Decision Intelligence – Know All About Decision Intelligence and Why You Need It!
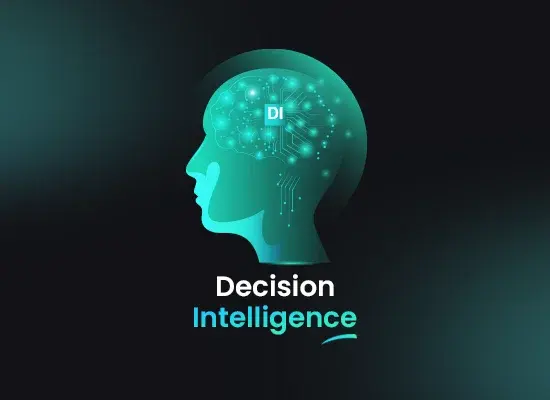
Contents
Decision intelligence is reshaping how companies approach decision-making, moving beyond instinct and limited data insights to strategic, data-driven methods. This approach integrates complex data analysis, artificial intelligence (AI), and human insights, helping businesses not only streamline decisions but also capture emerging opportunities that could otherwise be missed. In today’s fast-paced landscape, organizations that fail to adapt decision intelligence may struggle to remain competitive, as they lack the nuanced insights that drive smarter strategies and innovative growth.
Key Takeaways:
- Embrace decision intelligence by integrating data analysis and human insights to enhance your decision-making processes.
- Recognize the importance of decision intelligence in improving business outcomes, as it helps organizations make informed choices based on accurate data.
- Focus on key components such as data quality, analytics, and collaboration to build a solid foundation for effective decision intelligence.
- Implement decision intelligence tools and technologies to streamline operations and foster a data-driven culture within your organization.
- Learn from real-world success stories to understand how other businesses have effectively utilized decision intelligence for growth and efficiency.
- Invest in building decision intelligence skills within your team to ensure everyone can contribute to smarter, more effective decision-making.
- Embrace decision intelligence as a modern approach that combines data analysis and human judgment to improve your decision-making processes.
- Recognize the importance of decision intelligence in enhancing efficiency and effectiveness in various business operations, leading to better outcomes.
- Leverage the benefits of decision intelligence, such as improved accuracy, faster decisions, and the ability to predict outcomes, to gain a competitive edge.
- Measure the success of your decision intelligence initiatives by tracking key performance indicators (KPIs) related to decision quality and business results.
- Customize decision intelligence tools to fit your specific needs and workflows, ensuring they align with your organizational goals and culture.
- Stay aware of ethical considerations when implementing decision intelligence, ensuring transparency and fairness in the decision-making process.
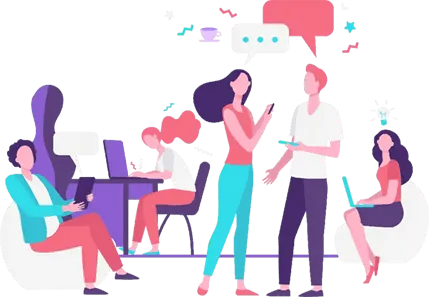
Getting Started with Decision Intelligence:
What is Decision Intelligence?
Decision intelligence (DI) is the strategic integration of data analysis, Artificial Intelligence and Machine Learning to transform decision-making into a repeatable, evidence-based process. With DI, organizations can uncover insights that were previously inaccessible, enabling them to make precise, context-aware choices. Gartner forecasts that by 2026, nearly a third of large organizations will incorporate DI frameworks to stay competitive, particularly in fast-evolving industries. By adopting DI, companies not only boost decision quality but also reduce the risks associated with guesswork or limited data insights.
When we think about the quality of our decisions, data plays a crucial role. The right information can guide us toward better outcomes. However, many firms struggle with this. A McKinsey survey from 2021 revealed that only 24% of companies identify as data-driven. Even more surprising, just 30% of employees use analytics or business intelligence tools effectively. These numbers show that we have room for improvement.
As we work towards implementing DI, understanding its components is essential. We need to recognize that today’s tools often operate in silos. Descriptive, diagnostic, and predictive analytics function separately. This separation creates gaps in insights and leads to time-consuming handoffs. By integrating these elements into a cohesive system, we can enhance our decision-making capabilities.
Why Decision Intelligence is Essential for Today’s Businesses?
Decision intelligence adds precision and agility to strategic choices, allowing organizations to make data-informed decisions that yield measurable results. For example, businesses that implement DI frameworks see improvements in innovation success rates, operational efficiency, and customer satisfaction. By empowering decision-makers with data and predictive insights, DI helps companies adapt to market changes faster, detect fraud proactively, and streamline resource allocation, ultimately providing a solid foundation for sustainable growth.
Organizations that embrace DI have a competitive edge. Gartner predicts that by 2026, nearly one-third of large organizations will integrate decision intelligence into their workflows. Those using reliable AI can achieve over a 75% success rate in innovative projects. In contrast, those without DI see only a 40% success rate. This stark difference shows the value DI brings to the table.
We should also consider the implications on fraud prevention and risk management. For example, the UK government plans to invest £25 million to combat fraudsters stealing taxpayer money. Leveraging DI can help organizations detect and prevent fraud more effectively. By analyzing patterns and anomalies in data, we can make informed decisions that protect resources.
A Brief History of Decision Making and How DI Fits In:
The evolution from intuition-based decisions to data-informed strategies marks a major shift in business. Initially, companies relied on instinct and limited data, which often restricted their ability to forecast or adapt quickly. With the advent of basic analytics, organizations began to incorporate data into decision-making, but siloed systems and limited integration meant insights were often incomplete.
Decision intelligence represents the next leap, consolidating data streams and analytics methods (descriptive, diagnostic, and predictive) into a unified, actionable system. This integration is a game-changer, allowing companies to respond to insights faster and make holistic, well-supported decisions.
The Core Components of Decision Intelligence:
Data: The Backbone of Effective Decision Making
Reliable data is the cornerstone of every informed decision. In decision intelligence, data must not only be accurate but also relevant and timely. High-quality data—sourced from customer feedback, social media analytics, market trends, and sales performance—allows organizations to understand patterns and forecast outcomes with precision. However, raw data alone isn’t enough. DI frameworks emphasize structured and unstructured data integration, transforming information into actionable insights through rigorous data quality checks and sophisticated analysis.
In 2021, businesses generated approximately 79 zettabytes of data. That’s a staggering amount! This shows how much information is available to us. We must learn to harness this wealth of data effectively.
Understanding the types of data is essential. We can categorize them into structured and unstructured data. Structured data fits neatly into tables, like numbers in a spreadsheet. Unstructured data includes text and images, which are more challenging to analyze. Both types play a vital role in shaping our decisions.
Analyzing Data: Turning Numbers into Knowledge through Decision Intelligence
Data analysis is the critical step that transforms raw data into valuable insights that drive decision-making. By utilizing advanced analytical tools—ranging from statistical software to dynamic visualization platforms—organizations can uncover trends, correlations, and anomalies that inform strategy. For example, visualizing customer feedback through graphs can highlight satisfaction trends that guide product improvements. Effective data analysis also requires a culture of inquiry: decision-makers must critically evaluate not only what the data reveals but also its implications for their business objectives.
The Role of Artificial Intelligence in Decision Making:
Artificial intelligence (AI) is a transformative force in decision intelligence, enabling organizations to process vast datasets with unprecedented speed and accuracy. AI algorithms can identify complex patterns and trends that may be overlooked in manual analyses, offering predictive insights that drive proactive decision-making.
For instance, AI-driven forecasting models can help businesses predict customer behavior, optimize inventory levels, and enhance marketing strategies based on real-time data. It is crucial, however, to remember that while AI enhances decision-making, human judgment remains vital; the best outcomes emerge when AI-generated insights are combined with contextual knowledge from experienced decision-makers.
The Essential Balance of Human Insight and Technology in Decision Intelligence:
In the realm of decision intelligence, the synergy between human insight and technological capability is essential. While data and AI provide critical information, human intuition, experience, and ethical considerations add depth to decision-making processes.
For example, a data-driven recommendation might suggest a cost-cutting measure based purely on numbers; however, a human perspective might factor in employee morale and long-term sustainability. This balance ensures that decisions are not only informed by data but are also aligned with organizational values and goals. Fostering a culture that values both analytical rigor and human judgment leads to well-rounded, strategic choices.
Moreover, collaboration among team members enhances our ability to make sound choices. Different perspectives lead to richer discussions and better solutions. We should always encourage open dialogue within our teams.
- Data Collection: Gather relevant data from various sources to inform decision-making.
- Data Integration: Combine data from different platforms and formats for a unified view.
- Analytics: Utilize statistical methods and algorithms to extract insights from data.
- Modeling: Create predictive models to simulate potential outcomes of decisions.
- Automation: Implement automated systems to streamline decision-making processes.
- Human Expertise: Incorporate expert knowledge and intuition alongside data-driven insights.
- Feedback Loops: Establish mechanisms to learn from past decisions and improve future processes.
- Visualization Tools: Use dashboards and visual aids to present data clearly for stakeholders.
- Collaboration: Foster teamwork among stakeholders to enhance decision quality and buy-in.
- Ethical Considerations: Address ethical implications in the decision-making process.
Understanding the Decision-Making Process: A Step-by-Step Guide
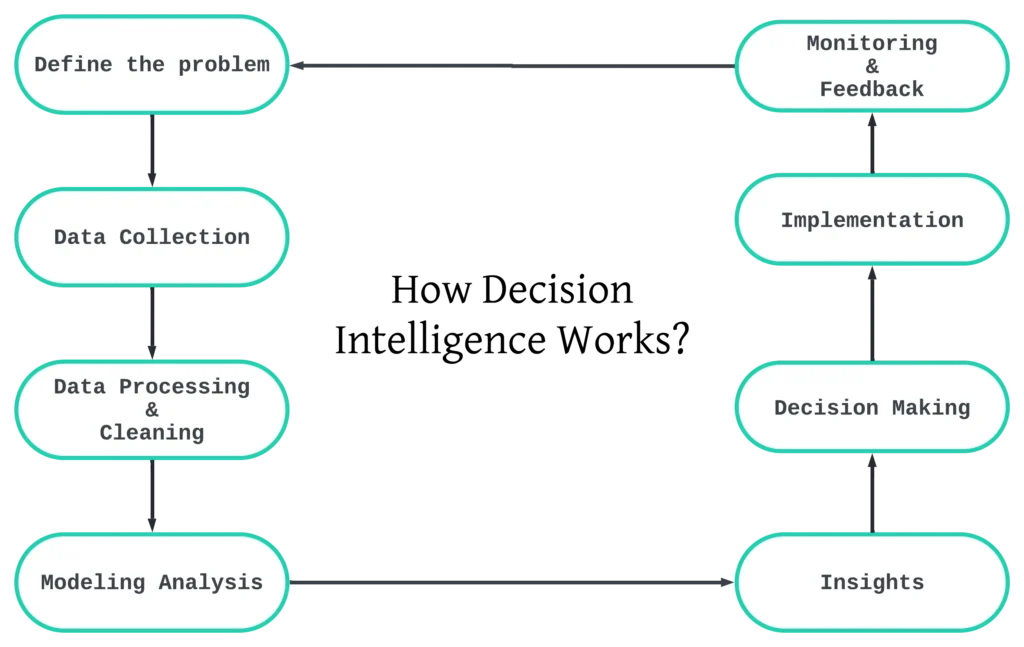
Step-by-Step: Implementing Decision Intelligence
Implementing decision intelligence involves a structured approach to the decision-making process. The first step is clearly defining the problem or opportunity at hand; without this clarity, subsequent decisions may lack direction. Following this, organizations gather relevant data from various sources, analyze this information to extract meaningful insights, and brainstorm potential solutions.
Creativity is vital in this phase, allowing for innovative options to surface. Each option is then evaluated based on its alignment with organizational goals, potential risks, and expected outcomes. Finally, decisions are made and actions taken, with ongoing monitoring of results to inform future decision-making. This systematic approach ensures that decision intelligence is applied consistently, maximizing its impact.
Tools You Need to Get Started with Decision Intelligence:
Successful implementation of decision intelligence relies on the right tools, which facilitate data management and streamline decision-making processes. Investing in effective software solutions can enhance the overall impact of decision intelligence initiatives.
Another useful tool is predictive analytics software. It helps us forecast future trends based on historical data. By using these predictions, we can make informed decisions that are more likely to succeed.
Collaboration platforms also play a vital role in our decision-making journey. They allow us to share insights and ideas with team members easily. Effective communication enhances our collective understanding of the problem at hand.
Lastly, machine learning algorithms can improve our analysis capabilities. These algorithms learn from past data and refine their predictions over time. By integrating them into our workflow, we enhance our decision-making efficiency.
From Data to Decisions: Making It Happen
Turning data into actionable decisions requires careful execution. We start by ensuring that our data is clean and accurate. Poor quality data can lead to faulty conclusions. Therefore, regular audits of our data sources are necessary.
Once we have reliable data, we apply analytical techniques to extract meaningful insights. For instance, using statistical methods can reveal significant correlations in the data. Understanding these relationships guides us toward better decisions.
Next, we create a structured framework for evaluating options. This framework should align with our organizational objectives. By doing so, we ensure that every decision contributes to our overall mission.
Aspect | Description |
---|---|
Definition | Decision Intelligence (DI) is a framework that combines data science, social science, and managerial science to improve decision-making. |
Components | – Data Collection – Data Analysis – Predictive Modeling – Decision Support Systems – Feedback Loops |
Processes | 1. Data Gathering 2. Data Cleaning 3. Data Analysis 4. Model Development 5. Decision Execution 6. Performance Monitoring |
Techniques Used | – Machine Learning – Statistical Analysis – Simulation Models – Optimization Algorithms |
Tools and Technologies | – Business Intelligence Software – Data Visualization Tools – Predictive Analytics Platforms |
Benefits | – Enhanced Decision Quality – Increased Speed of Decision-Making – Improved Operational Efficiency |
Challenges | – Data Privacy Concerns – Integration with Existing Systems – Resistance to Change from Stakeholders |
Applications | – Financial Services – Healthcare – Supply Chain Management – Marketing Strategies |
Future Trends | – Increased Automation in Decision Processes – Greater Use of AI and Machine Learning – Focus on Ethical Decision-Making |
After assessing options through our framework, we choose the best course of action. Implementing this choice requires teamwork and coordination among all involved parties. Communication remains key during this phase.
Finally, we must reflect on the outcomes of our decisions. Analyzing what worked and what didn’t, informs future choices. Continuous improvement is integral to successful decision intelligence.
The Benefits You Can Expect:
Boosting Business Performance with Decision Intelligence:
We often find ourselves looking for ways to improve our business decisions. Implementing decision intelligence can significantly enhance productivity and decision quality. Organizations leveraging DI often report improvements in operational efficiency and resource allocation, leading to better overall performance.
In 2020, a study showed that businesses using decision intelligence improved their performance by up to 20%. This means more profits and happier teams. We can make smarter choices about resource allocation and project management. By understanding what works best, we save time and money.
Implementing decision intelligence tools allows us to visualize data clearly. When we see trends and patterns, we can act quickly. This agility keeps us ahead of competitors. We can also tailor our strategies based on real-time insights. In turn, this builds trust with our clients and partners.
Making Better Personal Choices using Decision Intelligence:
We all face decisions daily, both big and small. Decision intelligence can guide us in making better personal choices. It helps us weigh options based on facts rather than emotions. For instance, when choosing a college or career path, we can analyze various factors like job market trends and salary expectations.
According to Gartner, by 2023, more than 33% of large organizations will have analysts practicing decision intelligence, including decision modelling. We can apply similar principles to our health and lifestyle choices as well. By analyzing our habits and preferences, we can create personalized plans that lead to healthier living.
Moreover, decision intelligence encourages us to reflect on past choices. By understanding what worked and what didn’t, we learn valuable lessons. This self-awareness shapes our future decisions positively. We become more confident in the choices we make every day.
Minimizing Risks and Uncertainties using Decision Intelligence:
Every decision carries some level of risk. We often worry about making the wrong choice. Decision intelligence helps us minimize these risks by providing clear data analysis. It allows us to assess potential outcomes before taking action.
For example, businesses using decision intelligence tools can predict market changes more accurately. A report states that in 2021, companies that adopted these tools reported a 25% reduction in financial losses due to poor decisions. We can avoid costly mistakes by understanding trends and customer behavior thoroughly.
Decision intelligence empowers us to prepare for uncertainties. By analyzing various scenarios, we can develop contingency plans. This proactive approach reduces stress and enhances our confidence in navigating challenges.
In our personal lives, we can apply similar strategies to manage uncertainties effectively. Whether planning a vacation or investing in stocks, having reliable data makes a difference. We feel more secure knowing we’ve examined all possible angles before deciding.
Benefit | Description | Impact on Decision-Making | Example Use Case | Key Takeaway |
---|---|---|---|---|
Improved Accuracy | Enhances precision in predictions and outcomes | Reduces errors in decision-making | Financial forecasting | More reliable data leads to better choices |
Enhanced Speed | Accelerates data analysis and insights generation | Enables quicker responses | Real-time market analysis | Faster decisions can capitalize on opportunities |
Better Risk Management | Identifies potential risks through predictive models | Minimizes negative consequences | Fraud detection in transactions | Proactive risk identification saves resources |
Increased Collaboration | Facilitates shared insights across teams | Promotes unified decision-making | Cross-departmental strategy planning | Collaborative efforts lead to comprehensive strategies |
Continuous Learning | Adapts and improves over time with new data | Evolves decision-making processes | Customer behavior analysis | Ongoing improvement enhances future decisions |
Where Can You Use Decision Intelligence?
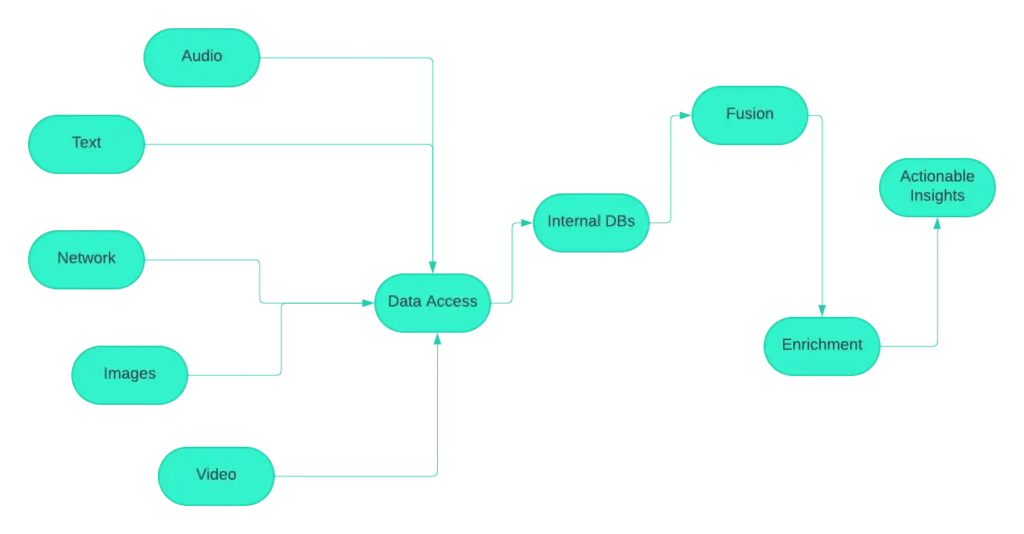
Decision Intelligence in the Business World: From Startups to Giants
We see decision intelligence playing a vital role in businesses of all sizes. Startups benefit from quick, data-driven choices. They often face tight budgets and fierce competition. Using decision intelligence tools helps them identify opportunities faster than their rivals.
Larger companies also use decision intelligence for strategic planning. They analyze vast amounts of data to make informed decisions. Companies like Amazon and Google rely on these insights to enhance customer experiences. This technology allows them to predict trends and adjust strategies accordingly.
In our experience, decision intelligence creates a more agile business environment. We can respond to market changes quickly. It reduces risks associated with poor decisions. By leveraging data, we enhance our chances of success.
Decision Intelligence in Healthcare: Making Critical Medical Decisions
Decision intelligence is crucial in healthcare settings. Doctors and medical teams rely on accurate data to make life-saving decisions. Algorithms can analyze patient records and suggest treatment options. This leads to better outcomes for patients.
For example, hospitals use decision intelligence systems to manage patient flow. These systems predict which patients need immediate care. This ensures that resources are allocated efficiently. We have seen how this technology improves hospital operations.
Moreover, decision intelligence supports research in medicine. By analyzing clinical trial data, researchers identify effective treatments faster. This accelerates the development of new drugs and therapies. We recognize that these advancements save lives and improve health outcomes.
Decision Intelligence in Finance: Smarter Investments and Risk Management
In finance, decision intelligence transforms how we manage investments. We can evaluate various investment options using data analytics tools. These tools provide insights into market trends and economic indicators.
Risk management is another area where decision intelligence shines. Financial institutions assess potential risks before making decisions. We see this in banks that use algorithms to detect fraud patterns. This proactive approach protects both the institution and its customers.
Investment firms also leverage decision intelligence for portfolio management. They analyze performance data to optimize asset allocation. This leads to smarter investment strategies that maximize returns while minimizing risks.
Decision Intelligence in Everyday Life: Simplifying Daily Choices
Decision intelligence doesn’t just belong in business or healthcare; it impacts our daily lives too. We encounter countless choices every day, from what to eat to where to shop. Decision intelligence helps us make better selections based on our preferences and needs.
For instance, apps use algorithms to recommend restaurants based on our taste profiles. These suggestions save us time and enhance our dining experiences. Similarly, shopping platforms track our preferences to provide personalized deals.
Even in planning events, decision intelligence plays a role. We can analyze guest preferences and budget constraints easily. This ensures that we create memorable experiences without overspending.
- Enhanced Decision-Making: Improves the quality of decisions by integrating data analysis and human intuition.
- Predictive Analytics: Utilizes historical data to forecast future trends and outcomes, aiding proactive decision-making.
- Risk Management: Identifies potential risks and evaluates their impacts to mitigate adverse effects on business operations.
- Operational Efficiency: Streamlines processes by optimizing resource allocation based on data-driven insights.
- Personalization: Tailors customer experiences by analyzing preferences and behaviors for targeted marketing strategies.
- Scenario Planning: Facilitates exploration of various outcomes to prepare for different future scenarios effectively.
- Collaboration: Enhances teamwork by providing a shared understanding of data and insights across departments.
- Continuous Improvement: Supports iterative learning from past decisions to refine strategies and improve future outcomes.
- Real-Time Insights: Offers immediate access to data analytics, enabling quick responses to changing conditions.
- Integration with AI: Combines human judgment with artificial intelligence for more robust decision frameworks.
Implementing Decision Intelligence in Your Organization:
Getting Started: First Steps to Take
A clear implementation plan is essential for decision intelligence. Start by identifying specific goals to achieve through DI, which will guide your strategy and help align team efforts. What do we want to achieve? This could be improving customer satisfaction or increasing sales. Setting specific objectives guides our efforts.
Next, we should gather our data. Data is crucial for decision intelligence. We can use data from various sources like customer feedback, sales reports, and market trends. This information helps us understand our current situation better.
Then, we need to involve our team. Everyone should understand the importance of decision intelligence. We can hold workshops or meetings to explain how it works. Engaging everyone creates a sense of ownership. This involvement encourages collaboration and innovation.
Common Challenges with Decision Intelligence and How to Overcome Them:
Implementing decision intelligence comes with challenges. Resistance to change is a common challenge when implementing decision intelligence. To overcome this, provide comprehensive training and demonstrate the tangible benefits of DI, fostering a culture of innovation and openness among team members. To overcome this, we can provide training sessions. These sessions can help our team feel more confident about using new tools.
Another challenge is data quality. Poor quality data leads to incorrect decisions. We should regularly check our data for accuracy and relevance. Establishing strict data management practices will help us maintain high standards.
Lastly, we might face integration issues with existing systems. Our current systems may not easily connect with new decision intelligence tools. To address this, we can work closely with IT specialists. They can help ensure smooth integration without disrupting our operations.
Best Practices for a Smooth Implementation using Decision Intelligence:
Following best practices makes implementation easier for us. First, we should start small. Focusing on one area allows us to test the waters before expanding further. For example, we might begin with customer service before moving on to marketing strategies.
Regularly reviewing progress is essential too. We need to check if our objectives are being met and adjust as necessary. Setting up monthly meetings helps keep everyone informed and engaged.
Lastly, we must celebrate successes along the way. Recognizing achievements boosts morale and encourages continued effort. Sharing positive outcomes reinforces the value of decision intelligence in our organization.
Pros | Cons | Considerations |
---|---|---|
Enhances decision-making speed and accuracy | High initial implementation costs | Assess budget and ROI before implementation |
Integrates data from multiple sources | Requires ongoing maintenance and updates | Plan for continuous support and training |
Improves predictive analytics capabilities | Potential resistance from staff | Engage stakeholders early in the process |
Facilitates data-driven culture | Complexity of technology integration | Ensure compatibility with existing systems |
Increases operational efficiency | Risk of over-reliance on technology | Balance human insight with AI-driven decisions |
Supports real-time decision-making | Data privacy and security concerns | Implement robust data governance policies |
Enables better resource allocation | May require significant training for employees | Develop a comprehensive training program |
Provides insights for strategic planning | Limited understanding of decision intelligence | Educate teams on the benefits and functionalities |
The Future of Decision Intelligence:
What’s Next? Emerging Trends to Watch:
We see several exciting trends shaping the future of decision intelligence. One major trend is the increasing integration of machine learning using Machine Learning Development. This technology helps us analyze large data sets quickly. It allows us to make better choices based on real-time information.
Another trend is the rise of automated decision-making systems. These systems can handle routine tasks without human intervention. They improve efficiency and reduce errors. With automation, we can focus on more complex decisions that require human insight.
We notice a shift towards more collaborative decision-making tools. These tools allow teams to work together seamlessly, regardless of location. We can share data and insights in real time. This fosters teamwork and leads to better outcomes.
The Growing Influence of AI in Decision Making:
Artificial intelligence (AI) plays a crucial role in decision intelligence. We use AI to uncover patterns and trends in our data. This capability enhances our understanding of various situations. It also empowers us to make informed choices faster.
AI-driven analytics tools are becoming more accessible for organizations of all sizes. We can leverage these tools to gain insights that were once difficult to obtain. For example, predictive analytics helps us forecast future trends based on historical data. This gives us a competitive edge.
Moreover, AI development improves personalized decision-making experiences. It tailors recommendations based on individual preferences. By analyzing user behavior, we can provide customized solutions that meet specific needs.
Ethics and Responsibility: Doing It Right with Decision Intelligence
As we embrace decision intelligence, we must consider ethics and responsibility. We face challenges related to data privacy and security. Protecting sensitive information is essential as we collect and analyze vast amounts of data.
Transparency is another critical factor in ethical decision-making. We should ensure that our processes are clear and understandable. This builds trust among our stakeholders and users.
Lastly, we need to address biases in our decision-making models. If left unchecked, biases can lead to unfair outcomes. We must actively work to identify and eliminate these biases from our algorithms.
- Enhanced Data Integration: Improved capabilities to integrate diverse data sources for more comprehensive insights.
- Real-Time Decision Making: Faster processing of information leading to immediate decision-making support.
- Predictive Analytics: Increased reliance on predictive models to anticipate outcomes and trends.
- User-Friendly Interfaces: Development of intuitive tools that simplify complex decision-making processes for non-experts.
- Ethical AI Considerations: Greater emphasis on ethical frameworks to guide AI-driven decisions and mitigate biases.
- Collaboration Tools: Enhanced platforms for teamwork, allowing multiple stakeholders to contribute to decision-making processes.
- Continuous Learning: Systems that adapt and improve over time based on feedback and new data inputs.
- Scenario Planning: Advanced simulations enabling organizations to visualize potential future scenarios and their impacts.
- Automated Decision Support: More automated systems that assist or even make decisions based on predefined criteria.
- Cross-Domain Applications: Broader use of decision intelligence across various sectors such as healthcare, finance, and logistics.
Real-World Success Stories:
How Leading Companies Are Winning with Decision Intelligence?
We often hear about how major companies use decision intelligence to enhance their operations. For instance, in 2020, Walmart implemented this technology to optimize its supply chain. By analyzing data, they reduced delivery times significantly. This change helped them serve customers better during peak seasons.
Another example is Netflix. They use decision intelligence to personalize recommendations for viewers. Their system analyzes watching habits and preferences. As a result, Netflix keeps viewers engaged and increases subscription rates.
Many companies are also using decision intelligence in finance. JPMorgan Chase utilizes algorithms to detect fraud quickly. They analyze transaction patterns in real time. This method allows them to protect customers effectively.
Lessons Learned from Real-Life Applications through Decision Intelligence:
We can learn valuable lessons from these success stories. First, data-driven decisions lead to better outcomes. Companies that rely on solid data make smarter choices. They reduce risks and improve performance.
Second, collaboration among teams is crucial. When departments share insights, they create a more comprehensive view of the situation. This teamwork enhances decision-making processes and leads to innovative solutions.
Lastly, we see the importance of adapting to changes. The business environment evolves rapidly. Companies that embrace decision intelligence can adjust their strategies quickly. For instance, during the COVID-19 pandemic, many firms shifted their focus based on new consumer behaviors.
Inspiring Examples from Various Industries using Decision Intelligence:
Different industries showcase how decision intelligence can drive success. In healthcare, hospitals use it to improve patient care. For example, Mount Sinai Health System uses predictive analytics for patient admissions. They analyze historical data to forecast patient needs accurately.
In retail, Target employs decision intelligence for inventory management. They track purchasing trends and adjust stock levels accordingly. This strategy minimizes waste and ensures products are available when needed.
The manufacturing sector also benefits from decision intelligence. General Electric (GE) applies it to monitor equipment performance. By analyzing data from machines, GE predicts when maintenance is necessary. This approach reduces downtime and saves costs.
Company | Benefits of Decision Intelligence | How They Use Decision Intelligence? |
---|---|---|
Improved data-driven decision-making | Utilizes AI algorithms to analyze vast amounts of data for insights | |
IBM | Enhanced operational efficiency | Implements predictive analytics to optimize business processes |
Amazon | Personalized customer experiences | Leverages machine learning to tailor recommendations for users |
Netflix | Content recommendation and viewer engagement | Analyzes viewing patterns to suggest relevant shows and movies |
Microsoft | Streamlined project management | Uses decision intelligence tools to prioritize tasks and resources |
Procter & Gamble | Optimized supply chain management | Employs analytics to forecast demand and manage inventory levels |
Uber | Improved route optimization | Analyzes real-time traffic data to enhance ride-sharing efficiency |
Salesforce | Enhanced sales forecasting | Uses AI-driven insights to predict sales trends and customer behavior |
Siemens | Better risk management | Applies decision intelligence for predictive maintenance in manufacturing |
Walmart | Efficient inventory management | Uses data analytics to optimize stock levels and reduce waste |
Essential Tools and Resources:
Top Software and Platforms for Decision Intelligence:
We often explore various software and platforms that enhance our decision-making process. These tools help us analyze data effectively. They allow us to visualize information clearly. Some popular options include Tableau, Microsoft Power BI, and Qlik. Each offers unique features.
Tableau stands out for its strong visualization capabilities. It helps us create interactive dashboards. These dashboards make complex data easy to understand. Users can drag and drop elements to customize their views. This flexibility improves our insights.
Microsoft Power BI integrates well with other Microsoft products. We find it useful for creating reports quickly. The interface is user-friendly, which makes it accessible for everyone. We can share our findings with team members easily. This collaboration strengthens our decision-making efforts.
Qlik focuses on associative data modeling. This means we can explore data in multiple ways at once. It encourages us to ask questions and dive deeper into the information. The platform’s unique approach helps uncover hidden patterns.
Learning More: Educational Resources You Need for Decision Intelligence
We believe in continuous learning about decision intelligence. Many resources are available to deepen our understanding. Online courses, webinars, and books provide valuable information.
Coursera offers several courses on decision-making frameworks. These courses cover both theory and practical applications. They help us grasp the concepts needed to make informed choices. Participating in these courses boosts our confidence in using decision intelligence tools.
Webinars hosted by industry experts also enrich our knowledge. They discuss current trends and best practices in decision intelligence. We gain insights into real-world applications from professionals who use these tools daily.
Books like “Competing on Analytics” by Thomas H. Davenport are excellent resources too. They provide case studies that illustrate successful decision-making strategies. Reading these materials broadens our perspective on how to implement decision intelligence effectively.
Joining the Community: Networks and Forums to Explore
We find value in connecting with others interested in decision intelligence. Networking opens doors to new ideas and collaborations. Several online forums and communities focus on this topic.
LinkedIn groups dedicated to decision intelligence are great places to start. We can engage with professionals from various industries there. Sharing experiences helps us learn from one another’s successes and challenges.
Reddit has communities where people discuss decision intelligence topics too. We can ask questions, share resources, or seek advice from experienced members. This interaction fosters a supportive learning environment.
Attending conferences focused on data analytics is another way to connect. These events allow us to meet experts face-to-face. We can participate in workshops and discussions that enhance our skills further.
- Apache Spark: A unified analytics engine for large-scale data processing, ideal for machine learning tasks in decision intelligence.
- KNIME: An open-source platform for data analytics, reporting, and integration, facilitating visual programming and workflow design.
- Orange: A data visualization and analysis tool that uses a visual programming interface for machine learning and data mining.
- TensorFlow: An open-source library for machine learning that offers flexible tools, libraries, and community resources for building decision intelligence applications.
- R (and RStudio): A statistical programming language and environment widely used for data analysis and visualization in decision-making processes.
- Scikit-learn: A Python library that provides simple and efficient tools for data mining and machine learning, essential for predictive modeling.
- RapidMiner: A data science platform offering a suite of tools for data preparation, machine learning, and model deployment.
- H2O.ai: An open-source AI and Machine Learning development platform that enables users to build predictive models and perform advanced analytics with ease.
- WEKA: A collection of machine learning algorithms for data mining tasks, providing tools for data preprocessing, classification, regression, and clustering.
- Apache Airflow: A platform to programmatically author, schedule, and monitor workflows, useful in managing decision intelligence pipelines.
Building Your Decision Intelligence Skills:
Key Skills to Develop:
We need to focus on several key skills to excel in decision intelligence. First, analytical thinking is crucial. This skill helps us break down complex problems into manageable parts. We can identify patterns and trends through careful analysis. This leads to better decision-making.
Next, we must develop our data literacy. Understanding data is essential in today’s world. We should learn how to read, interpret, and visualize data effectively. This allows us to make informed decisions based on real evidence.
Finally, collaboration is important. Working with others enhances our decision-making abilities. We gain new perspectives from teammates. Sharing ideas often leads to more innovative solutions.
Training and Certifications to Consider:
We can enhance our skills through various training programs and certifications. Many organizations offer courses that focus on decision intelligence. For example, the Data Science Council of America provides certifications that cover data analysis and decision-making strategies.
Online platforms like Coursera and Udacity offer specialized courses. These courses allow us to learn at our own pace. They cover topics such as machine learning and predictive analytics.
Attending workshops and conferences also helps us grow. Networking with professionals in the field opens up new opportunities. We can share experiences and learn from others’ successes.
- Data Analysis Courses: We can take courses in data analysis to learn how to interpret data accurately. Platforms like Coursera or edX offer these courses from reputable universities.
- Decision-Making Workshops: Attending workshops focused on decision-making strategies can be beneficial. These workshops often provide hands-on experience and real-life case studies.
- Certifications in Business Analytics: Obtaining a certification in business analytics can enhance our credentials. Programs like the Certified Business Analysis Professional (CBAP) equip us with essential tools for effective decision-making.
- Emotional Intelligence Training: Participating in training sessions focusing on emotional intelligence can improve our interpersonal skills. Organizations like TalentSmart offer valuable resources and certifications.
- Leadership Development Programs: Engaging in leadership programs can help us cultivate decision-making abilities within teams. These programs often cover strategic thinking and problem-solving techniques.
Staying Updated: Keeping Up with the Latest Decision Intelligence Trends
We must stay updated with the latest trends in decision intelligence. Technology changes rapidly, so continuous learning is vital. Following industry news sources keeps us informed about new tools and methodologies.
Joining professional groups or forums is another way to stay connected. These platforms allow us to discuss challenges and share insights with peers. Engaging in conversations about recent developments helps us adapt quickly.
Participating in webinars is also beneficial. Many experts share their knowledge through these sessions. By attending regularly, we gain valuable information that can improve our decision-making processes.
- Data Analysis Skills: Ability to interpret and analyze complex datasets effectively.
- Statistical Knowledge: Understanding of statistical methods and models to derive insights.
- Machine Learning Proficiency: Familiarity with machine learning algorithms and their applications in decision-making.
- Programming Skills: Competence in programming languages such as Python or R for data manipulation and model building.
- Domain Expertise: In-depth knowledge of the specific industry or field to contextualize decisions.
- Critical Thinking: Strong analytical thinking to evaluate options and outcomes critically.
- Communication Skills: Ability to clearly present findings and recommendations to stakeholders.
- Ethical Considerations: Awareness of ethical implications in data usage and decision-making processes.
- Collaboration Abilities: Teamwork skills to work effectively with cross-functional teams.
- Adaptability: Openness to new tools, technologies, and methodologies in a rapidly evolving field.
Technical Deep Dive:
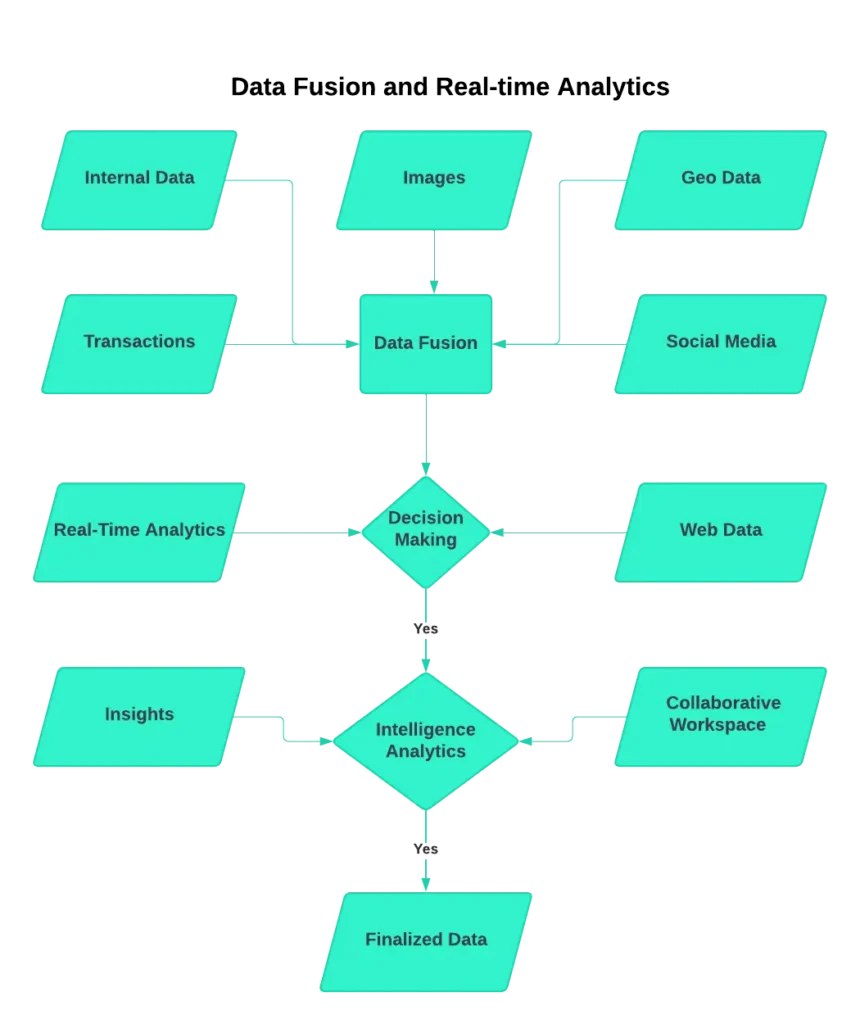
Understanding Machine Learning in Decision Intelligence:
We often wonder how machine learning fits into decision intelligence. This technology plays a crucial role in helping us make better choices. Machine learning uses algorithms to analyze data. It learns from past experiences and improves over time.
For instance, we can see this in action with recommendation systems. Netflix and Amazon use these systems to suggest movies or products based on our previous choices. They gather data about our likes and dislikes. Then, they apply machine learning to predict what we might enjoy next. This process helps businesses tailor their services to meet our needs.
Machine learning Operations (MLOps) also help us identify patterns. We can use these patterns to forecast future trends. For example, companies analyze sales data to understand customer behavior. By recognizing these trends, we can make informed decisions about inventory and marketing strategies. This approach leads to better outcomes for both businesses and customers.
Integrating Decision Intelligence with Existing Systems:
Integrating decision intelligence with our current systems is essential for success. We need to ensure that new technologies work well with what we already have. This integration can be challenging but offers significant benefits.
First, we should assess our existing infrastructure. Understanding our current systems allows us to identify gaps. We can then determine how decision intelligence can fill those gaps effectively. For example, if we have an outdated database, we may need to upgrade it before implementing new technologies.
Next, collaboration is key during the integration process. Teams from different departments should work together. This teamwork ensures everyone understands how decision intelligence will enhance operations. Regular meetings can help align goals and address concerns.
Finally, testing is vital after integration. We must evaluate how well decision intelligence performs within our systems. Pilot programs allow us to monitor results and make adjustments as needed. By doing this, we can maximize the effectiveness of our new tools.
Overcoming Common Obstacles:
Dealing with Data Quality Issues in Decision Intelligence:
We often face challenges with data quality. Poor data can lead to bad decisions. It can throw off our entire analysis. We need to ensure that the information we use is accurate and reliable.
First, we should establish clear standards for data collection. This means defining what good data looks like. For instance, we can use specific formats for dates or numbers. This helps everyone understand how to input data correctly.
Next, regular audits of our data are crucial. These checks help us spot errors early. We might find duplicates or missing values. Addressing these issues promptly keeps our analysis on track.
Finally, training our team is essential. Everyone needs to understand the importance of high-quality data. By holding workshops, we can teach best practices in data entry and management. This way, we create a culture that values accurate information.
Navigating Organizational Resistance with Decision Intelligence:
We frequently encounter resistance within organizations when implementing decision intelligence. People may fear change or feel uncertain about new processes. Understanding their concerns is the first step in overcoming this challenge.
We must communicate the benefits clearly. Sharing success stories from other organizations can help us demonstrate value. For example, a company that improved its sales by using decision intelligence techniques can inspire others to follow suit.
Involving key stakeholders early on is also vital. We should engage them in discussions about the implementation process. Their input can ease fears and build support for new initiatives. When people feel included, they are more likely to embrace change.
Lastly, providing ongoing support during the transition is crucial. We can set up help desks or regular check-ins to address questions and concerns. By being there for our colleagues, we foster a smoother transition and greater acceptance of decision intelligence.
Ensuring Data Privacy and Security with Decision Intelligence:
Data privacy and security are critical issues we cannot ignore. With increasing regulations like GDPR, protecting sensitive information is essential for our organization’s credibility.
First, we must implement strong security measures. This includes using encryption for sensitive data both at rest and in transit. Regularly updating software protects against vulnerabilities too.
Next, educating our team about data privacy is necessary. Everyone should understand their role in safeguarding information. We can host training sessions that cover topics like phishing scams and secure password practices.
Finally, we need to establish clear policies regarding data access and sharing. Limiting access to only those who need it reduces risks significantly. By creating tiered access levels, we ensure that sensitive information remains secure while still allowing necessary collaboration.
Measuring Success in Decision Intelligence:
Key Metrics to Track:
To gauge the success of decision intelligence initiatives, focus on key performance indicators (KPIs) such as decision accuracy, speed of decision-making, and user satisfaction. Regularly reviewing these metrics allows for continuous improvement in decision-making processes.
First, we should track the accuracy of decisions. This means checking how often our decisions lead to the desired outcomes. If we see a high accuracy rate, we know our system is effective.
Next, we must consider speed of decision-making. In today’s fast-paced world, quick decisions are crucial. We can measure how long it takes to reach a decision using our system. A shorter time frame indicates that our tools are efficient.
Another important metric is user satisfaction. We should gather feedback from those who use the system. Their insights will show us if the tools meet their needs. High satisfaction rates suggest that our decision intelligence approach is on the right track.
Evaluating the Impact of Decision Intelligence on Your Business:
Evaluating how decision intelligence impacts our business is essential. We must look at both financial and non-financial results. Financially, we can analyze changes in revenue or cost savings after implementing decision intelligence systems. If we notice an increase in profits, it shows that our efforts are paying off.
We also need to evaluate customer engagement. By tracking changes in customer interactions, we can see if our decisions improve their experience. For example, if we launch a new product based on data insights and customers respond positively, this reflects well on our decision-making process.
Lastly, we should assess team performance. If decision intelligence tools enhance collaboration among team members, this is a significant win. Improved teamwork often leads to better outcomes across all areas of the business.
Continuous Improvement: Keeping the Momentum Going
Continuous improvement is vital for maintaining success in decision intelligence. We need to regularly review our processes and results. This allows us to identify areas for enhancement and keep up with changing trends.
One way to ensure continuous improvement is through regular training sessions for our team. By keeping everyone updated on new tools and techniques, we can maximize our effectiveness. Training fosters a culture of learning and adaptation within our organization.
We should also encourage open communication among team members. Sharing experiences helps us learn from each other’s successes and mistakes. This collaborative environment drives innovation and keeps our decision intelligence practices relevant.
Decision Intelligence vs. Traditional Decision Making
Comparing Approaches: What’s the Difference?
We often hear about decision intelligence and traditional decision-making. Both methods help us make choices, but they differ significantly.
Traditional decision-making relies on human intuition and past experiences. We gather data, analyze it, and then make a choice based on what we know. This method can be slow and sometimes biased. Our feelings might cloud our judgment. For example, when choosing a restaurant, we might pick one based on a good experience rather than objective reviews.
In contrast, decision intelligence uses advanced technology. It combines data analysis with machine learning. This approach helps us see patterns in large sets of data. Algorithms do the heavy lifting, providing insights that we might miss. For instance, if we want to choose a vacation spot, decision intelligence can analyze trends from social media, weather data, and travel costs. This results in a more informed choice.
The key difference lies in how we process information. Traditional methods depend on our knowledge and instincts. Decision intelligence focuses on data-driven insights and predictive analytics. We can make faster and more accurate decisions using this modern approach.
When to Use Decision Intelligence Over Traditional Methods:
We need to consider when to use decision intelligence instead of traditional methods. Certain situations benefit more from technology-driven approaches.
High-stakes decisions often require precise data analysis. In business, for example, companies face choices that affect profits and growth. Using decision intelligence allows them to predict market trends accurately. They can respond quickly to changes.
Another scenario is when we deal with large volumes of data. Traditional methods struggle with big data sets. Decision intelligence excels here by processing vast amounts of information quickly. It finds connections that we might overlook manually.
However, some situations still call for traditional decision-making. Personal choices often rely on our values and emotions. When choosing a college or career path, we consider personal aspirations more than cold hard facts.
Blending Both Approaches for Best Results:
We believe that combining both decision intelligence and traditional methods yields the best outcomes. Each approach has its strengths and weaknesses.
By blending these strategies, we can enhance our decision-making process. For example, in a business setting, we can start with data analysis using decision intelligence tools. These tools identify trends and risks quickly.
After gathering insights from technology, we can apply our understanding of the company culture and market dynamics. This combination ensures that decisions are not just data-driven but also aligned with our values.
Blending approaches fosters collaboration among team members. We can discuss insights from decision intelligence while considering individual perspectives from traditional methods. This leads to richer discussions and better final decisions.
Customizing Decision Intelligence for Your Needs:
Tailoring Solutions to Fit Your Specific Goals:
We can customize decision intelligence to meet our specific goals. Each of us has unique challenges. Whether we are in business, healthcare, or education, our needs differ. We start by identifying our objectives clearly. This helps us choose the right tools and methods.
For example, businesses often seek to improve sales. We might use data analytics to understand customer behavior better. In contrast, schools may focus on student performance. Here, we could analyze test scores and attendance records. This way, we tailor our approach based on what we want to achieve.
We also need to consider the technology we have. Some tools work better for certain tasks than others. By assessing our resources, we can select the most effective solutions. This ensures that our decision intelligence system aligns with our goals.
Scaling Decision Intelligence as You Grow:
As we grow, so do our needs. Scaling decision intelligence is essential for long-term success. We must ensure that our systems can handle increased data and complexity over time.
Starting small is often a good strategy. We can implement basic decision intelligence tools first. These tools help us gather insights without overwhelming us. As we become comfortable, we can expand our capabilities.
For instance, a small business might begin with simple reporting tools. These tools provide basic information about sales and expenses. Once comfortable, we can integrate more advanced solutions like predictive analytics. This allows us to forecast trends and make proactive decisions.
Moreover, scaling requires ongoing training and support. We should invest in learning opportunities for ourselves and our teams. This helps everyone adapt to new technologies smoothly. When we all understand how to use these tools effectively, it leads to better outcomes.
Personalizing Decision Intelligence for Individual Use:
Personalization plays a crucial role in decision intelligence. Each of us has different preferences and ways of working. By personalizing our experience, we increase engagement and effectiveness.
We can start by customizing dashboards and reports. For example, some team members may prefer visual data representations like charts and graphs. Others might find detailed spreadsheets more helpful. By allowing individual customization, we cater to different learning styles.
Setting up alerts for specific metrics can enhance our decision-making process. If one of us focuses on customer satisfaction scores, receiving updates on these scores helps us react quickly. This personalized approach keeps us informed and ready to act.
Furthermore, feedback loops are essential for personalization. By sharing experiences and suggestions among ourselves, we can enhance the decision intelligence system continuously. Regular check-ins help identify what works well and what doesn’t.
The Human Side of Decision Intelligence:
Balancing Automation with Human Judgment:
We often find ourselves in discussions about how decision intelligence can enhance our lives. Automation plays a significant role in this process. It helps us analyze vast amounts of data quickly. However, we must remember that human judgment is irreplaceable. Machines can crunch numbers, but they lack the intuition and empathy that we bring to decision-making.
In many cases, relying solely on automation can lead to poor choices. For example, an automated system might suggest a solution based on past data. But it cannot account for new variables or changing circumstances. We have to consider the human element in every decision. Our experiences and emotions shape our choices in ways that technology cannot replicate.
Finding the right balance between automation and our insights is crucial. We can use technology to support our decisions rather than replace them. By doing this, we enhance our capabilities while still valuing human input. This approach leads to better outcomes for everyone involved.
Building a Decision-Minded Culture:
Creating a culture focused on decision-making is vital for us all. A decision-minded culture encourages critical thinking and open dialogue. When we foster this environment, it empowers everyone to contribute their perspectives. Each person’s input adds value to the decision-making process.
To build this culture, we need to promote continuous learning. We should encourage team members to share their experiences and lessons learned from past decisions. This sharing creates a collective knowledge base that benefits everyone. It also helps us avoid repeating mistakes.
Moreover, leaders play a critical role in shaping this culture. They should model decision-making behaviors and encourage others to do the same. By showing vulnerability and admitting mistakes, they create trust within the team. This trust allows us to explore ideas freely without fear of judgment.
Encouraging Collaboration Between Teams and Tech:
Collaboration is key when integrating technology into our decision-making processes. We must ensure that teams work together effectively with the tools at their disposal. Technology should act as a bridge, connecting different departments and facilitating communication.
We can achieve this by providing training on how to use decision intelligence tools effectively. Understanding how these tools work enables us to maximize their potential. When everyone is on the same page, we make more informed decisions together.
Creating cross-functional teams can enhance collaboration further. These teams combine diverse skill sets and perspectives, leading to richer discussions. By leveraging each member’s strengths, we can tackle complex problems more efficiently.
Case Studies and Examples:
Small Business Success with Decision Intelligence:
We often hear stories about small businesses that thrive using decision intelligence. One such example is a local coffee shop. This shop used data to understand customer preferences. They analyzed sales during different times of the day. With this information, they adjusted their staffing and inventory. As a result, they increased profits by 20% in just six months.
Another small business, a boutique clothing store, applied decision intelligence to improve marketing strategies. They collected data from social media and website visits. This data helped them identify popular trends among their customers. The store tailored its promotions to match these trends. Their targeted campaigns led to a 30% increase in foot traffic.
We also see restaurants benefiting from decision intelligence. A restaurant chain analyzed customer reviews and feedback. They discovered which dishes were most liked and which ones needed improvement. By focusing on customer favorites, they enhanced their menu. This change resulted in higher customer satisfaction and repeat visits.
Large Enterprises Transforming with Decision Intelligence:
Large enterprises are also embracing decision intelligence to drive transformation. For instance, a major retail company implemented advanced analytics tools. These tools helped them predict inventory needs more accurately. By reducing overstock and stockouts, they saved millions annually.
Another large enterprise, an automotive manufacturer, used decision intelligence to streamline production processes. They analyzed factory data to identify bottlenecks in the assembly line. By making adjustments based on this analysis, they improved efficiency by 25%. This change allowed them to meet growing demand without sacrificing quality.
We can look at financial institutions as well. A leading bank adopted decision intelligence for risk assessment. They used algorithms to evaluate loan applications more effectively. This method reduced default rates significantly while increasing loan approvals. Customers benefited from faster decisions, enhancing their overall experience.
Non-Profit Organizations Leveraging Decision Intelligence:
Non-profit organizations are not left behind in this trend either. We have seen many non-profits use decision intelligence to maximize their impact. One organization focused on environmental conservation leveraged data for project planning. They analyzed geographical data to determine the best areas for tree planting initiatives. This approach led to a successful campaign that planted over 100,000 trees in one year.
Another non-profit dedicated to education utilized decision intelligence for fundraising efforts. They analyzed past donation patterns and donor demographics. By understanding who their donors were, they created personalized outreach strategies. This resulted in a 40% increase in donations during their annual campaign.
Healthcare non-profits also benefit from decision intelligence by improving patient outreach programs. One organization used data analytics to identify communities with high health risks. They tailored their health education programs accordingly, leading to better health outcomes for those communities.
Troubleshooting Common Issues:
Identifying and Fixing Data Problems:
We often face challenges when working with data. Inaccurate or incomplete data can lead to poor decisions. We need to identify these issues early. For example, if we notice missing values in our dataset, it affects our analysis.
To fix these problems, we can start by cleaning the data. This involves removing duplicates and filling in gaps. Tools like Excel or specialized software can help us with this task. Regular audits of our data also keep it reliable. We should check for any inconsistencies frequently.
Another common issue is outdated information. We must ensure that our data is current. Outdated data can mislead us into making wrong choices. Establishing a regular update schedule can help maintain accuracy. By doing this, we support better decision-making processes.
Addressing Misaligned Goals and Objectives:
Misalignment of goals can derail our projects. When our objectives don’t match, confusion arises. We need to align our team’s goals with the overall vision. To do this, we should hold meetings to discuss our targets.
We can use frameworks like SMART goals to guide us. SMART stands for Specific, Measurable, Achievable, Relevant, and Time-bound. This method helps clarify our objectives. It ensures everyone understands what we aim to achieve.
Moreover, regular feedback sessions are crucial. These meetings allow us to assess progress and make adjustments. They help keep everyone on the same page and motivated. When we share insights and concerns openly, we foster collaboration.
Resolving Technical Glitches and Integration Hurdles:
Technical glitches can be frustrating during our decision-making process. Software bugs or system failures disrupt our workflow. We need a plan to troubleshoot these issues effectively.
First, we should document any problems clearly. This documentation helps us understand the situation better. Next, reaching out to technical support can provide quick solutions. Many software companies offer resources for common issues.
Integration hurdles also pose challenges when combining different systems. We have to ensure that all tools communicate effectively. Using APIs or middleware solutions can streamline this process. Testing integrations before full deployment prevents future complications.
Integrating Decision Intelligence with Other Technologies:
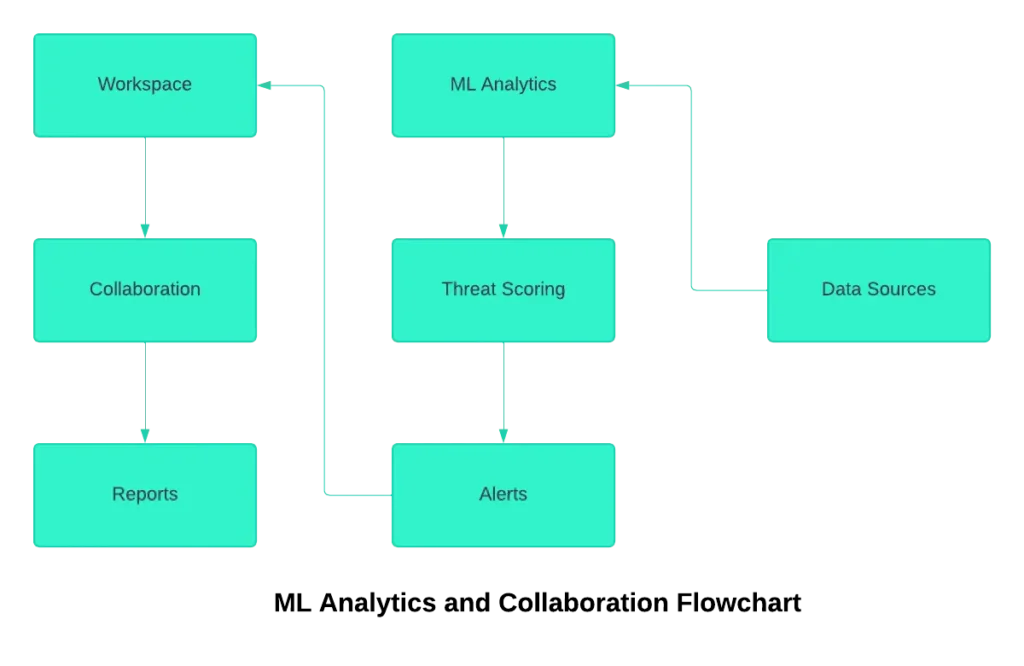
Combining Decision Intelligence with Big Data:
We often hear about big data in discussions about technology. It refers to the vast amounts of information generated every second. With decision intelligence, we can analyze this data effectively. We can spot patterns and trends that help us make better choices.
In 2020, a study showed that companies using big data improved their decision-making speed by 5 times. This means they could react quickly to changes in the market. Imagine being able to adjust our strategies almost instantly. That’s the power of combining decision intelligence with big data.
Using advanced analytics tools helps us process large datasets. We can uncover insights that drive our decisions forward. For instance, businesses can track customer behavior in real-time. This information allows them to tailor their products and services to meet customer needs.
Synergies Between Decision Intelligence and IoT:
The Internet of Things (IoT) connects devices and gathers data from various sources. We find that integrating decision intelligence with IoT creates powerful synergies. Smart devices collect data continuously. This data can inform our decisions immediately.
For example, smart home systems monitor energy usage. They provide feedback on how to save energy costs. By applying decision intelligence, we can optimize our energy consumption based on this information. Companies also use IoT sensors in factories to monitor equipment health. This helps prevent breakdowns and improve efficiency.
In 2019, research indicated that IoT adoption increased productivity by 30%. This proves how effective the combination of decision intelligence and IoT can be for businesses. We must embrace these technologies to stay competitive in today’s market.
Enhancing Decision Intelligence with Cloud Computing:
Cloud computing offers flexibility and scalability for businesses. By enhancing decision intelligence with cloud solutions, we gain access to vast resources without heavy investments in hardware. Cloud platforms allow us to store and analyze large volumes of data seamlessly.
In 2021, a report showed that 94% of businesses saw improvements after adopting cloud computing. This is due to the ability to share insights across teams quickly. We can collaborate effectively, making informed decisions based on real-time data.
Furthermore, cloud-based tools enable us to implement machine learning models easily. These models enhance our decision-making processes by predicting outcomes based on historical data. For instance, retailers can forecast sales trends more accurately, allowing them to manage inventory better.
Ethical Considerations in Decision Intelligence:
Ensuring Fairness and Avoiding Bias:
We often hear about the importance of fairness in decision-making. In our adventures, we notice how bias can creep into choices. When we use decision intelligence to eradicate bias in AI, we must ensure that algorithms treat everyone equally.
Data used in these systems can reflect past biases. For instance, if an algorithm learns from biased data, it may continue those unfair patterns. This can lead to unjust outcomes in areas like hiring or lending. We should actively work to identify and eliminate these biases. Regular audits of algorithms help us spot issues before they cause harm.
In 2020, a study found that facial recognition systems misidentified people of color more often than white individuals. This highlights the need for fairness in our technologies. We must strive for diverse data sets. By including different perspectives, we can create more balanced decision-making processes.
Transparency in Automated Decisions using Decision Intelligence:
Transparency is crucial when using automated systems. We want to understand how decisions are made. If we can’t see inside the “black box” of algorithms, we might not trust their outcomes.
For example, when we apply for loans, we expect to know why we were approved or denied. Clear explanations build trust with users. We should advocate for systems that provide insights into their decision-making process.
In 2018, the European Union introduced regulations requiring companies to explain automated decisions. This step promotes accountability and helps users understand the criteria behind choices. By demanding transparency, we empower ourselves and others to make informed decisions.
Accountability in Decision Intelligence: Who’s Responsible for Decisions?
Accountability plays a vital role in decision intelligence. When an algorithm makes a mistake, who is responsible? Is it the developers, the company, or the system itself? We must clarify these roles to ensure fair treatment.
In our experiences, we have seen how important it is to own up to errors. For example, if a self-driving car gets into an accident, questions arise about liability. Is it the manufacturer’s fault or the software’s? These questions need clear answers.
We should push for laws that define accountability in technology use. In 2021, California passed a law requiring companies to be accountable for AI-driven decisions. This sets a precedent for other states and countries to follow.
Building a Roadmap for Decision Intelligence:
Setting Clear Objectives and Goals for Decision Intelligence:
We must begin our journey by defining what we want to achieve with decision intelligence. Setting clear objectives is crucial. It helps us focus our efforts and resources. For instance, we might aim to improve customer satisfaction or increase operational efficiency. These goals guide our actions and decisions.
Next, we should ensure that our objectives are specific, measurable, achievable, relevant, and time-bound (SMART). By following this framework, we can track our progress effectively. For example, if we decide to enhance customer service response times, we can set a goal of reducing them by 20% within six months. This clarity motivates us to stay on course.
Involving team members in this process is vital. Their insights can lead to more comprehensive objectives. We should hold brainstorming sessions to gather ideas and perspectives. This collaboration fosters ownership among all team members. When everyone feels included, they are more likely to commit to the goals we’ve set.
Planning Your Implementation Strategy:
After setting our objectives, we need a solid implementation strategy. This strategy outlines how we will achieve our goals. First, we should identify the tools and technologies required for decision intelligence. For instance, data analytics software can help us analyze trends and patterns in real-time.
Next, we need to establish a timeline for implementation. Breaking down the process into smaller steps makes it manageable. We can create a timeline that includes key milestones along the way. This way, we can celebrate small victories as we progress toward our larger goals.
Furthermore, training is essential for our team members. They must understand how to use new tools effectively. We should schedule workshops or training sessions to ensure everyone is equipped with the necessary skills. This investment in our people pays off in the long run.
Monitoring Progress and Making Adjustments using Decision Intelligence:
As we implement our strategy, monitoring progress becomes critical. Regularly reviewing our performance helps us stay aligned with our objectives. We should track key performance indicators (KPIs) related to our goals. For example, if we aimed to improve response times, we could measure how quickly customer inquiries are handled.
If we notice any discrepancies between our targets and actual results, adjustments may be needed. Flexibility allows us to adapt to changing circumstances or unexpected challenges. For instance, if a particular strategy isn’t yielding the desired results, we can pivot and explore alternative approaches.
Moreover, feedback from team members is invaluable during this phase. Their experiences provide insights into what’s working well and what isn’t. We should encourage open communication within our group. This dialogue creates an environment where everyone feels comfortable sharing their thoughts.
Getting Expert Help:
When to Consult a Decision Intelligence Specialist:
We often face complex decisions in our lives. These choices can impact our future significantly. Knowing when to seek help is crucial. We should consider consulting a decision intelligence specialist when we encounter problems that require deep analysis. If our data is vast and difficult to interpret, experts can guide us. They possess the skills to transform raw data into actionable insights.
Timing matters too. Seeking help early in the decision-making process can save us time and resources. If we notice patterns in our data but can’t decipher them, it’s time to reach out. Specialists can help us understand these trends better. They can also assist us in establishing clear goals for our projects.
Moreover, if we feel overwhelmed by the options available, we should consult an expert. They can simplify our choices and provide clarity. Their experience allows them to identify potential pitfalls. This guidance helps us avoid costly mistakes. We must remember that asking for help shows strength, not weakness.
Finding the Right Partners and Vendors for Decision Intelligence:
We know that not all decision intelligence specialists are the same. Finding the right partners is essential for successful collaboration. First, we need to define what we want from this partnership. Identifying our goals will help narrow down our options.
Research plays a vital role in this process. We should look for vendors with proven track records, such as Kodexo Labs. Checking reviews and testimonials can provide insight into their capabilities. Networking within our industry can also lead us to reputable specialists. Recommendations from trusted sources are invaluable.
Once we have a list of potential partners, we should evaluate their expertise closely. Are they familiar with our specific challenges? Do they have experience with similar projects? Asking these questions helps ensure that we choose a vendor who understands our needs.
Cost is another factor to consider. While we may want top-tier services, budget constraints often play a role in our decisions. We must balance quality with affordability. Requesting quotes from multiple vendors allows us to compare prices and services.
Collaborating with Experts for Best Results using Decision Intelligence:
Collaboration is key in decision intelligence. Working closely with experts leads to better outcomes for our projects. We should communicate clearly about our objectives and expectations from the start. Establishing open lines of communication fosters trust between us and the specialists.
Regular check-ins are important as well. These meetings allow us to discuss progress and address any concerns promptly. By staying engaged throughout the process, we ensure that everyone is on the same page.
Sharing data and insights openly enhances collaboration. The more information we provide, the better equipped our partners will be to assist us effectively. This exchange of knowledge can spark innovative ideas that may not have emerged otherwise.
Finally, we must be willing to adapt based on expert feedback. Decision intelligence specialists bring valuable perspectives to the table. Being open-minded about their suggestions can lead us to more informed decisions.
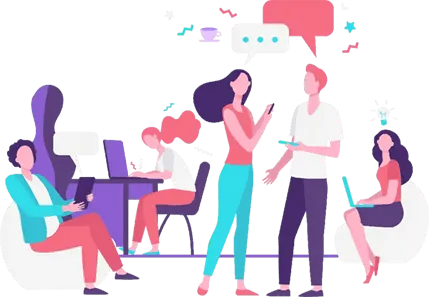
Closing Thoughts:
Decision intelligence is transforming how we make choices. We’ve explored its core components, benefits, and real-world applications. This powerful approach blends data and human insight, leading to smarter decisions. As we integrate these strategies into our organizations, we’ll see efficiency soar and outcomes improve.
Let’s embrace decision intelligence together. The future is bright with possibilities. We can enhance our skills and face challenges head-on. Let’s stay informed and keep pushing the boundaries of what we can achieve. Join us in this journey and unlock the full potential of decision intelligence today!
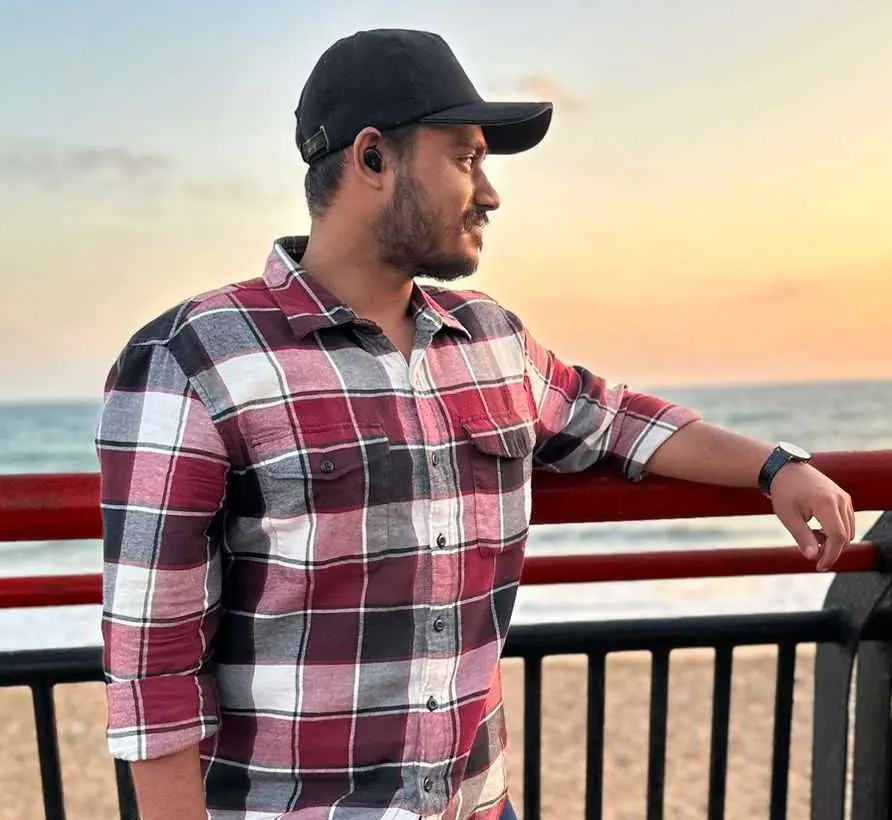