AI in Clinical Trial Optimization – Healthcare is now Optimized with Clinically Automated Software
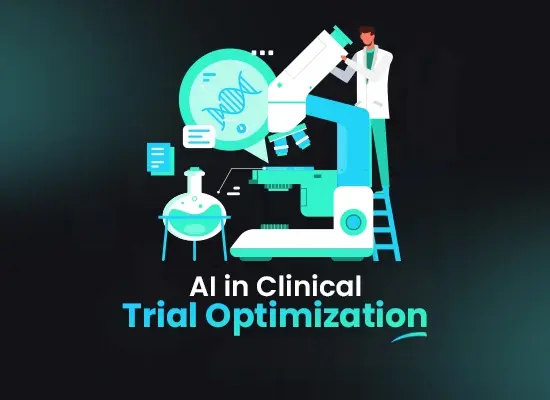
Contents
Clinical trials are crucial for developing new medical treatments, but they are often slow, expensive, and struggle to enroll enough participants. Artificial intelligence (AI) is emerging as a game-changer in this field, offering a suite of tools to optimize clinical trials at every stage. From designing more efficient studies to streamlining patient recruitment and analyzing data, AI has the potential to revolutionize how we bring new therapies to patients.
What is Clinical Trial Optimization?
Clinical trial optimization is a broad term encompassing various strategies designed to make clinical research studies more efficient, effective, and successful. Traditionally, clinical trials have faced challenges like slow enrollment rates, high costs, and potential delays. Clinical trial optimization tackles these issues by leveraging technological advancements and innovative approaches.
One key area of focus in clinical trial optimization is patient recruitment. Here, machine learning in healthcare and clinical AI solutions play a crucial role. By analyzing vast datasets of patient medical records and demographics, these AI-powered tools can identify potential participants who meet the specific eligibility criteria of a trial. This targeted approach streamlines the recruitment process, saving time and resources for researchers.
Clinical trial optimization also involves optimizing the design and execution of the trial itself. Automated clinic software and clinic automation tools can significantly improve data collection, management, and analysis. This not only reduces the burden on clinical staff but also ensures data accuracy and consistency. Additionally, advanced statistical methods and machine learning algorithms can be used to analyze clinical trial data in real-time, allowing for early identification of trends or potential issues. This facilitates proactive adjustments to the trial design or protocols, maximizing the chances of success.
Ultimately, clinical trial optimization aims to bring new treatments and therapies to patients faster and more efficiently. By utilizing advancements like clinical AI solutions, machine learning in healthcare, and clinic automation, researchers can conduct more streamlined and effective clinical trials, paving the way for improved healthcare outcomes.
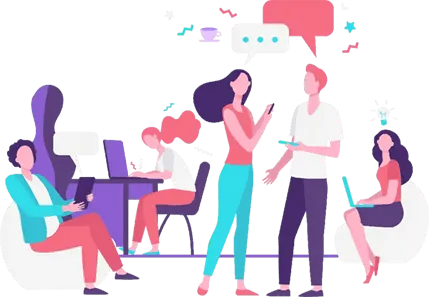
What is the Role of AI in Clinical Trial Optimization?
Clinical trial optimization is a critical area in the development of new drugs and therapies. Traditionally, this process has been fraught with challenges, including slow enrollment rates, high costs, and difficulties in meeting diversity goals. However, the emergence of Artificial Intelligence (AI) is revolutionizing the landscape of clinical trial optimization, offering a powerful set of tools to streamline processes and improve efficiency.
Clinical AI solutions are transforming how clinical trials are designed, conducted, and analyzed. Machine learning algorithms can be used to analyze vast amounts of patient data from electronic medical records (EMRs) and other sources. This data can then be leveraged to identify potential participants who meet the specific eligibility criteria for a trial. Automated clinic software can further streamline the process by automating patient screening and enrollment tasks, reducing the administrative burden on clinical research staff.
The impact of AI implementation extends beyond just patient recruitment. Machine learning can be used to optimize trial design by identifying the most effective treatment arms and minimizing the number of patients needed to achieve statistically significant results. Additionally, AI can be used to monitor trial progress in real-time, identifying potential problems early on and allowing for course correction. This proactive approach can help to ensure that trials stay on track and deliver meaningful results.
Clinic automation powered by AI is another key aspect of clinical trial optimization. By automating repetitive tasks such as data entry and scheduling, clinical research associates (CRAs) can free up their time to focus on more patient-centric activities. This not only improves efficiency but also allows for a more personalized patient experience.
Overall, the role of AI in clinical trial optimization is significant and continuously evolving. As clinical AI solutions become more sophisticated and widely adopted, we can expect to see a dramatic improvement in the efficiency and effectiveness of clinical trials. This will ultimately lead to faster development of new treatments and improved patient care.
5 Uses of Machine Learning in Healthcare:
Machine learning in healthcare is rapidly transforming the medical field, offering a plethora of benefits for both patients and healthcare providers. Here, we explore five key uses of machine learning that are making a significant impact:
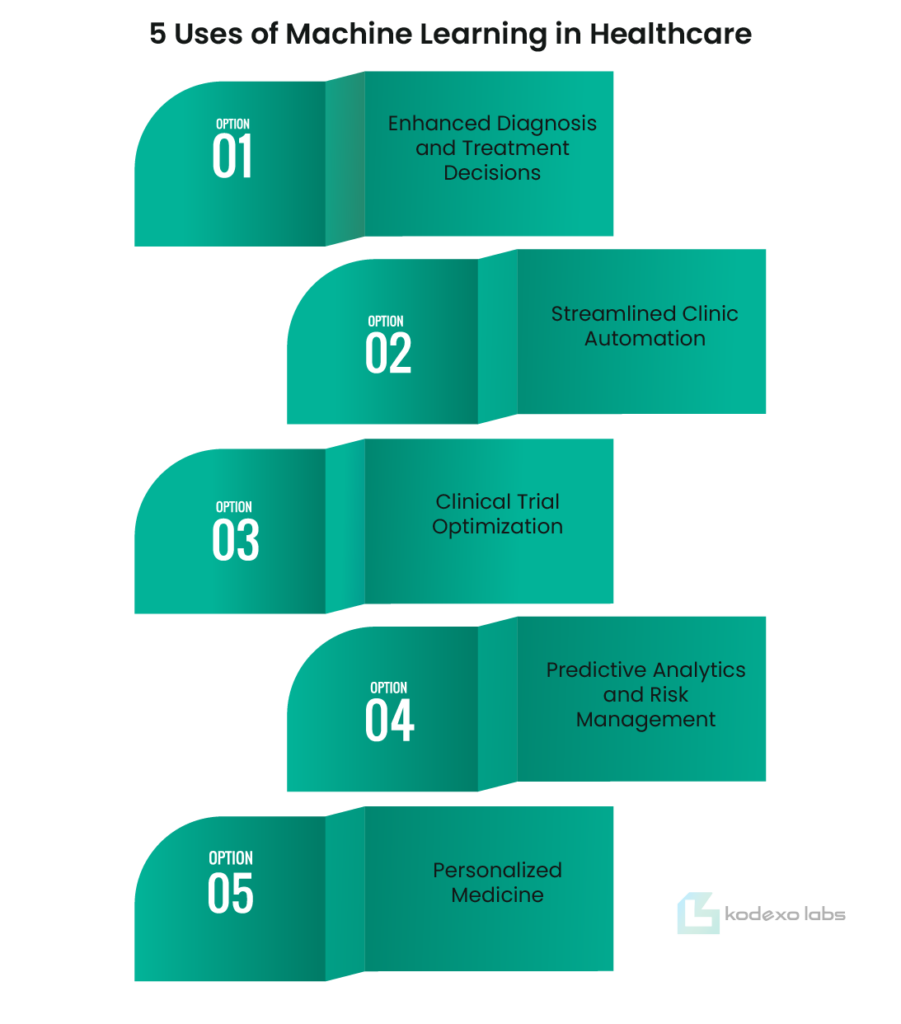
1. Enhanced Diagnosis and Treatment Decisions:
Machine learning algorithms can analyze vast amounts of medical data, including patient records, imaging results, and genetic information. This allows for the identification of patterns and trends that might be missed by the human eye. By leveraging this data analytics, clinical AI solutions can assist doctors in making more accurate diagnoses and recommending personalized treatment plans.
2. Streamlined Clinic Automation:
Clinic automation through Machine Learning is revolutionizing workflows. Automated clinic software can handle tasks like appointment scheduling, insurance verification, and medication reminders. This frees up valuable time for doctors and nurses, allowing them to focus on providing high-quality care to their patients.
3. Clinical Trial Optimization:
Machine learning can significantly improve the efficiency of clinical trials. By analyzing patient data and identifying potential candidates, machine learning can optimize participant selection, leading to faster and more targeted trials. This not only reduces costs but also accelerates the development of new drugs and treatments.
4. Predictive Analytics and Risk Management:
Machine learning algorithms can analyze medical data to predict a patient’s risk of developing certain diseases. This allows for early intervention and preventative measures, potentially improving patient outcomes. Additionally, machine learning can be used to identify patients at high risk of complications during hospitalization, enabling healthcare providers to take necessary precautions.
5. Personalized Medicine:
Machine learning is paving the way for a future of personalized medicine. By analyzing a patient’s unique genetic makeup and medical history, machine learning algorithms can tailor treatment plans to individual needs. This personalized approach can lead to more effective treatments and improved patient outcomes.
The applications of machine learning in healthcare are vast and constantly evolving. As machine learning technology continues to develop, we can expect to see even more innovative clinical AI solutions and automated software emerge, further revolutionizing the way healthcare is delivered.
What is the Role of Generative AI in Clinic Automation?
Clinic automation is rapidly transforming the healthcare landscape. At the forefront of this change is generative AI, a powerful branch of machine learning that can not only analyze data but also create entirely new content. This technology holds immense potential to streamline workflows, improve efficiency, and ultimately enhance patient care.
Generative AI development can play a significant role in automating various administrative tasks within clinics. Imagine automated clinic software that utilizes generative AI to handle appointment scheduling and rescheduling. This would free up valuable time for clinic staff, allowing them to focus on more critical tasks. Additionally, generative AI can be integrated with electronic health records (EHR) systems to automate data entry and generate reports, further reducing administrative burdens.
Beyond administrative tasks, generative AI integration is making waves in the world of clinical AI solutions. By analyzing vast amounts of medical data, generative AI can assist with tasks such as identifying potential patients for clinical trials. This can significantly improve clinical trial optimization by allowing researchers to target the most suitable participants, leading to faster and more effective drug development.
Machine learning in healthcare has already shown promise in areas like disease diagnosis and treatment planning. Generative AI builds upon this foundation by offering the ability to generate personalized treatment plans and educational materials for patients. This can empower patients to take a more active role in their health and improve overall treatment outcomes.
The integration of generative AI in healthcare for clinic automation is still in its early stages. However, the potential benefits are undeniable. By automating tasks, optimizing clinical trials, and personalizing patient care, generative AI has the power to revolutionize the way clinics operate and ultimately improve the lives of patients everywhere. As machine learning in healthcare continues to evolve, so too will the capabilities of generative AI, pushing the boundaries of clinic automation and ushering in a new era of healthcare efficiency.
How is Deep Learning (DL) beneficial for Automated Clinic Software?
The healthcare industry is undergoing a digital revolution, and machine learning, particularly deep learning (DL), is at the forefront of this transformation. Clinic automation is a key area benefiting from this powerful technology. By integrating DL into automated clinic software, healthcare providers can unlock a multitude of advantages that improve patient care, streamline workflows, and optimize clinic operations. Here’s how deep learning brings significant value to automated software:
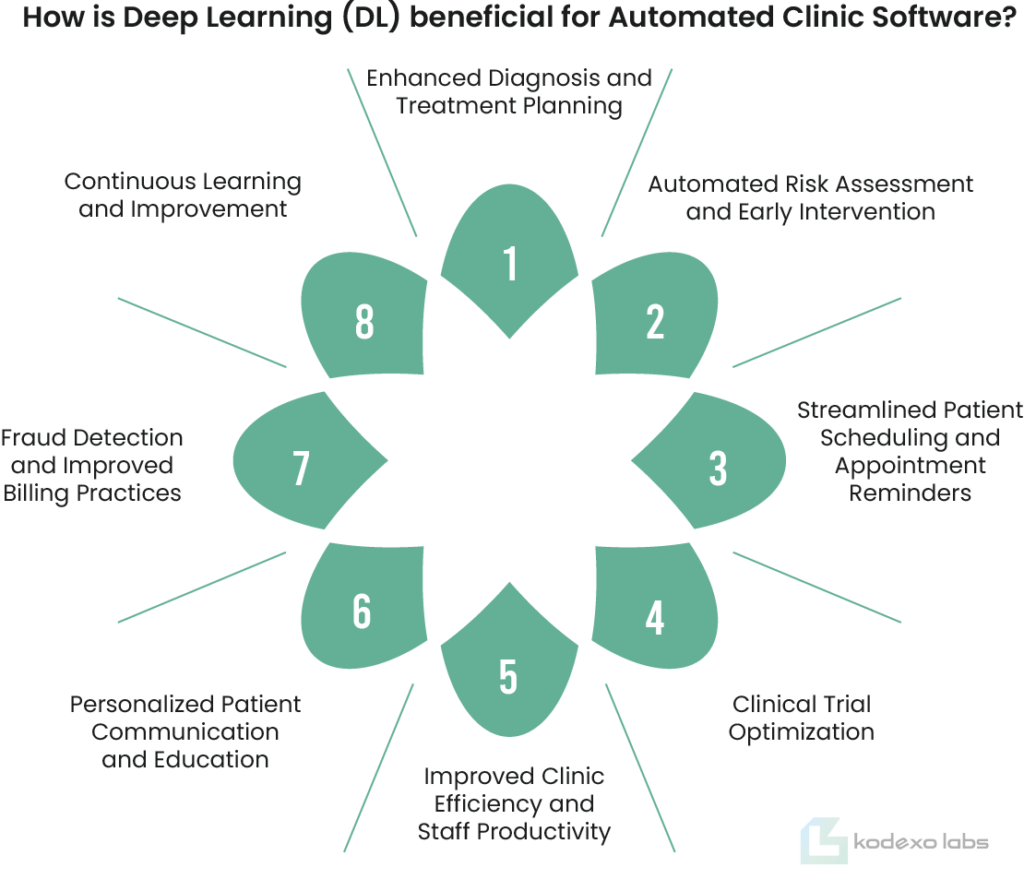
Enhanced Diagnosis and Treatment Planning:
Deep learning algorithms can analyze vast amounts of medical data, including patient records, imaging results, and clinical studies. This allows them to identify patterns and relationships that may be missed by the human eye. This translates to more accurate diagnoses, personalized treatment plans, and improved clinical decision-making.
Automated Risk Assessment and Early Intervention:
DL models can be trained to predict a patient’s risk of developing certain diseases. This enables automated clinic software to flag high-risk individuals, prompting earlier interventions and potentially preventing complications.
Streamlined Patient Scheduling and Appointment Reminders:
Deep learning can analyze historical data to optimize patient scheduling. This includes predicting appointment durations, identifying peak clinic hours, and suggesting optimal appointment times. Additionally, DL-powered automated reminders can improve patient adherence to appointments, reducing missed visits and improving overall clinic efficiency.
Clinical Trial Optimization:
Deep learning plays a crucial role in clinical AI solutions. By analyzing patient data and medical records through data engineering service, DL helps identify suitable candidates for clinical trials. This not only expedites the trial process but also ensures a better match between patients and potential treatments.
Improved Clinic Efficiency and Staff Productivity:
Automated clinic software with deep learning capabilities can automate various administrative tasks, such as appointment scheduling, insurance verification, and data entry. This frees up valuable time for clinic staff, allowing them to focus on providing better patient care.
Personalized Patient Communication and Education:
Deep learning can be used for AI chatbot development that answer patients’ questions, provide educational materials, and offer appointment reminders. This personalized approach to patient communication improves patient engagement and empowers them to take a more active role in their health.
Fraud Detection and Improved Billing Practices:
Deep learning algorithms can analyze billing data to identify potential fraudulent claims. This not only protects clinics from financial losses but also ensures accurate and efficient billing practices.
Continuous Learning and Improvement:
Deep learning models are constantly evolving as they are exposed to new data. This allows automated clinic software to continuously learn and improve its functionalities, ensuring that clinics remain at the forefront of technological advancements in healthcare.
By using the power of deep learning, automated clinical software is poised to revolutionize healthcare delivery. From enhanced patient care to optimized clinic operations, the benefits are far-reaching. As deep learning technology continues to evolve, we can expect even more innovative applications to emerge, shaping the future of clinic automation and patient care.
How can Business Owners benefit from Neural Network (NN) in Clinical Trial Optimization?
Clinical trials are the backbone of bringing new drugs and treatments to market. However, they are notoriously expensive and time-consuming. This is where Neural Networks (NNs), a type of artificial intelligence (AI), come in. By leveraging NNs, business owners in the pharmaceutical and healthcare industries can significantly optimize clinical trials, leading to substantial benefits.
One key advantage of NNs is their ability to analyze vast amounts of complex data. Clinical trials generate a wealth of information, including patient demographics, medical history, treatment responses, and potential side effects. NNs can sift through this data to identify patterns and trends that would be difficult, if not impossible, for humans to detect. This allows business owners to make more informed decisions about trial design, patient selection, and resource allocation.
For instance, NNs can be used to predict which patients are most likely to respond positively to a new treatment. This can help business owners target their trials more effectively, reducing the number of participants needed and accelerating the approval process. Additionally, NNs can be used to identify potential safety risks early on, allowing for course correction and mitigating the chances of trial delays or failures.
Overall, by optimizing clinical trials with NNs, business owners can achieve significant cost savings and get new products to market faster. This translates to a stronger competitive edge and increased profitability. Furthermore, successful clinical trials ultimately lead to better treatments for patients, creating a positive impact on public health.
7 Uses of Modern Clinical AI Solutions:
The field of medicine is undergoing a revolution with the integration of artificial intelligence (AI) into clinical practices. Modern clinical AI solutions leverage various AI techniques, including AI and machine learning development and deep learning algorithms, to analyze vast amounts of medical data and assist healthcare professionals in numerous ways. Here, we explore seven key uses of modern clinical AI solutions:
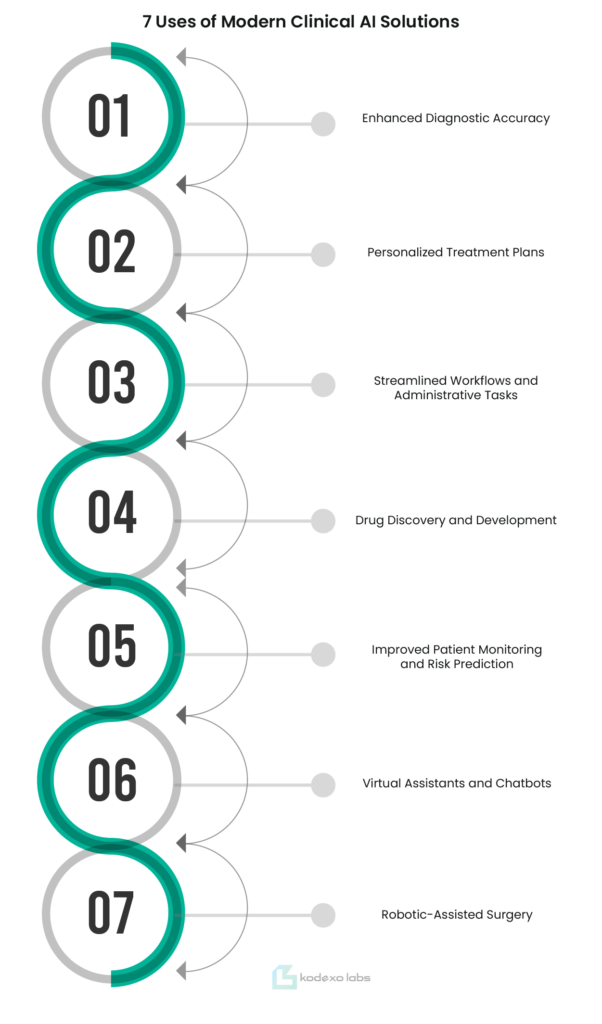
1- Enhanced Diagnostic Accuracy:
AI algorithms can analyze medical images like X-rays, CT scans, and mammograms with exceptional precision, aiding in early disease detection and improved diagnosis. AI can identify subtle patterns invisible to the human eye, potentially leading to faster and more accurate diagnoses.
2- Personalized Treatment Plans:
By analyzing a patient’s medical history, genetic data, and current health status, AI can help create personalized treatment plans. This allows for a more targeted approach to healthcare, potentially leading to better treatment outcomes and reduced side effects.
3- Streamlined Workflows and Administrative Tasks:
AI can automate various administrative tasks in healthcare settings, such as scheduling appointments, managing patient records, and generating reports. This frees up valuable time for medical professionals, allowing them to focus on patient care.
4- Drug Discovery and Development:
AI is accelerating drug discovery by analyzing vast datasets of molecular structures and patient information. This can lead to the identification of new drug targets and the development of more effective medications.
5- Improved Patient Monitoring and Risk Prediction:
AI can continuously monitor a patient’s vital signs and medical data, identifying potential health risks and complications early on. This allows for preventive measures and timely interventions, potentially improving patient outcomes.
6- Virtual Assistants and Chatbots:
AI-powered virtual assistants and chatbots can provide patients with 24/7 access to information and support. These tools can answer basic medical questions, schedule appointments, and offer guidance on managing chronic conditions.
7- Robotic-Assisted Surgery:
AI is playing an increasingly important role in robotic-assisted surgery. AI algorithms can guide robotic instruments with high precision, minimizing human error and improving surgical outcomes.
These are just a few examples of how modern clinical AI solutions are transforming the healthcare landscape. As AI technology continues to evolve, we can expect even more innovative applications to emerge, ultimately leading to a future of more efficient, personalized, and effective healthcare.
How does Kodexo Labs help Business Owners Equip themselves with Automated Clinic Software?
In today’s fast-paced healthcare landscape, clinic efficiency is paramount. This is where Kodexo Labs comes in, offering a suite of solutions to empower business owners with automated clinic software. But how exactly does Kodexo Labs, an AI software development company assist business owners in this process? Let’s explore ten key ways:
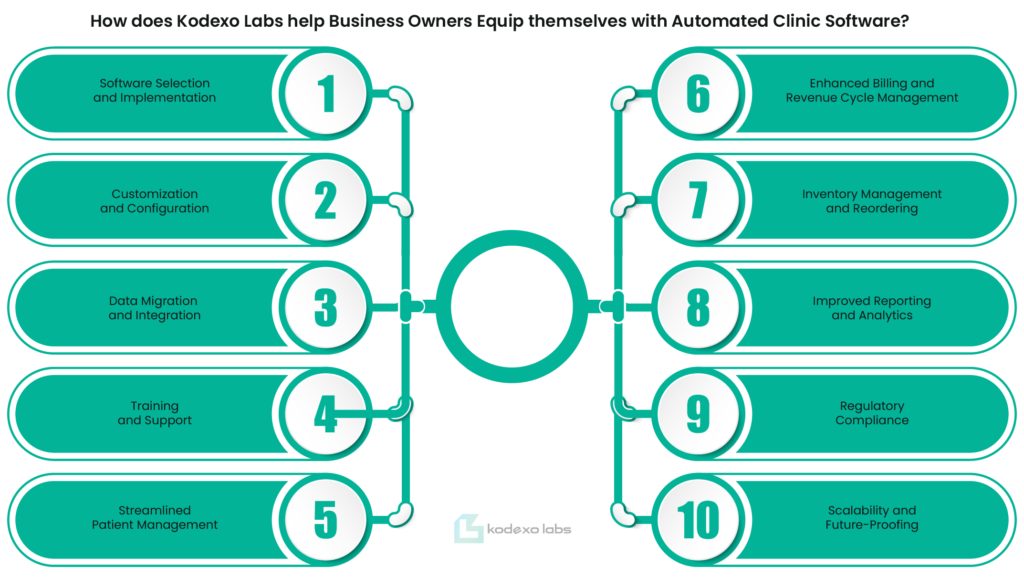
Software Selection and Implementation:
Choosing the right clinic management software can be overwhelming. Kodexo Labs acts as a guide, helping business owners assess their needs and identify software that best suits their clinic’s size, specialty, and budget. They then assist with seamless implementation, ensuring a smooth transition to automated workflows.
Customization and Configuration:
Not all clinics operate the same way. Kodexo Labs understands this and provides custom product development options for the chosen software. This ensures the software aligns perfectly with the clinic’s unique practices and optimizes clinic-specific workflows.
Data Migration and Integration:
Transitioning from manual systems to automated software can be daunting, especially when it comes to data migration. Kodexo Labs offers data migration services, ensuring a secure and efficient transfer of patient information and clinic records to the new software.
Training and Support:
Even the most user-friendly software requires proper training. Kodexo Labs provides comprehensive training for clinic staff, ensuring they can leverage the software’s full potential. Additionally, they offer ongoing support to address any questions or challenges that may arise.
Streamlined Patient Management:
Automated clinic software streamlines patient management by automating tasks like appointment scheduling, appointment reminders, and patient intake forms. Kodexo Labs helps configure these features within the software, leading to improved patient experience and reduced administrative burden. Automated clinic software streamlines patient management by automating tasks like appointment scheduling, appointment reminders, and patient intake forms.
Enhanced Billing and Revenue Cycle Management:
Revenue cycle management is crucial for any clinic’s financial health. Kodexo Labs helps configure billing modules within the software and automates tasks like insurance claim submissions and follow-ups. This ensures timely payments and improves cash flow.
Inventory Management and Reordering:
Automated clinical software can track inventory levels and automate reordering when supplies run low. Kodexo Labs helps set up these features, ensuring clinics never face stockouts that can disrupt patient care.
Improved Reporting and Analytics:
Data-driven decision-making is essential for clinic growth. Kodexo Labs helps configure reporting modules within the software, providing valuable insights into clinic performance, patient demographics, and treatment trends.
Regulatory Compliance:
The healthcare industry has strict regulations regarding data privacy and security. Kodexo Labs ensures the chosen software adheres to all relevant regulations and helps clinics implement best practices for data protection.
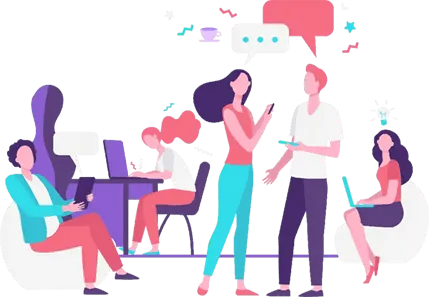
Scalability and Future-Proofing:
Clinic needs can evolve over time. Kodexo Labs helps choose software that is scalable and can accommodate future growth. They also provide guidance on how to leverage the software’s advanced features as the clinic expands.
By partnering with Kodexo Labs, business owners can equip themselves with the tools and expertise needed to implement and leverage automated software effectively. This translates to a more efficient clinic operation, improved patient satisfaction, and a thriving healthcare practice.
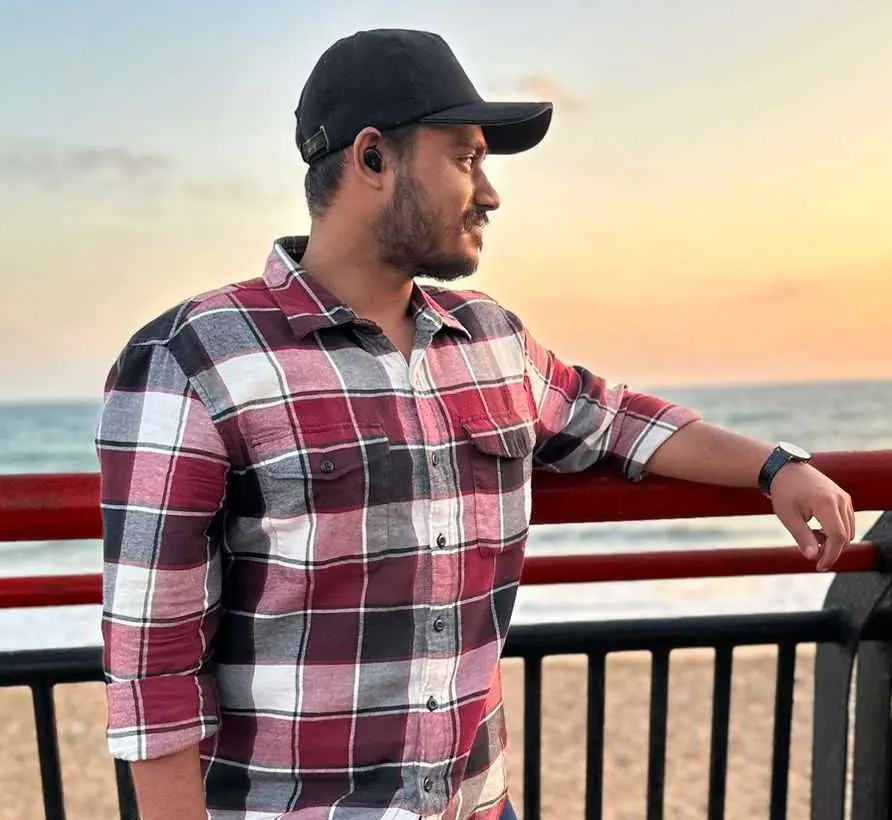