment outcomes and improved patient satisfaction.
The impact of AI in treatment recommendation extends beyond individual patients. By analysing healthcare data on a broader scale, these systems can identify trends and patterns that inform public health initiatives and resource allocation within healthcare systems. Overall, AI in treatment recommendation is playing a crucial role in revolutionising healthcare. By leveraging the power of AI to analyse vast amounts of data and suggest personalised recommendations, healthcare professionals can deliver more effective and efficient care for their patients.
7 Ways How Deep Learning (DL) Helps Healthcare Systems:
Deep Learning (DL), a powerful subfield of artificial intelligence (AI), is rapidly transforming healthcare systems by enabling groundbreaking advancements in diagnosis, treatment, and patient care. Here are seven key ways deep learning is making a positive impact:
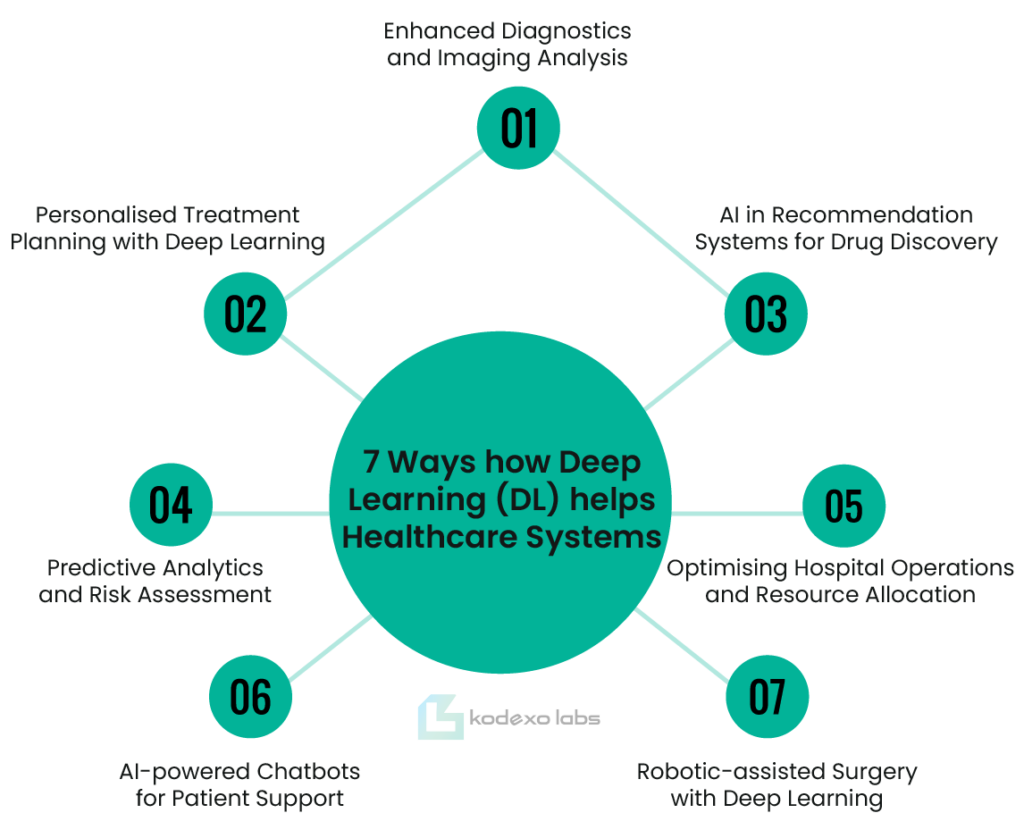
1- Enhanced Diagnostics and Imaging Analysis:
Deep learning algorithms can analyse medical images like X-rays, MRIs, and CT scans with exceptional accuracy. This allows for earlier detection of diseases, improved cancer diagnoses, and more precise identification of abnormalities. By sifting through vast amounts of medical data, deep learning can even uncover subtle patterns unseen by the human eye, leading to a new era of AI-powered diagnostics.
2- Personalised Treatment Planning with Deep Learning:
Deep learning is revolutionising treatment planning by providing healthcare professionals with valuable insights into individual patient data. AI algorithms can analyse a patient’s medical history, genetic makeup, and real-time health information to recommend personalised treatment options. This “automated treatment planning” empowers doctors to tailor therapies to each patient’s unique needs, potentially leading to more effective and successful outcomes.
3- AI in Recommendation Systems for Drug Discovery:
Deep learning is accelerating drug discovery by analysing vast datasets of molecular structures and patient responses. AI in recommendation systems can identify promising drug candidates and predict their potential effectiveness, streamlining the development process. This not only reduces the time it takes to bring new drugs to market but also allows for a more targeted approach to treatment, paving the way for personalised medicine.
4- Predictive Analytics and Risk Assessment:
Deep learning excels at identifying patterns in complex data sets. Healthcare systems can leverage this capability for predictive analytics, allowing them to assess a patient’s risk of developing certain diseases based on their medical history and lifestyle factors. This early risk identification empowers doctors to implement preventative measures and personalise care plans, potentially improving patient outcomes and reducing overall healthcare costs.
5- Optimising Hospital Operations and Resource Allocation:
Deep learning can use data analytics vast amounts of healthcare data to identify inefficiencies and optimise hospital operations. By predicting patient flow, resource needs, and potential bottlenecks, AI can help healthcare systems allocate resources more effectively, leading to faster wait times, improved patient care, and reduced administrative burdens.
6- AI-powered Chatbots for Patient Support:
Deep learning is creating intelligent chatbots that can answer patient questions, provide basic medical information, and even offer emotional support. AI chatbot development can alleviate pressure on healthcare providers by handling routine inquiries and offering 24/7 patient support, ultimately improving the overall patient experience.
7- Robotic-assisted Surgery with Deep Learning:
Deep learning algorithms are enhancing robotic-assisted surgery by providing real-time guidance and improving surgical precision. AI can analyse medical images during surgery, identify anatomical structures, and even predict potential complications. This collaboration between human expertise and Machine Learning development is leading to minimally invasive surgeries, faster recovery times, and improved patient outcomes.
In conclusion, deep learning is playing a pivotal role in transforming healthcare systems by offering a new level of diagnostic accuracy, personalised treatment planning, and operational efficiency. As AI in treatment continues to evolve, we can expect even more groundbreaking advancements that will revolutionise how we approach healthcare in the future.
How can Business Owners Benefit from AI in Treatment Systems?
The healthcare industry is witnessing a transformative shift with the integration of Artificial Intelligence (AI) in treatment systems. Business owners in this domain can leverage AI to unlock a multitude of benefits, propelling their organisations towards a future of efficiency, accuracy, and improved patient outcomes.
One of the most impactful ways AI is revolutionising treatment systems is through AI in treatment recommendation. Imagine a scenario where a doctor is presented with a complex case. AI, having analysed vast amounts of medical data and treatment histories through data engineering services, can act as a powerful support system. It can recommend personalised treatment plans, factoring in the patient’s unique medical profile, response to past treatments, and potential drug interactions. This not only streamlines the decision-making process for doctors but also ensures a more data-driven approach, potentially leading to better patient care.
Furthermore, AI paves the way for automated treatment planning. Repetitive tasks like analysing medical scans and historical data can be automated using AI algorithms. This frees up valuable time for doctors, allowing them to focus on more intricate aspects of patient care. Additionally, AI-powered automated treatment planning can identify patterns and potential risks that may be missed by human analysis, leading to a more comprehensive and effective treatment approach.
The benefits of AI in treatment systems extend beyond individual patients. By analysing vast datasets of patient information, AI can identify trends and patterns within healthcare systems. This allows business owners to make informed decisions about resource allocation, optimise staffing levels, and predict potential outbreaks or disease patterns. Essentially, AI in treatment recommendation can be used to proactively address healthcare needs, leading to a more efficient and responsive healthcare infrastructure.
However, it’s important to remember that AI is a tool, and its effectiveness hinges on responsible implementation. Business owners must ensure proper data security measures are in place to protect patient privacy. Additionally, human expertise remains irreplaceable. AI should be viewed as a support system for doctors, not a replacement. By embracing AI responsibly and ethically, business owners can unlock its true potential and transform their healthcare organisations into bastions of cutting-edge treatment and improved patient care.
5 Benefits of Neural Network (NN) in Automated Treatment Planning?
The integration of Artificial Intelligence (AI) in treatment planning has the potential to revolutionise healthcare systems. Neural networks (NNs), a powerful subset of AI, are making significant strides in automating treatment planning, leading to several key benefits:
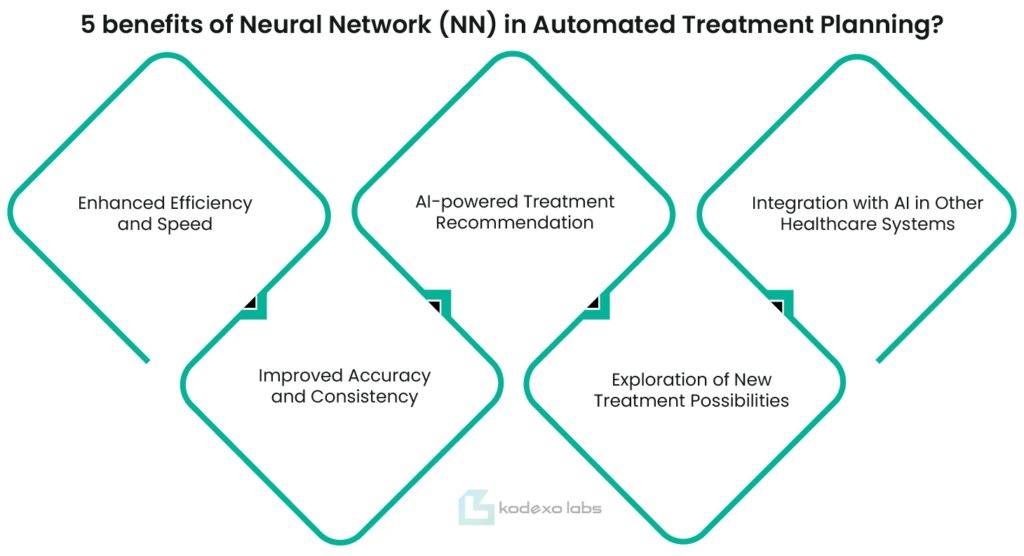
1- Enhanced Efficiency and Speed:
Traditional treatment planning is a time-consuming process requiring extensive expertise. Neural networks can analyse vast amounts of medical data, including patient scans, treatment history, and response rates. This allows them to generate personalised treatment plans much faster, improving workflow efficiency for oncologists.
2- Improved Accuracy and Consistency:
Human experts, while highly skilled, can introduce inconsistencies into treatment plans due to factors like fatigue or experience level. Neural networks, trained on vast datasets of successful treatment outcomes, can generate more standardised and potentially more accurate treatment plans, reducing the risk of human error.
3- AI-powered Treatment Recommendation:
Neural networks can act as powerful treatment recommendations within the context of automated treatment planning. By analysing patient data and treatment response rates, they can suggest optimal treatment options, radiation dosages, and beam configurations to the oncologist. This empowers physicians to make more informed decisions while personalising care for each patient.
4- Exploration of New Treatment Possibilities:
The vast computational power of neural networks allows them to explore a wider range of treatment possibilities compared to traditional methods. This can lead to the identification of more effective treatment strategies and potentially improve patient outcomes.
5- Integration with AI in Other Healthcare Systems:
The benefits of neural networks extend beyond automated treatment planning. As AI continues to play a larger role in healthcare systems, neural networks in treatment planning can seamlessly integrate with other AI-powered systems, such as those for diagnostics or drug discovery. This holistic approach to patient care, facilitated by AI, has the potential to significantly improve health outcomes.
Overall, the use of neural networks in automated treatment planning offers a promising avenue for increased efficiency, accuracy, and personalisation in cancer care. By leveraging AI’s capabilities, healthcare professionals can focus on delivering the best possible care to their patients while optimising treatment strategies.
What are the Applications of Machine Learning Operations (MLOps) in Treatment Systems?
Machine Learning Operations (MLOps) plays a crucial role in transforming how healthcare leverages machine learning (ML) for treatment systems. Here’s how MLOps empowers the development and deployment of effective ML models in this domain:
Enhanced Efficiency and Scalability:
MLOps streamlines the entire ML lifecycle, from data preparation and model training to deployment and monitoring. This automation allows healthcare institutions to develop and integrate ML models into treatment systems faster, enabling them to address evolving patient needs and adapt to new medical discoveries.
Improved Model Performance and Reliability:
MLOps practices like version control and continuous integration/continuous delivery (CI/CD) ensure consistent and reliable model performance. By automating testing and monitoring, MLOps helps identify and address issues with models before they impact patient care.
Regulatory Compliance and Explainability:
The healthcare industry adheres to strict regulations regarding patient data privacy and model interpretability. MLOps tools can facilitate data governance and ensure models meet regulatory requirements. Additionally, MLOps can help document the reasoning behind a model’s decisions, fostering trust and transparency in treatment recommendations.
Personalised Medicine and Early Intervention:
MLOps empowers the creation of ML models that analyse vast amounts of patient data to personalise treatment plans. Early detection of potential health issues becomes possible through real-time monitoring and analysis supported by MLOps. This allows for preventative measures and targeted interventions.
In conclusion, MLOps acts as a bridge between the potential of ML and its practical application within treatment systems. By streamlining processes, ensuring reliability, and facilitating regulatory compliance, MLOps paves the way for more effective and personalised healthcare.
What is the Role of Generative AI in Recommendation Systems for Healthcare?
Generative AI, a branch of artificial intelligence adept at creating new data, is transforming the landscape of treatment recommendation in healthcare. These systems analyse vast amounts of patient data to suggest personalised treatment plans, clinical trials, or preventative measures. Here’s how generative AI plays a crucial role in this domain:
Expanding the Data Landscape:
Generative AI can address the challenge of limited or incomplete patient data. By creating synthetic data that replicates real-world scenarios, it allows healthcare providers to train recommendation systems on a broader range of information. This leads to more comprehensive insights and reduces bias caused by data scarcity.
Personalised Treatment Recommendations:
Traditional treatment recommendations often rely on historical data, which may not always account for individual patient variations. Generative AI development can create patient-specific profiles that consider factors like genetics, lifestyle, and medical history. This enables healthcare providers to recommend treatments with a higher chance of success for each patient.
Drug Discovery and Clinical Trial Matching:
Generative AI integration can accelerate drug discovery by simulating potential molecules and their interactions with diseases. This helps researchers identify promising drug candidates more efficiently. Additionally, generative AI can analyse patient data to suggest clinical trials that best match their specific needs and conditions.
Proactive Preventative Care:
By analysing trends and patterns in patient data, generative AI can predict potential health risks. This allows healthcare providers to recommend preventative measures and early interventions, potentially leading to better health outcomes and reduced healthcare costs.
It’s important to note that generative AI in healthcare is still evolving. Ensuring data privacy and addressing potential biases in the generated data are crucial considerations. However, the potential of generative AI to personalise recommendations and improve healthcare delivery is undeniable.
Seamless Collaboration | Cost-Efficient Solutions | Faster Time-to-Market
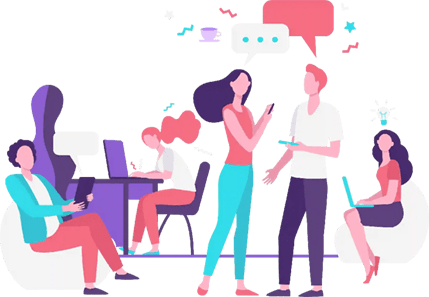
10 Ways Kodexo Labs can help Business Owners Acquire an Edge in Treatment Systems:
In today’s competitive healthcare landscape, automation is key to streamlining processes and delivering exceptional patient care. Automated treatment systems can revolutionise how you manage patient data, personalise treatment plans, and optimise resource allocation. Here’s how Kodexo Labs, an AI software development company can empower your business to acquire a significant edge in this transformative area:
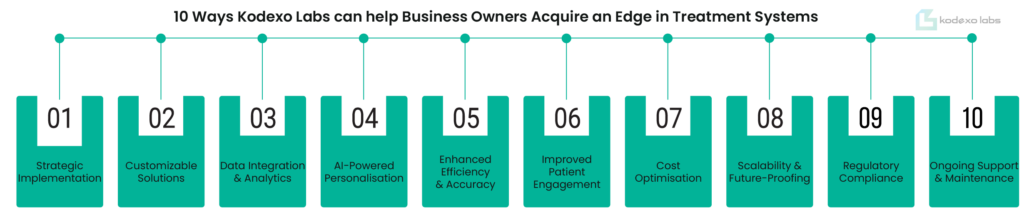
1- Strategic Implementation:
Kodexo Labs boasts a team of experts who can assess your specific needs and identify the most effective automation strategies for your treatment systems. They’ll help you navigate the complexities of integrating automation while ensuring it aligns with your overall business goals
2- Customizable Solutions:
A one-size-fits-all approach doesn’t work in healthcare. Kodexo Labs can develop custom product development solutions tailored to your unique treatment protocols and patient population. This ensures you maximise the benefits of automation while addressing the specific nuances of your practice.
3- Data Integration & Analytics:
Effective automation relies on robust data integration. Kodexo Labs can help seamlessly connect your existing data sources with your automated treatment systems, enabling real-time analysis and informed decision-making.
4- AI-Powered Personalisation:
Kodexo Labs can leverage the power of artificial intelligence to personalise treatment plans within your automated systems. This allows you to deliver more targeted and effective care to each individual patient.
5- Enhanced Efficiency & Accuracy:
Automated treatment systems can significantly reduce manual tasks and human error. Kodexo Labs can help you implement solutions that streamline workflows, free up valuable staff time, and ensure higher treatment accuracy.
6- Improved Patient Engagement:
Automation can facilitate better patient communication and education. Kodexo Labs can integrate features that allow for automated appointment reminders, medication adherence monitoring, and personalised health information delivery.
7- Cost Optimisation:
By streamlining processes and reducing errors, automation can lead to significant cost savings. Kodexo Labs can help you identify areas where automation can optimise resource allocation and improve your bottom line.
8- Scalability & Future-Proofing:
As your business grows, your treatment systems need to keep pace. Kodexo Labs can design scalable automation solutions that can easily adapt to your evolving needs and future expansion.
9- Regulatory Compliance:
The healthcare industry is subject to strict regulations. Kodexo Labs ensures your automated treatment systems comply with all relevant data privacy and security standards.
10- Ongoing Support & Maintenance:
Kodexo Labs understands that successful automation requires ongoing support. They offer comprehensive maintenance packages to ensure your systems continue to operate smoothly and deliver optimal results over the long term.
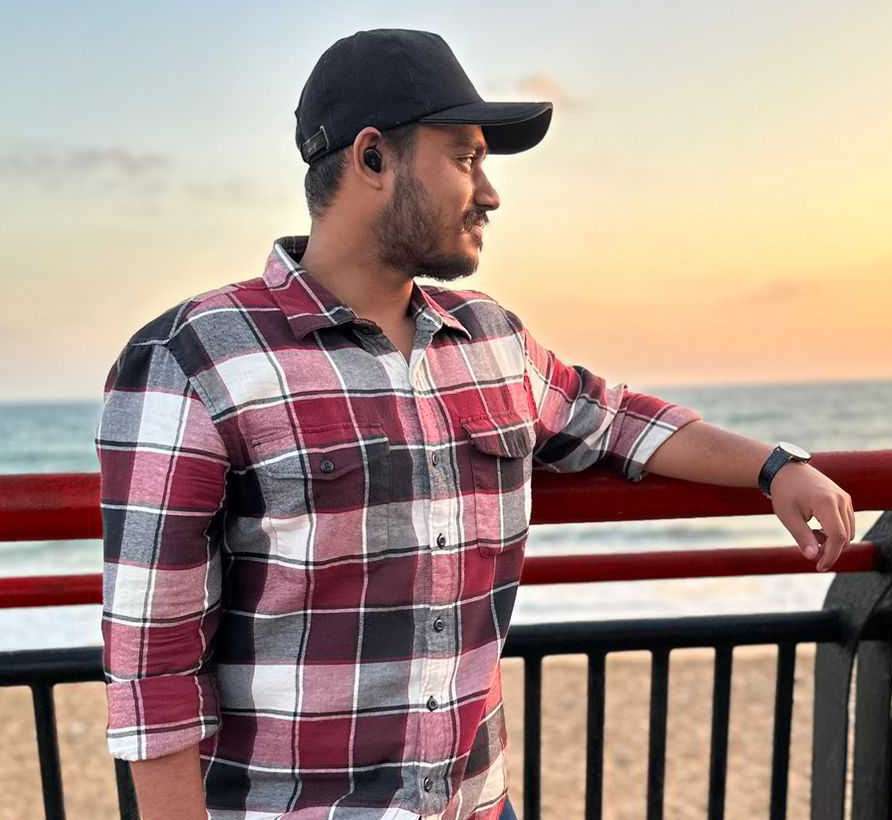
Author Bio
Syed Ali Hasan Shah, a content writer at Kodexo Labs with knowledge of data science, cloud computing, AI, machine learning, and cyber security. In an effort to increase awareness of AI’s potential, his engrossing and educational content clarifies technical challenges for a variety of audiences, especially business owners.