AI in Facial Emotion Recognition – You Can’t Hide Your Smile from AI
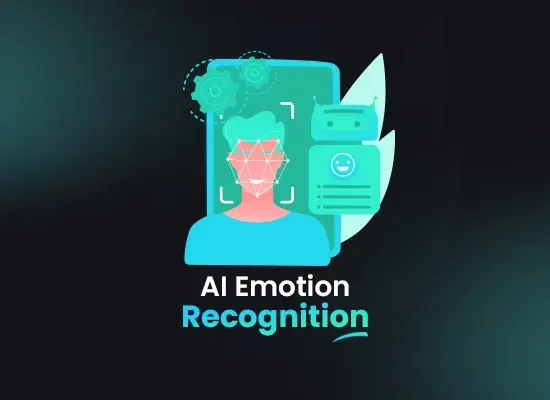
Artificial intelligence is rapidly advancing in its ability to read human emotions. This facial emotion recognition technology, fuelled by deep learning algorithms, analyses facial expressions to decipher a person’s feelings. With applications ranging from education to customer service, AI is poised to transform how we understand and respond to human emotions on a digital level.
What is a Facial Emotion Recognition System?
Facial Emotion Recognition (FER) systems are a type of emotion recognition technology that analyses human faces to infer emotional states. This technology relies on software that can detect and track facial features, like the position of the eyebrows, mouth, and eyes. By analysing these features and their movements, the software attempts to categorise the emotions a person might be feeling.
There are different approaches to Artificial Intelligence for emotion recognition. Some systems focus on analysing a single image, capturing a fleeting expression. Others track expressions over time in videos to gain a more nuanced understanding of a person’s emotional state.
While FER systems are becoming increasingly sophisticated, it’s important to remember that emotions are complex and can be influenced by cultural background and context. This technology should be used with caution and should not be taken as a definitive indicator of a person’s true feelings.
Despite these limitations, AI for emotion recognition has the potential for a wide range of applications. For example, FER systems could be used in customer service applications to improve interaction quality or in educational settings to gauge student engagement.
Seamless Collaboration | Cost-Efficient Solutions | Faster Time-to-Market
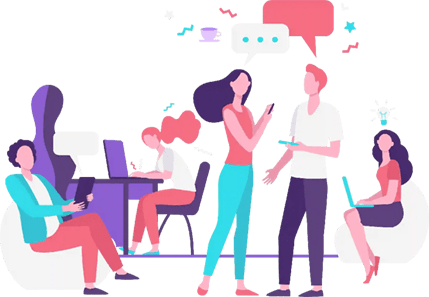
What is the Role of AI Emotion Recognition Technology?
Facial emotion recognition systems are revolutionising the way we understand human emotions through technology. AI and machine learning development plays a critical role in this process. AI for emotion recognition software analyses facial features and expressions to infer a person’s emotional state.
This software is trained on vast amounts of data through data engineering services containing faces paired with labelled emotions. By analysing the position of eyebrows, eyes, and the mouth, the AI can detect patterns associated with happiness, sadness, anger, and other emotions.
Emotion recognition technology is constantly evolving. AI systems can now even identify micro-expressions, fleeting facial changes that can reveal underlying emotions. This ability to go beyond basic expressions makes AI for emotion recognition a powerful tool.
However, it’s important to remember that AI for emotion recognition is still under development. Factors like cultural background and individual differences can influence facial expressions. Therefore, AI for emotion recognition software should be seen as a tool to gain insights, not a definitive measure of someone’s emotions.
History of Evolution by Emotion Recognition Technology:
Facial emotion recognition (FER) technology is a branch of artificial intelligence (AI) that strives to interpret emotions from a person’s face. It has a surprisingly long history, dating back to the 1960s.
The earliest pioneers, like Woodrow Bledsoe, weren’t working with the powerful computers we have today. Back then, facial recognition, the foundation for FER, relied on manually encoding facial features and rudimentary scanners. These early attempts were largely unsuccessful.
The 1970s saw advancements in mapping facial features with more points, but processing power remained a limiting factor. FER truly began to evolve alongside significant growth in computer science.
The late 20th and early 21st centuries saw a boom in AI research, with deep learning techniques playing a transformative role. FER benefited immensely. Algorithms could now analyse vast amounts of facial data, leading to more sophisticated emotion recognition.
Today, FER is a rapidly developing field. While not without limitations, it’s finding applications in various sectors, from human-computer interaction to market research. The future of FER promises even greater accuracy and wider uses.
Current Trends and Future Directions in AI for Facial Emotion Recognition:
Facial emotion recognition (FER) with Artificial Intelligence (AI) is a rapidly developing field. Researchers are using AI, particularly deep learning techniques, to analyse facial expressions and infer emotions. This technology has the potential to revolutionise how we interact with machines and understand human behaviour.
One current trend is the focus on improving accuracy. Machine learning and MLOps are becoming adept at recognizing basic emotions like happiness, sadness, and anger from static images. However, challenges remain in recognizing more nuanced emotions and dealing with factors like lighting variations and head poses.
Researchers are also looking beyond static images. Analysing facial expressions in videos allows for a more dynamic understanding of emotions. Additionally, combining facial recognition with other modalities like voice analysis is another promising area of exploration, aiming for a more comprehensive picture of human emotion.
The future of AI-powered FER involves addressing ethical concerns. Issues around privacy, bias in datasets, and potential misuse of the technology need to be addressed. Additionally, ensuring transparency and explainability in how AI models arrive at their emotional judgments is crucial.
As FER technology matures, we can expect applications in various fields. From improving human-computer interaction to providing insights for AI in education and AI in healthcare, AI-powered facial emotion recognition has the potential to significantly impact our lives.
Comparison with Human Perception of Facial Emotions:
This explores how well machines recognize emotions from faces compared to humans, considering accuracy and factors influencing interpretation.
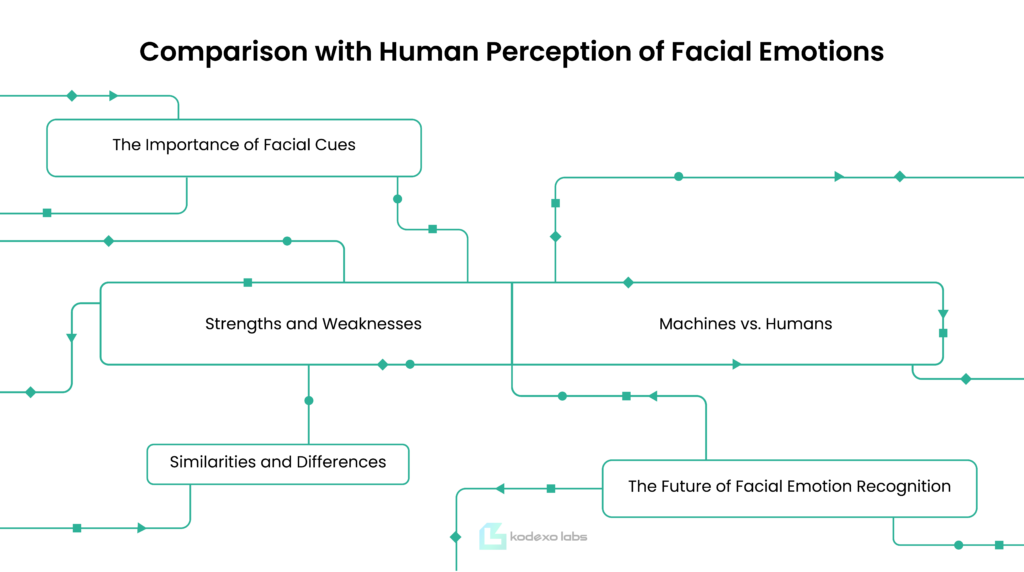
1- The Importance of Facial Cues:
Human faces are incredibly expressive, conveying a wealth of information beyond just identity. Recognizing emotions from facial expressions is crucial for navigating social interactions, understanding intentions, and building rapport. Both humans and machines are adept at this skill but to varying degrees.
2- Strengths and Weaknesses:
Humans excel at interpreting facial expressions in real-world contexts. We consider factors like body language, tone of voice, and cultural nuances. However, our accuracy can be affected by factors like lighting, ambiguity in expressions, and even our own emotional state.
3- Machines vs Humans:
Artificial intelligence (AI) has made significant strides in facial expression recognition. AI can analyse vast amounts of data and identify subtle facial movements. However, AI often struggles with the complexities of human interaction and the role of context in interpreting emotions.
4- Similarities and Differences:
Both humans and AI rely on identifying patterns in facial features like brow position, lip curvature, and eye widening. However, humans use a more holistic approach, integrating emotional cues with situational awareness.
5- The Future of Facial Emotion Recognition:
Research in this field is ongoing, aiming to improve AI’s ability to understand and respond to human emotions. The ultimate goal is to create technology that can seamlessly integrate with our social interactions, enhancing communication and fostering deeper connections.
Advances in Deep Learning for Facial Emotion Recognition:
Facial Emotion Recognition (FER) aims to identify a person’s emotions by analysing their facial expressions in images or videos. This technology has applications in various fields like human-computer interaction, psychology, and education.
Traditionally, FER relied on manually crafted features to identify emotions. Deep learning, a form of artificial intelligence, has revolutionised FER in recent years. Deep learning algorithms, particularly Convolutional Neural Networks (CNNs), can automatically learn these features directly from data, leading to more accurate emotion recognition.
Deep learning advancements have led to significant improvements in FER accuracy. Researchers are now tackling challenges like overfitting, which occurs when models perform well on training data but struggle with unseen data. Techniques like data augmentation and transfer learning are helping to address this issue.
Another challenge is handling variations in facial data, such as lighting, pose, and individual facial characteristics. Deep learning models are being designed to be more robust to these variations, leading to more generalizable emotion recognition across diverse situations.
The field of deep learning for FER is constantly evolving. Researchers are exploring new architectures, incorporating additional data modalities like voice and body language, and focusing on real-world applications that can leverage this technology for better human-computer interaction and emotional understanding.
Algorithms Used by Emotion Recognition Software:
Emotion recognition technology is a developing field in artificial intelligence (AI) that aims to automatically detect a person’s emotions. This technology is often implemented in software programs called emotion recognition software. AI for emotion recognition software can analyse various data points to infer emotional states. These data analytics can include facial expressions, vocal tones, and even word choice in text.
By employing a range of algorithms, emotion recognition software extracts features from the data it analyses. Some common algorithms used for facial expression recognition include Support Vector Machines (SVMs) and Convolutional Neural Networks (CNNs). For vocal analysis, Mel-Frequency Cepstral Coefficients (MFCCs) are frequently used for feature extraction.
Once features are extracted, the software employs classification algorithms to categorise the emotions. Common classification algorithms include k-Nearest Neighbours (kNN) and Naive Bayes. These algorithms rely on training data that consists of labelled examples, where emotions are explicitly identified alongside the corresponding data (facial expressions, speech patterns, etc.).
The accuracy of emotion recognition software depends on the quality of the algorithms used and the training data. While AI for emotion recognition is constantly evolving, it’s important to remember that emotions are complex and influenced by cultural background and individual experiences. As such, emotion recognition software should be used with caution and with an understanding of its limitations.
Seamless Collaboration | Cost-Efficient Solutions | Faster Time-to-Market
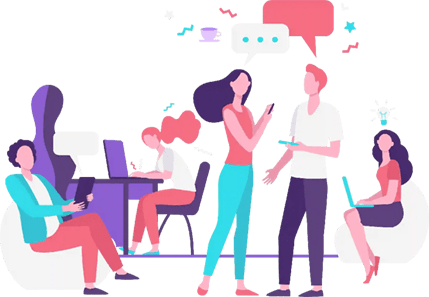
Benefits of AI Emotion Recognition:
Artificial intelligence (AI) is making waves in many fields, and emotion recognition is one of its most fascinating applications. AI emotion recognition technology analyses facial expressions, vocal tones, and even written text to understand a person’s emotional state. This technology is still under development, but it has the potential to revolutionise the way we interact with machines and each other.
Emotion recognition software can be a powerful tool for businesses. By understanding customer sentiment through facial expressions during interactions or analysing tones in written reviews, companies can improve customer service, tailor marketing campaigns, and develop more user-friendly products. Imagine a virtual assistant that adjusts its communication style based on your emotional state, providing a more empathetic and helpful experience.
AI emotion recognition can also have a significant impact on education and healthcare. Emotion recognition software can help teachers identify students who are struggling to understand concepts or feeling overwhelmed. In healthcare, this technology has the potential to detect signs of depression or anxiety early on, allowing for earlier intervention and improved patient outcomes.
Law enforcement is another area that could benefit from AI emotion recognition. Security systems that analyse facial expressions in public places could potentially help identify individuals who may pose a threat. However, it’s important to remember that AI emotion recognition is not foolproof, and ethical considerations around privacy and bias need to be addressed before widespread adoption.
Conclusion:
AI emotion recognition shows promise, but challenges remain. Cultural differences, complexity of emotions, and limited data accuracy require further development for reliable use. The need for AI in this technology advances with time and promises a bright future for the tech giants. This technology not only benefits businesses, but can also be implemented among other AI models to provide a personalised experience to its users.
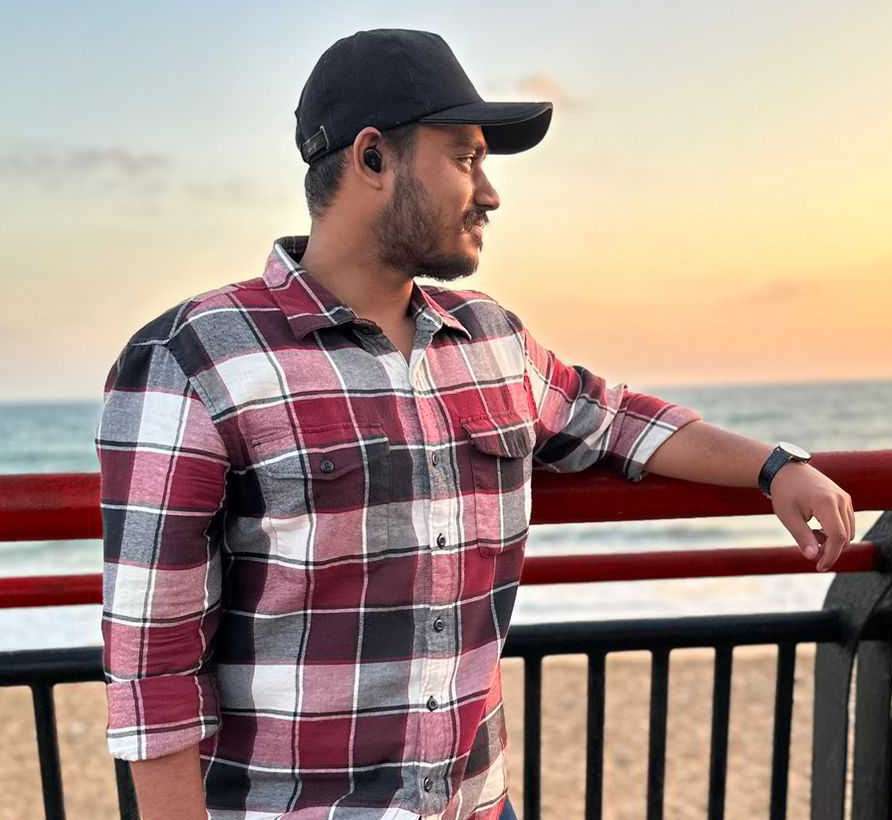
Author Bio
Syed Ali Hasan Shah, a content writer at Kodexo Labs with knowledge of data science, cloud computing, AI, machine learning, and cyber security. In an effort to increase awareness of AI’s potential, his engrossing and educational content clarifies technical challenges for a variety of audiences, especially business owners.