AI Content Recommendation – An Upgrade for Advertising and Marketing Industry
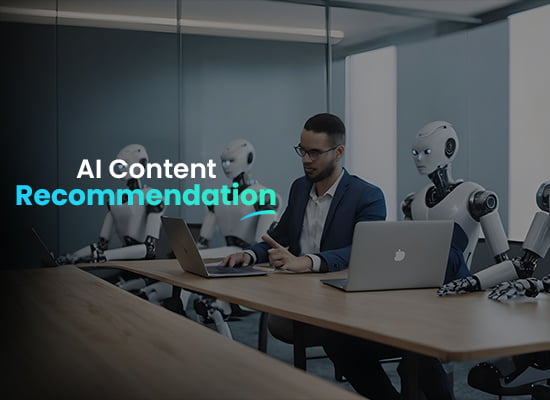
AI Content Recommendation:
AI content recommendation revolutionises the user experience by leveraging sophisticated algorithms to predict and suggest personalised content, enhancing engagement and satisfaction. Through analysing user behaviour and preferences, it offers tailored suggestions, optimising content discovery and consumption.
What is Personalized Content?
Personalized content refers to tailored information or experiences delivered to individuals based on their preferences, behaviours, demographics, or past interactions. In the realm of personalized content marketing, this strategy involves creating and distributing content that resonates with specific audience segments, ultimately aiming to enhance engagement, conversion rates, and customer satisfaction.
At its core, personalized content marketing is about understanding the unique needs and interests of target audiences and crafting content that speaks directly to them. This approach involves leveraging data analytics, user insights, and segmentation techniques to deliver relevant messages and offerings.
One prominent aspect of personalized content marketing is the use of data-driven personalisation. By collecting and analysing data such as browsing history, purchase behaviour, and demographic information, marketers can tailor content to individual preferences. For example, an e-commerce website might recommend products based on a user’s past purchases or browsing activity, providing a more personalised shopping experience.
Another key element of personalized content marketing is dynamic content customisation. This involves dynamically adjusting content elements such as headlines, images, and calls-to-action based on user attributes or behaviour. For instance, an email marketing campaign might use dynamic content blocks to display different product recommendations to subscribers based on their past interactions with the brand.
Personalized content examples abound across various digital channels. In the realm of e-commerce, platforms often employ personalised product recommendations based on user browsing and purchase history. Streaming services like Netflix utilise algorithms to suggest content tailored to each user’s viewing preferences. Similarly, social media platforms employ personalised content algorithms to curate users’ news feeds based on their interests and interactions.
In addition to enhancing the user experience and engagement, personalized content marketing can also drive tangible business results. By delivering targeted messages to specific audience segments, marketers can increase the likelihood of conversion and drive higher ROI on their marketing efforts. Furthermore, personalised content can foster stronger customer relationships by demonstrating an understanding of individual needs and preferences.
Overall, personalized content marketing represents a shift away from one-size-fits-all approaches towards more targeted and relevant communication strategies. By leveraging data and technology to tailor content to individual preferences, marketers can create more meaningful connections with their audiences and drive better business outcomes.
What is AI Content Recommendation?
AI content recommendation is a process where artificial intelligence algorithms analyse user data to suggest personalised content based on their preferences and behaviours. This technology leverages machine learning and data analytics to deliver tailored recommendations across various platforms, such as streaming services, e-commerce websites, social media platforms, and news websites.
At its core, AI personalized content recommendation aims to enhance the user experience by presenting relevant and engaging content to individuals. By collecting and analysing user interactions, including browsing history, past purchases, likes, shares, and demographic information, AI algorithms can understand user preferences and predict their future interests. This enables platforms to deliver content that is more likely to resonate with users, ultimately increasing engagement and satisfaction.
One of the key components of artificial intelligence content recommendation is the use of collaborative filtering techniques. These techniques involve analysing user behaviour and preferences to identify patterns and similarities among users. By understanding these patterns, AI algorithms can recommend content that users with similar preferences have enjoyed in the past. This approach is particularly effective in situations where explicit user feedback (e.g., ratings or reviews) may be limited.
Another important aspect of artificial intelligence content recommendation is the use of content-based filtering. This technique involves analysing the attributes of the content itself, such as genre, topic, or keywords, to recommend similar items. By matching content attributes with user preferences, AI algorithms can suggest relevant content that aligns with users’ interests. Content-based filtering is particularly useful in scenarios where user data may be sparse or when users are seeking specific types of content.
Furthermore, AI content recommendation systems often employ hybrid approaches that combine collaborative filtering and content-based filtering techniques to provide more accurate and diverse recommendations. These hybrid models leverage the strengths of both approaches to overcome their respective limitations and deliver more personalised and relevant suggestions to users.
Overall, artificial intelligence content recommendation plays a vital role in enhancing user engagement and satisfaction across various digital platforms. By harnessing the power of artificial intelligence to analyse user data and predict preferences, these systems enable platforms to deliver personalised content experiences that cater to the unique interests and preferences of each individual user. As AI technology continues to advance, so too will the capabilities of artificial intelligence content recommendation systems, further improving the user experience in the digital landscape.
Seamless Collaboration | Cost-Efficient Solutions | Faster Time-to-Market
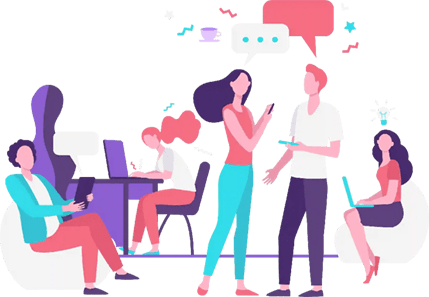
Benefits of AI Content Recommendation:
In today’s digital era, where information overload is a common challenge, AI personalized content recommendation systems have emerged as powerful tools for enhancing user experience and engagement across various platforms. Leveraging advanced algorithms and machine learning techniques, these systems analyse user behaviour, preferences, and contextual data to deliver tailored content suggestions. Here are key points outlining the benefits of AI content recommendation:
Enhanced User Engagement:
AI content recommendation algorithms enable platforms to provide users with personalised content that aligns with their interests and preferences. By presenting relevant articles, videos, products, or services, users are more likely to engage with the platform, leading to increased time spent and improved overall satisfaction.
Improved Content Discovery:
AI-driven recommendation engines help users discover new and diverse content that they may have otherwise overlooked. By analysing user interactions and similarities with others, these systems can suggest relevant content outside of users’ typical preferences, fostering serendipitous discovery and broadening their interests.
Increased Content Consumption:
Through artificial intelligence content recommendation, platforms can entice users to consume more content by presenting them with a continuous stream of items tailored to their tastes. This increased consumption not only benefits users by providing them with more relevant information but also helps content creators and platform owners by driving higher engagement metrics.
Personalized User Experience:
AI personalized content recommendation systems create a customised user experience by delivering content that resonates with each individual’s preferences, browsing history, and demographic information. Whether it’s a news aggregator suggesting articles based on a user’s reading habits or a streaming service recommending movies based on viewing history, personalisation enhances user satisfaction and loyalty.
Optimized Marketing Efforts:
For businesses, artificial intelligence content recommendation offers a powerful tool for targeted marketing and advertising. By analysing user data and behaviour, companies can deliver personalised product recommendations, promotional offers, and advertisements to specific audience segments, increasing the likelihood of conversions and sales.
Enhanced Customer Retention:
Providing users with relevant and engaging content through AI-driven recommendations fosters a sense of loyalty and satisfaction, reducing churn rates and increasing customer retention. When users feel understood and catered to, they are more likely to remain active on the platform and continue consuming its content.
Efficient Resource Allocation:
AI content recommendation systems help platforms optimise resource allocation by prioritising the promotion of content that is likely to generate the highest engagement and user satisfaction. By identifying trending topics, popular items, and individual preferences in real-time, these systems ensure that resources are allocated effectively to maximise impact.
Data-Driven Insights:
Through continuous analysis of user interactions and feedback, AI content recommendation systems generate valuable insights into user preferences, trends, and behaviours. These insights can inform content strategy, product development, and marketing initiatives, enabling organisations to make data-driven decisions that drive growth and innovation.
Tailored Content Delivery:
AI-driven recommendation engines enable platforms to deliver content across various channels and formats, ensuring a seamless and personalised user experience. Whether it’s recommending articles in a news app, suggesting songs on a music streaming service, or highlighting products on an e-commerce website, personalised content delivery enhances user engagement across all touchpoints.
Personalized Content Examples:
Netflix utilises AI algorithms to recommend movies and TV shows based on users’ viewing history, ratings, and preferences, enhancing the streaming experience.
Amazon employs AI-powered recommendation systems to suggest products based on users’ browsing history, purchase behaviour, and demographic data, facilitating personalised shopping experiences.
Spotify leverages machine learning to create personalised playlists, recommend songs, and discover new artists based on users’ listening habits and preferences.
Google News provides personalised news recommendations by analysing users’ interests, reading patterns, and location data, delivering a tailored newsfeed that reflects individual preferences.
YouTube utilises AI algorithms to recommend videos based on users’ watch history, likes, and subscriptions, enhancing content discovery and engagement on the platform.
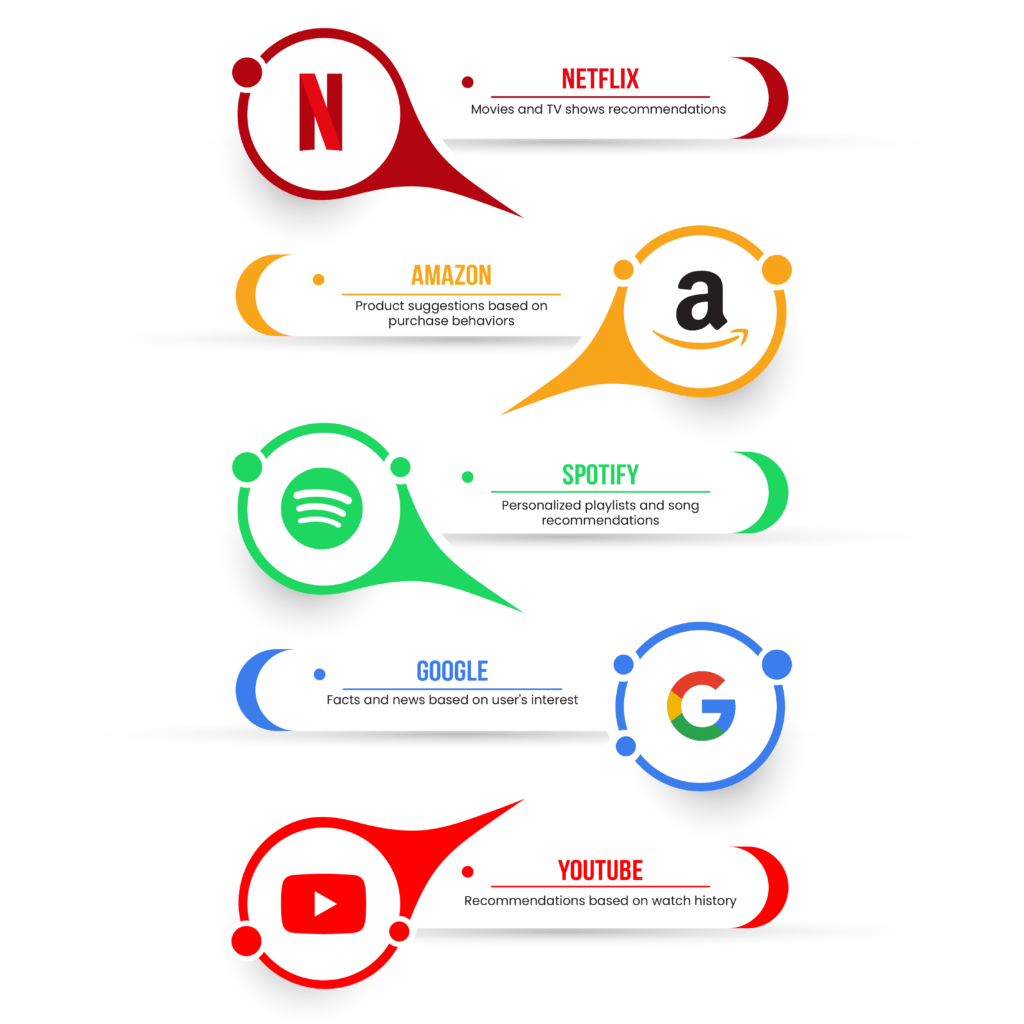
Types of AI Content Recommendation
In the world of digital interaction, AI-driven recommendation systems play an indispensable role in guiding users towards relevant content. These systems leverage advanced algorithms and machine learning techniques to analyse vast datasets, delivering personalised recommendations tailored to individual preferences. Among the various types of AI recommendation systems, content-based filtering stands out as a cornerstone in the field of personalized content marketing.
Content-Based Filtering:
Content-based filtering relies on the intrinsic characteristics of items to generate recommendations that match users’ past interactions and preferences. Leveraging AI personalized content analysis, this approach assesses the attributes of items and users’ historical engagement patterns to predict future preferences. By understanding users’ preferences through their interactions with content, AI personalized content recommendation systems can effectively curate suggestions that align with users’ interests, enhancing user engagement and satisfaction.
Collaborative Filtering:
In addition to content-based filtering, collaborative filtering represents another prominent category in artificial intelligence content recommendation systems. Unlike content-based approaches that focus on item attributes, collaborative filtering leverages the collective wisdom of users’ interactions. By analysing user behaviour and preferences, AI personalized content recommendation systems can identify similarities among users and recommend items that other users with similar tastes have enjoyed. This collaborative approach empowers recommendation systems to offer diverse and personalised content suggestions, fostering a deeper connection between users and the platform.
Hybrid Recommendation System:
Hybrid recommendation systems combine elements of content-based and collaborative filtering to capitalise on the strengths of both approaches. By integrating AI personalized content analysis with collaborative filtering techniques, these systems can deliver recommendations that are not only tailored to individual preferences but also enriched by the collective wisdom of the user community. This hybrid approach allows recommendation systems to overcome the limitations of individual methods, providing more accurate and diverse recommendations that resonate with users’ interests.
Knowledge-Based Recommendation:
Moreover, knowledge-based recommendation systems leverage domain-specific knowledge to enhance the quality of recommendations. By incorporating domain expertise and contextual information, these systems can offer personalised content recommendations that align with users’ preferences and situational needs. AI-driven knowledge-based recommendation systems excel in domains where explicit knowledge about items and user preferences is available, enabling them to deliver highly relevant and tailored suggestions.
Content-Aware Recommendation System:
Context-aware recommendation systems represent a cutting-edge approach to AI personalized content marketing, leveraging contextual information to refine recommendations further. By considering factors such as time, location, and device type, these systems can adapt recommendations based on the user’s current context, enhancing the relevance and utility of the suggested content. Through AI-driven context analysis, recommendation systems can anticipate users’ needs and deliver timely and contextually appropriate recommendations, driving engagement and satisfaction.
AI Content Recommendation Algorithms:
In AI personalized content recommendation, recommendation algorithms play a pivotal role in delivering tailored experiences to users. These algorithms leverage advanced machine learning techniques to sift through vast amounts of data and unearth relevant content that aligns with individual preferences. At the heart of personalized content marketing strategies, these algorithms enable platforms to curate bespoke recommendations for each user, thereby enhancing engagement and driving conversions.
One prominent type of recommendation algorithm utilised in artificial intelligence content recommendation is collaborative filtering. This approach leverages user interactions and similarities to suggest content that others with comparable tastes have enjoyed. Through analysing user behaviour and preferences, collaborative filtering algorithms excel at delivering personalised recommendations, thereby enhancing the overall user experience. Furthermore, these algorithms are adept at adapting to evolving user preferences over time, ensuring the continued delivery of relevant and engaging content.
Another key recommendation algorithm employed in AI personalised content recommendation is content-based filtering. Unlike collaborative filtering, content-based filtering focuses on the intrinsic characteristics of the content itself rather than user interactions. By analysing attributes such as keywords, tags, and metadata, these algorithms recommend content that closely matches a user’s historical preferences. This approach enables platforms to deliver personalised recommendations even for new or niche content, enhancing the discoverability of relevant material for users.
In addition to collaborative filtering and content-based filtering, hybrid recommendation algorithms are gaining traction in the field of AI content recommendation. These algorithms combine elements of both collaborative and content-based filtering to leverage the strengths of each approach. By integrating user behaviour with content attributes, hybrid recommendation algorithms offer a holistic approach to personalised content recommendation, maximising the accuracy and relevance of recommendations.
Furthermore, deep learning techniques are increasingly being employed in AI content recommendation algorithms to extract intricate patterns and insights from vast datasets. Through neural networks and advanced algorithms, deep learning models can discern nuanced user preferences and deliver highly personalised recommendations. This enables platforms to elevate their personalized content marketing strategies, fostering deeper engagement and driving business outcomes.
Seamless Collaboration | Cost-Efficient Solutions | Faster Time-to-Market
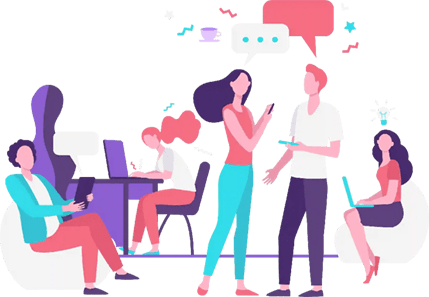
Future Trends and Innovations in AI Content Recommendation:
As the digital landscape continues to evolve, the future of content recommendation is increasingly intertwined with artificial intelligence (AI). AI-driven recommendation systems are poised to revolutionise how personalised content is delivered to users across various platforms. This section delves into some key trends and innovations shaping the future of AI content recommendation.
Personalized Content Examples:
Personalized content examples are becoming more prevalent as AI algorithms refine their ability to analyse user data and preferences. For instance, streaming platforms like Netflix leverage AI to recommend movies and TV shows based on users’ viewing history and preferences. Similarly, e-commerce giants like Amazon utilise AI-powered recommendation systems to suggest products tailored to each user’s browsing and purchasing behaviour. These personalized content examples demonstrate the effectiveness of AI in delivering relevant and engaging experiences to users.
Personalized Content Marketing:
In marketing, personalized content marketing is gaining traction as brands seek to connect with consumers on a more individualised level. AI plays a crucial role in this domain by enabling marketers to deliver targeted content based on user demographics, interests, and past interactions. For instance, email marketing campaigns can utilise AI algorithms to recommend products or services tailored to each subscriber’s preferences, leading to higher engagement and conversion rates. Through personalized content marketing, brands can foster deeper connections with their audience and drive business growth.
AI Personalized Content:
AI personalized content is at the forefront of innovation in content recommendation, leveraging advanced algorithms to deliver highly relevant and tailored experiences to users. These systems analyse vast amounts of user data, including browsing history, search queries, and social interactions, to generate personalised recommendations in real-time. For example, social media platforms employ AI algorithms to curate users’ news feeds based on their interests and behaviours, ensuring that they are exposed to content that resonates with them. By harnessing the power of AI personalized content, platforms can enhance user engagement and satisfaction.
AI Content Recommendation:
AI content recommendation is evolving rapidly, with advancements in machine learning and natural language processing driving its capabilities to new heights. One notable trend is the integration of context-aware recommendation systems, which take into account situational factors such as location, time of day, and device type to deliver more relevant content recommendations. Additionally, multimodal recommendation systems are emerging, leveraging AI to analyse text, images, and audio data simultaneously for a more holistic understanding of user preferences. These innovations in artificial intelligence content recommendation promise to deliver more personalised and immersive experiences to users across diverse platforms.
Conclusion:
AI recommendation systems encompass a diverse array of approaches, each tailored to meet specific use cases and user preferences. From content-based filtering to collaborative and hybrid approaches, these systems leverage AI personalized content analysis to deliver recommendations that resonate with users’ interests and preferences. By harnessing advanced algorithms and machine learning techniques, recommendation systems empower businesses to enhance user engagement, drive conversions, and foster long-term customer relationships in the era of personalized content marketing.
Recommendation algorithms are the cornerstone of AI personalized content recommendation, enabling platforms to deliver tailored experiences to users. By leveraging collaborative filtering, content-based filtering, hybrid approaches, and deep learning techniques, these algorithms empower platforms to curate bespoke recommendations that resonate with individual preferences. As personalized content marketing continues to evolve, recommendation algorithms will play an indispensable role in driving engagement, fostering brand loyalty, and maximising business impact.
The future of AI content recommendation is bright, with personalized content examples, personalized content marketing, AI personalized content, and AI content recommendation at the forefront of innovation. As AI algorithms continue to advance, we can expect to see even more sophisticated recommendation systems that cater to the unique preferences and interests of individual users. By embracing these trends and innovations, businesses can stay ahead of the curve and deliver compelling content experiences that resonate with their audience.
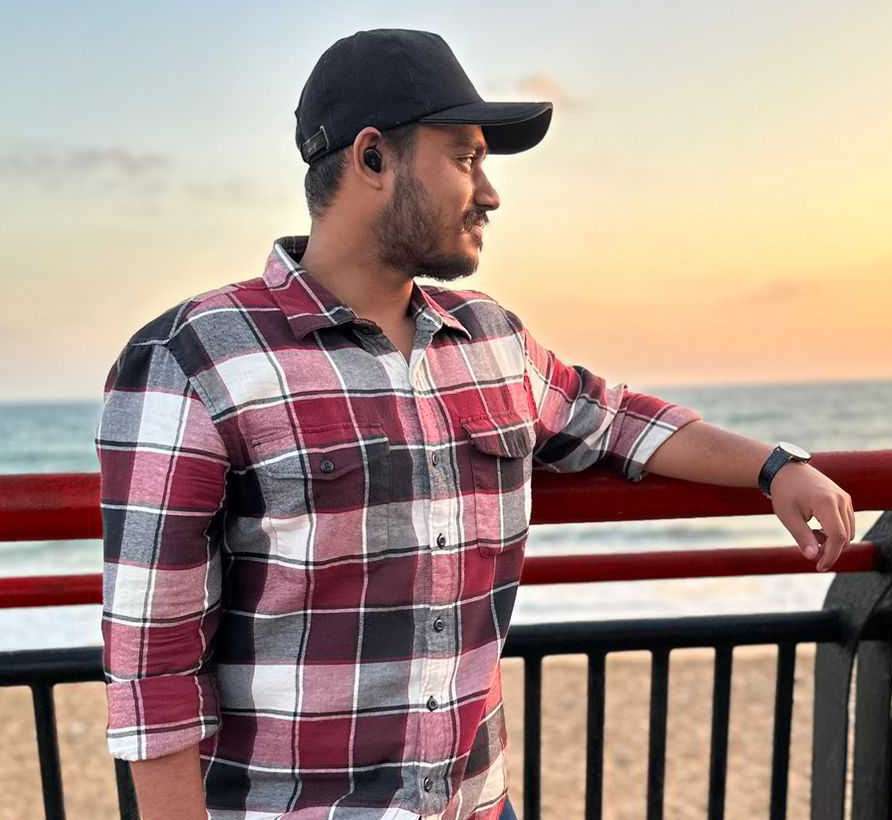
Author Bio
Syed Ali Hasan Shah, a content writer at Kodexo Labs with knowledge of data science, cloud computing, AI, machine learning, and cyber security. In an effort to increase awareness of AI’s potential, his engrossing and educational content clarifies technical challenges for a variety of audiences, especially business owners.