Meta SAM 2 – What Sets it Apart From its Predecessor or Competitors?
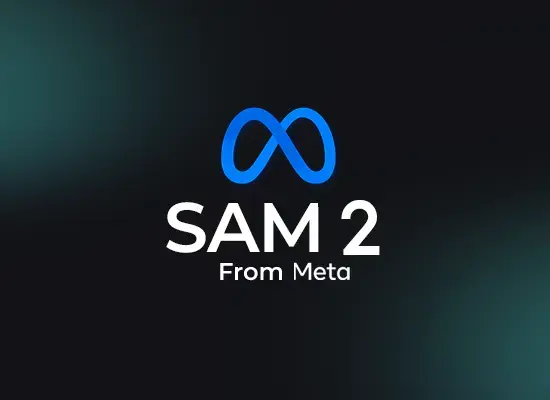
Contents
Meta’s SAM 2 represents a significant leap forward in image segmentation technology. Building upon the foundation laid by its predecessor, SAM 2 introduces groundbreaking capabilities that set it apart from both earlier versions and competing models. This article delves into the key advancements that make SAM 2 a standout in the field, exploring how it pushes the boundaries of object detection and image analysis.
What is Meta SAM 2?
Meta SAM 2, or Segment Anything Model 2, is the latest advancement from Meta in the field of computer vision, specifically targeting the complex task of object segmentation in both images and videos. This model represents a significant leap forward from its predecessor, SAM, by extending its capabilities to real-time video processing, allowing for accurate object segmentation across entire video sequences.
The key feature of Meta SAM 2 is its ability to segment any object and track it consistently throughout all frames of a video in real-time. This is made possible through a combination of sophisticated machine learning techniques, including a two-way transformer architecture and a newly introduced “occlusion head,” which enhances the model’s ability to handle scenarios where objects may be temporarily obscured or disappear from view. The model’s ability to recall information from previous frames makes it especially effective in dynamic environments, where objects might move quickly or change appearance.
One of the most groundbreaking aspects of Meta SAM 2 is its training on the Segment Anything Video (SA-V) dataset, which is the largest and most diverse video segmentation dataset ever created. This dataset includes over 50,000 videos and nearly 36 million segmentation masks, providing the model with extensive real-world scenarios from across the globe. This extensive training allows Meta SAM 2 to outperform previous models in both accuracy and speed, making it a state-of-the-art solution for tasks that require real-time object recognition and tracking.
Meta SAM 2’s applications are vast and varied. It is expected to revolutionize video editing by enabling quick and precise modifications to specific objects within video frames. This model is also poised to enhance augmented reality (AR) and virtual reality (VR) experiences by allowing for more realistic interactions between virtual and real-world objects. Furthermore, Meta SAM 2 shows promise in specialized fields such as autonomous driving, where it can assist in identifying pedestrians, vehicles, and other critical objects in real time, as well as in medical imaging and environmental monitoring, where accurate and reliable segmentation is crucial.
In summary, Meta SAM 2 is not just an incremental update but a comprehensive overhaul that broadens the horizons of what is possible in visual segmentation. Its impact is likely to be felt across multiple industries, from entertainment and social media to scientific research and beyond (according to Ultralytics).
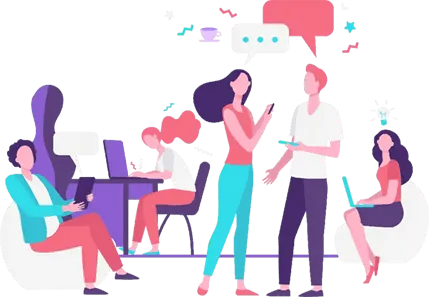
What are the Key Takeaways for Meta SAM 2?
Meta Segment Anything Model 2 (Meta SAM 2) represents a significant advancement in the field of object segmentation, specifically tailored for both images and videos. This innovative model extends the capabilities of its predecessor by introducing a memory module, enabling consistent object tracking across video frames, even in challenging scenarios. Here are the nine key takeaways for Meta SAM 2:
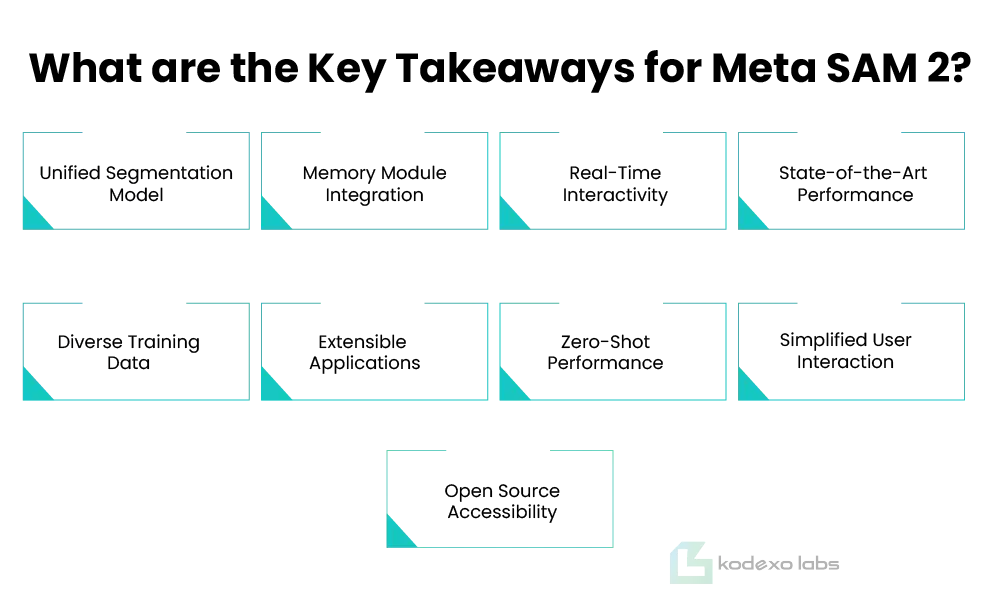
1- Unified Segmentation Model:
Meta SAM 2 unifies object segmentation for both images and videos, offering a versatile tool for various applications.
2- Memory Module Integration:
The addition of a per-session memory module allows for accurate tracking of objects across multiple video frames.
3- Real-Time Interactivity:
SAM 2 supports streaming inference, ensuring real-time, interactive applications.
4- State-of-the-Art Performance:
It outperforms existing models in object segmentation, particularly in videos, enhancing efficiency and accuracy.
5- Diverse Training Data:
Meta SAM 2 was trained on a vast and geographically diverse dataset, improving its robustness across different environments.
6- Extensible Applications:
The model’s outputs can be integrated into other Artifical Intelligence (AI) systems, broadening its applicability.
7- Zero-Shot Performance:
SAM 2 delivers strong results even in unseen scenarios, thanks to its robust design.
8- Simplified User Interaction:
With minimal prompts, users can achieve precise segmentation, making it accessible to a broader audience.
9- Open Source Accessibility:
Meta SAM 2 is open-sourced, allowing researchers and developers to explore and extend its capabilities.
These takeaways highlight the comprehensive capabilities of SAM 2, making it a cutting-edge tool in the field of AI-driven object segmentation.
How is Meta SAM 2 Different from its Predecessors?
Meta SAM 2 represents a significant evolution from its predecessor, SAM 1, primarily through its expanded capabilities and performance improvements in object segmentation tasks. Unlike the original SAM, which focused exclusively on image segmentation, Meta SAM 2 extends these capabilities to video, allowing it to perform real-time, promptable object segmentation across both images and video frames. This advancement makes Meta SAM 2 a more versatile and powerful tool for various applications, from video editing to complex scientific analysis.
One of the key differences with Meta SAM 2 is its enhanced performance in video object segmentation. The model introduces a sophisticated memory mechanism, including a memory encoder and memory bank, which allows it to recall information about objects across different frames in a video. This enables consistent and accurate tracking of objects even when they move, change appearance, or become temporarily occluded—challenges that were difficult for previous models to overcome. As a result, SAM 2 has shown superior results across numerous video segmentation benchmarks, including DAVIS and YouTube-VOS, outperforming its predecessor by a significant margin.
Moreover, Meta SAM 2 incorporates an “occlusion head” to handle scenarios where objects may be temporarily hidden or move out of view, further refining its video segmentation accuracy. This is particularly useful in dynamic scenes where objects frequently move or are obscured by other elements. Additionally, SAM 2’s ability to resolve ambiguities by generating multiple possible masks for uncertain prompts is another feature that distinguishes it from earlier models. This capability is crucial for ensuring that the model can handle complex tasks with minimal human intervention, making it approximately three times more efficient in interactive video segmentation than SAM 1.
In terms of real-time performance, Meta SAM 2 operates at a remarkable 44 frames per second, making it highly efficient for applications that require fast processing times, such as real-time video editing and live surveillance systems. This speed is combined with improved accuracy, making SAM 2 six times faster than its predecessor in zero-shot image segmentation benchmarks. These enhancements ensure that Meta SAM 2 not only provides faster results but also maintains high-quality segmentation across a broader range of tasks.
Another critical improvement is SAM 2’s open-source nature, which continues Meta’s commitment to collaborative AI development. The model, along with its code and datasets, is available under permissive licenses, allowing researchers and developers to explore and expand on its capabilities. This openness encourages further innovation and the development of new applications, ensuring that SAM 2 can be adapted for a wide variety of uses beyond those initially envisioned by its creators.
Overall, Meta SAM 2’s advancements in memory handling, real-time processing, and open-source accessibility mark it as a significant upgrade from SAM 1, poised to set new standards in both image and video segmentation tasks. These improvements, coupled with its real-world performance, make Meta SAM 2 not just a successor, but a leap forward in the field of computer vision and AI (according to Hyperstack).
How was Meta SAM 2 Built?
Meta SAM 2, or Segment Anything Model 2, represents a significant leap forward in AI-driven visual segmentation, extending the capabilities of its predecessor, SAM, to both images and videos. The development of Meta SAM 2 involved a meticulous process that combined cutting-edge AI and machine learning development, advanced data engines, and a massive dataset tailored for video segmentation.
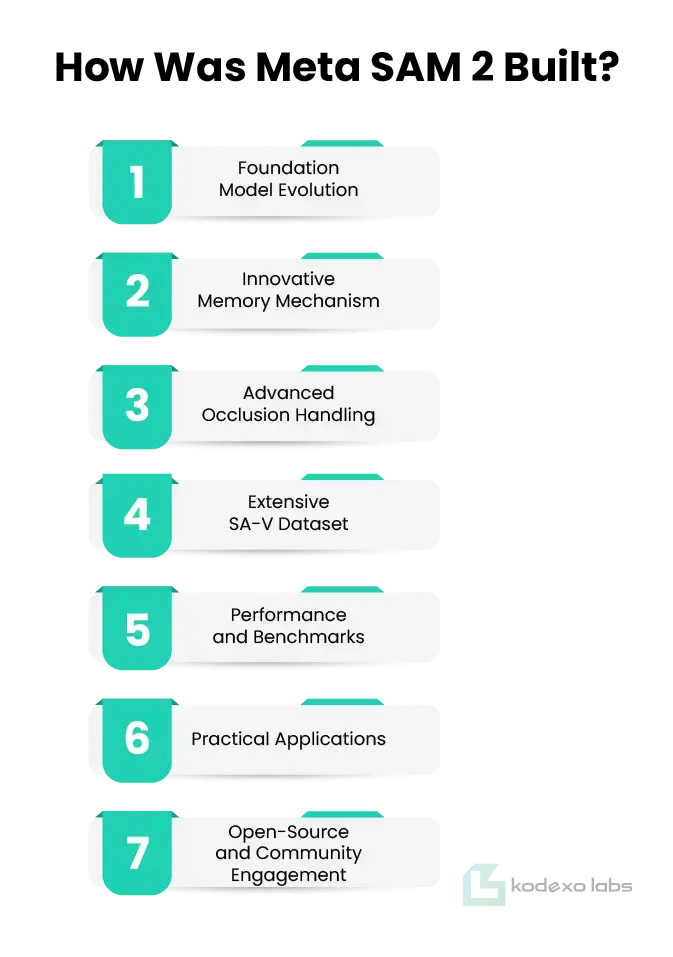
1- Foundation Model Evolution:
Meta SAM 2 builds on the success of the original SAM model by introducing a unified architecture that can handle both images and videos. The model was designed with a transformer-based architecture optimized for real-time processing, making it capable of handling video frames sequentially while storing and recalling information across frames.
2- Innovative Memory Mechanism:
One of the standout features of Meta SAM 2 is its sophisticated memory mechanism. This includes a memory encoder, memory bank, and memory attention modules, all of which work together to track and segment objects consistently across video sequences. This memory system is essential for ensuring that objects remain accurately segmented even as they move across frames or become temporarily occluded.
3- Advanced Occlusion Handling:
Meta SAM 2 introduces an “occlusion head,” a critical innovation for handling scenarios where objects temporarily disappear from view. This feature predicts whether the object of interest is present in each frame, allowing the model to maintain segmentation accuracy even in challenging video environments.
4- Extensive SA-V Dataset:
The development of Meta SAM 2 was heavily supported by the creation of the Segment Anything Video (SA-V) dataset. This dataset, which is significantly larger and more diverse than previous video segmentation datasets, was collected using an iterative data engine. The dataset includes over 600,000 mask annotations across approximately 51,000 videos, featuring diverse and complex real-world scenarios from 47 countries.
5- Performance and Benchmarks:
Meta SAM 2 outperforms its predecessor and other state-of-the-art models across various benchmarks. For instance, it achieves superior accuracy in video object segmentation (VOS) tasks, demonstrating its robustness in real-world applications. It is also significantly faster, capable of processing at 30 frames per second (FPS) on an A100 GPU, making it a powerful tool for real-time applications.
6- Practical Applications:
Meta SAM 2’s advancements open up a range of practical applications. These include object tracking for autonomous vehicles, video editing and effects, augmented reality, and even medical imaging. The model’s ability to segment and track objects in real-time makes it a versatile tool across different industries.
7- Open-Source and Community Engagement:
Consistent with Meta’s commitment to open science, SAM 2 has been released as an open-source project. This release includes the SAM 2 code, model weights, and the SA-V dataset under permissive licenses, encouraging further research and development in the field.
Meta SAM 2 represents a monumental step in AI-based image and video segmentation, with its design and implementation reflecting Meta’s ongoing efforts to push the boundaries of what is possible with Machine Learning Development. As an open-source tool, it is poised to have a broad impact, allowing researchers and developers around the world to explore and expand upon its capabilities.
Meta SAM 2 vs Previous SOTA Models:
To effectively compare Meta SAM 2 against previous state-of-the-art (SOTA) models, it’s essential to analyze various aspects where SAM 2 either excels or offers unique advancements. Here’s a detailed comparison table that highlights these points:
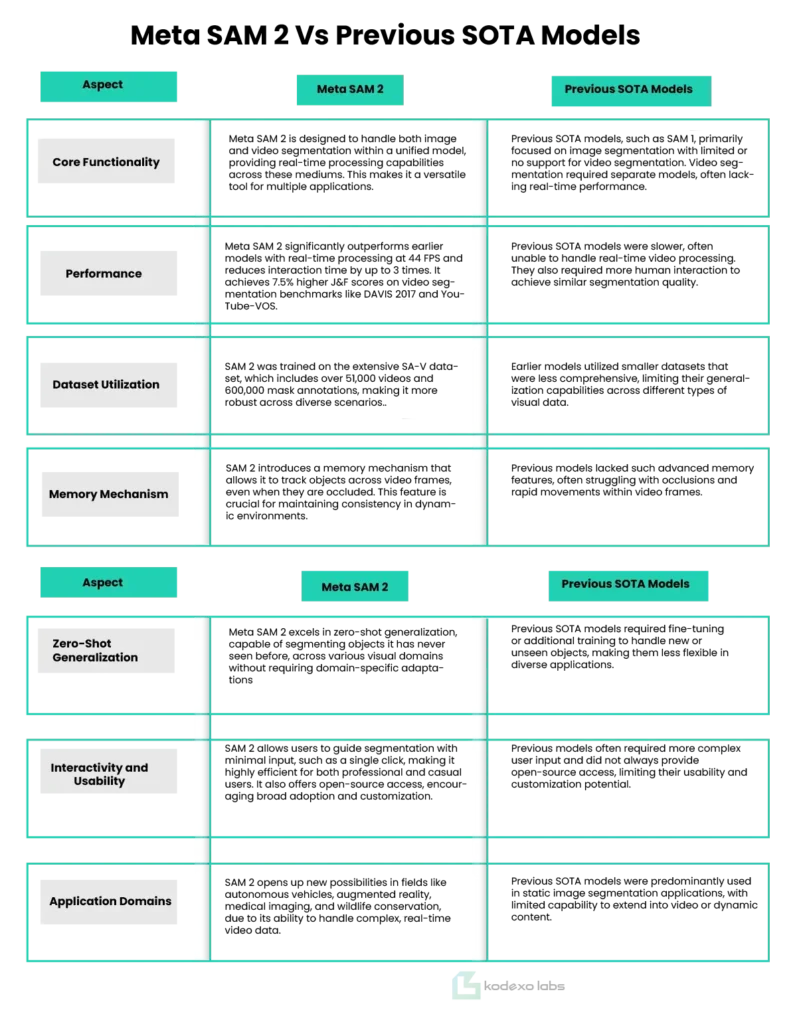
Meta SAM 2 represents a substantial leap forward in segmentation technology, particularly in its ability to handle both image and video content within a single framework. By overcoming limitations such as slow processing speeds, high interaction requirements, and restricted generalization capabilities, SAM 2 sets a new benchmark for future segmentation models (According to CTOL).
What are the Limitations to Meta SAM 2?
Meta SAM 2, the latest iteration of Meta’s Segment Anything Model, represents a significant advancement in AI-driven object segmentation across both images and videos. However, despite its groundbreaking capabilities, there are several limitations to SAM 2 that users and developers should be aware of.
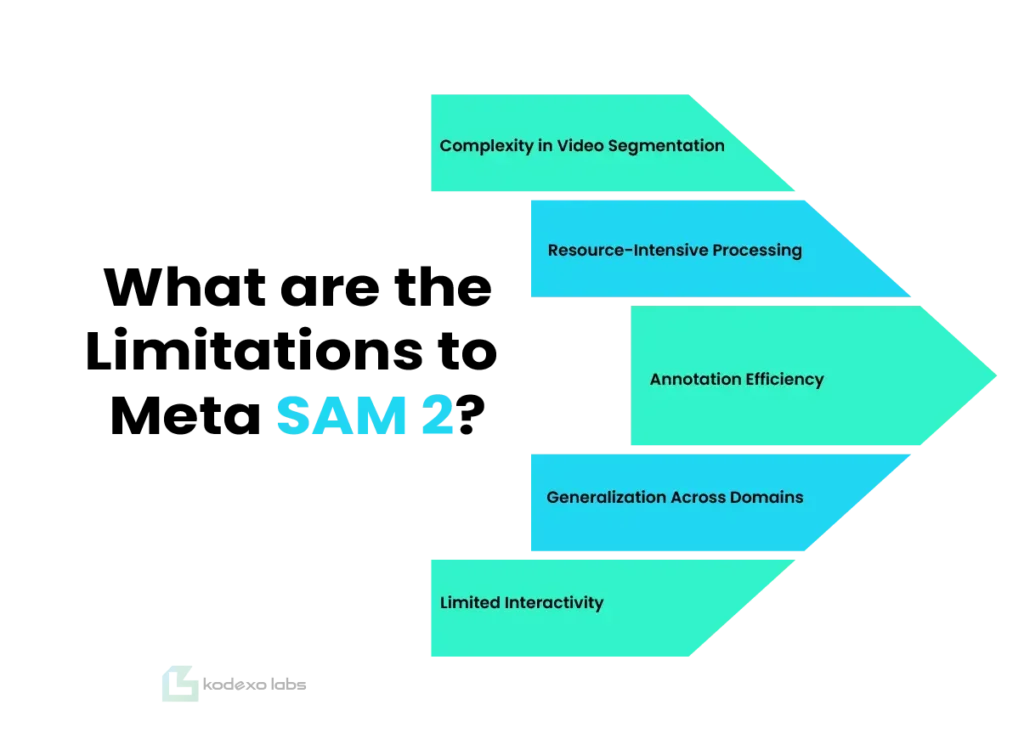
1- Complexity in Video Segmentation:
While SAM 2 excels in segmenting objects in videos, it still faces challenges when dealing with highly dynamic scenes where objects move rapidly, change appearance, or become occluded. Although SAM 2 incorporates an innovative memory mechanism to track objects across frames, handling severe occlusions or complex motions remains a difficult task. This limitation can impact the accuracy of segmentation in fast-paced or cluttered video content.
2- Resource-Intensive Processing:
The advanced architecture of SAM 2, which includes components like the memory bank and attention modules, demands substantial computational resources. This can be a bottleneck for real-time applications or in environments where processing power is limited. High-resolution content, in particular, may require significant processing time, which could limit the model’s practical application in scenarios requiring immediate results.
3- Annotation Efficiency:
Despite SAM 2’s improvements in annotation speed, which is up to 8.4 times faster than its predecessor, the process can still be time-consuming for large-scale video projects. The initial setup and fine-tuning, especially in scenarios where precise manual refinement is required, can become labor-intensive, particularly for complex scenes with multiple overlapping objects.
4- Generalization Across Domains:
Although SAM 2 is trained on a vast and diverse dataset (SA-V) with over 600,000 masklets, its performance might not generalize equally well across all domains or specific niche applications. The zero-shot generalization capability, while impressive, may still require additional adaptation or fine-tuning for highly specialized tasks or uncommon objects not well represented in the training data.
5- Limited Interactivity:
While SAM 2 supports various prompt types and interactive segmentation, the level of interactivity may not always meet the needs of all users. For example, in scenarios requiring real-time adjustments or in highly interactive environments like gaming or live broadcasting, the current capabilities might fall short, necessitating further improvements in responsiveness and adaptability.
SAM 2 is a powerful tool that pushes the boundaries of image and video segmentation. However, users should be mindful of its limitations, especially regarding its handling of complex video scenes, computational demands, and potential constraints in certain specialized applications. As Meta continues to refine and develop this model, addressing these limitations will be key to unlocking its full potential across diverse fields
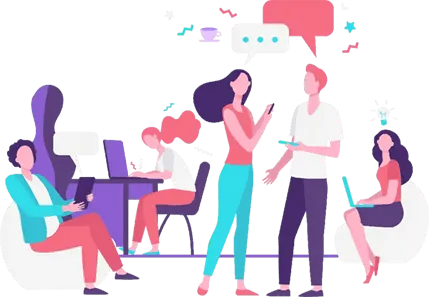
How to Put Meta SAM 2 to Work Out Magic Through Integration?
Meta SAM 2 is revolutionizing the world of AI-driven segmentation, providing advanced tools to integrate and enhance your digital workflows. Whether you’re working on video segmentation, image processing, or complex AI models, SAM 2 is designed to deliver unparalleled accuracy and efficiency. In this guide, we will explore seven easy steps to put SAM 2 to work, making sure you harness its full potential for your projects.
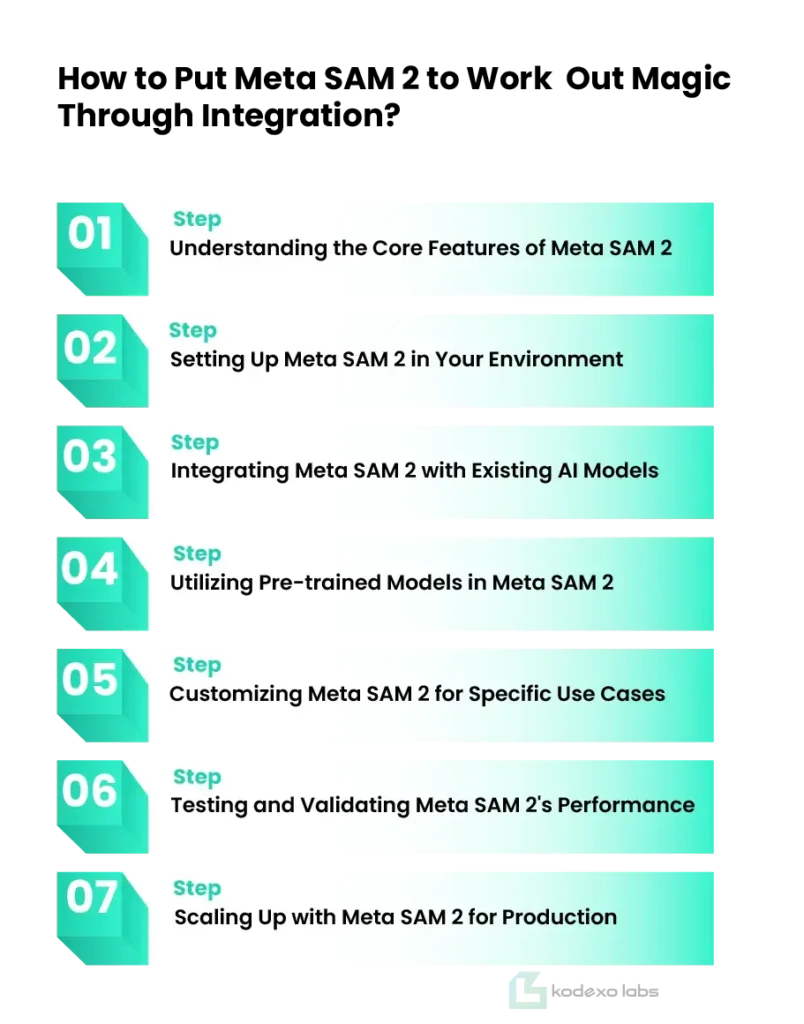
Step 1: Understanding the Core Features of Meta SAM 2
Before diving into integration, it’s essential to grasp the core features that make SAM 2 a standout tool. SAM 2 builds on the foundation of its predecessor with enhanced capabilities in segmentation accuracy, processing speed, and adaptability across various domains such as video and images. By leveraging these features, users can achieve more precise and efficient outcomes, streamlining their AI projects.
Step 2: Setting Up Meta SAM 2 in Your Environment
The first practical step involves setting up SAM 2 in your development environment. Whether you’re using Python, MATLAB, or other platforms, Meta SAM 2 offers comprehensive documentation and support to get you started. Make sure your system meets the necessary requirements to optimize performance, as SAM 2’s advanced algorithms demand robust computing power.
Step 3: Integrating Meta SAM 2 with Existing AI Models
Integration is key to unlocking the magic of SAM 2. By seamlessly incorporating Meta SAM 2 into your existing AI models, you can enhance their segmentation capabilities without starting from scratch. This step is particularly crucial for those working with large datasets or complex video sequences, where SAM 2’s efficiency shines.
Step 4: Utilizing Pre-trained Models in Meta SAM 2
One of the standout features of Meta SAM 2 is its access to a library of pre-trained models. These models, trained on vast datasets, allow you to apply advanced segmentation techniques right out of the box. Leveraging these pre-trained models can save time and resources, providing high-quality results with minimal effort.
Step 5: Customizing Meta SAM 2 for Specific Use Cases
Meta SAM 2 is highly adaptable, allowing for customization to meet the specific needs of your project. Whether you’re working on medical imaging, autonomous vehicles, or content creation, tweaking SAM 2’s parameters will enable you to fine-tune its performance to your unique requirements.
Step 6: Testing and Validating Meta SAM 2’s Performance
Testing is a critical part of the integration process. By rigorously testing Meta SAM 2 within your environment, you can validate its performance, ensuring it meets the desired standards. Utilize a variety of datasets and scenarios to comprehensively evaluate Meta SAM 2’s capabilities, identifying any areas for further optimization.
Step 7: Scaling Up with Meta SAM 2 for Production
Once tested and validated, the final step is to scale Meta SAM 2 for production. This involves optimizing resource allocation, managing data flows, and ensuring that Meta SAM 2 can handle the demands of large-scale operations. With proper scaling, Meta SAM 2 can be a cornerstone of your AI infrastructure, delivering consistent and reliable results.
By following these seven easy steps, you can fully integrate Meta SAM 2 into your AI workflows, unlocking its potential to perform magic across various applications. Whether you are a seasoned AI professional or a newcomer to the field, SAM 2 offers the tools and support to take your projects to the next level. Start leveraging the power of Meta SAM 2 today and see the difference it can make in your work.
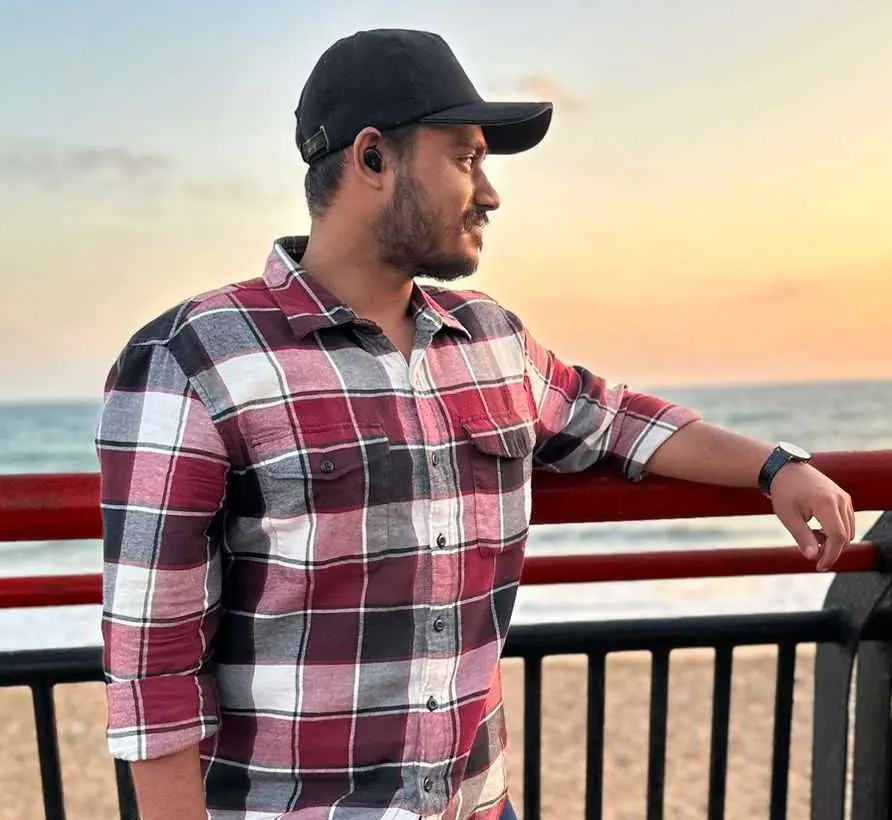